Why AI needs more human intelligence if it's to succeed
That robot may be able to mimic what you say, but it can't attach meaning to the words
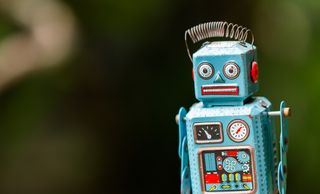
I got suckered into watching "The Robot Will See You Now" during Channel 4's November week of robot programmes. Shoddy though it was, it conveyed more of the truth than the more technically correct offerings. Its premise: a family of hand-picked reality TV stereotypes being given access to robot Jess, complete with a "cute" flat glass face that looked like a thermostat display, and they consulted he/she/it about their relationship problems and other worries.
Channel 4 admitted Jess operates "with some human assistance", which could well have meant somebody sitting in the next room speaking into a microphone, but Jess was immediately recognisable to me as ELIZA in a smart new plastic shell.
ELIZA, for those too young to know, was one of the first AI natural language programs, written in 1964 by Joseph Weizenbaum at MIT and 16 years later by me while learning Lisp. It imitated a psychiatrist by taking a user's questions and turning them around into what looked like intelligent further questions, while having no actual understanding of meaning. Jess did something similar in a more up-to-date vernacular.
Both Jess and ELIZA work because they give their patients somebody neutral to unload upon, someone non-threatening and non-judgemental. Someone unlike their friends and family. Jess's clearly waterproof plastic carapace encouraged them to spill the beans. Neither robot doctor needs understand the problem being shared, merely to act as a mirror in which patients talks themselves into solving it.
Interacting with Jess was more about emotion than about AI, which points up the blind alley that AI is currently backing itself into. I've written here several times before about the way we confuse *emotions* with *feelings*: the former are chemical and neural warning signals generated deep within our brains' limbic system, while feelings are our conscious experience of the effects of these signals on our bodies, as when fear speeds our pulse, clarifies vision, makes us sweat.
These emotional brain subsystems are evolutionarily primitive and exist at the same level as perception, well before the language and reasoning parts of our brains. Whenever we remember a scene or event, the memory gets tagged with the emotional state it produced, and these tags are the stores of value, of "good" versus "bad". When memories are later retrieved, our frontal cortex processes them to steer decisions that we believe we make by reason alone.
All our reasoning is done through language, by the outermost, most recent layers of the brain that support the "voice in your head". But language itself consists of a huge set of memories laid down in your earliest years, and so it's inescapably value-laden.
Get the ITPro. daily newsletter
Receive our latest news, industry updates, featured resources and more. Sign up today to receive our FREE report on AI cyber crime & security - newly updated for 2024.
Even purely abstract thought, say mathematics, can't escape some emotional influence (indeed it may inject creativity). Meaning comes mostly from this emotional content, which is why language semantics is such a knotty problem that lacks satisfactory solutions - what a sentence means depends on who's hearing it.
The problem for AI is that it's climbing the causality ladder in exactly the opposite direction, starting with algorithmic or inductive language processing, then trying to attach meaning afterwards. There's an informative and highly readable article by James Somers in the September MIT Technology Review, about the current explosion in AI capabilities - driverless cars, Siri, Google Translate, AlphaGo and more.
He explains that they all involve "deep learning" from billions of real-world examples, using computer-simulated neural nets based around the 1986 invention of "backpropagation" by Geoffrey Hinton, David Rumelhart and Ronald Williams.
Somers visits Hinton in the new Vector Institute in Toronto, where they're finally able to decipher the intermediate layers of multilayer backpropagation networks, and are amazed to see structures spontaneously emerge that somewhat resemble those in the human visual and auditory cortexes.
Somers eschews the usual techno-utopian boosterism, cautioning us that "the latest sweep of progress in AI has been less science than engineering, even tinkering". He adds: "Deep learning in some ways mimics what goes on in the human brain, but only in a shallow way."
For example, all these whizzy AI products exhibit a fragility quite unlike the flexibility of HI (human intelligence). I believe this fundamental fragility stems from AI's back-to-front approach to the world, that is, from its lack of HI's underlying emotional layer that lies below the level of language and is concurrent with perception itself. We judge and remember what we perceive as being good or bad, relative to deep interests like eating, escaping, playing and procreating. Jess could never *really* empathise with his fleshy interrogators because, unlike them, he/she/it never gets hungry or horny.
Image: Shutterstock