How Intel's FaceCatcher hopes to eradicate real-time deepfakes
The company’s ‘blood flow’ breakthrough could banish deepfakes to history
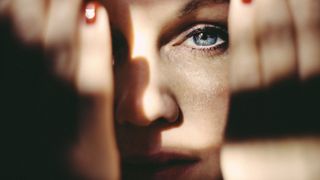
Artificially generated fake videos featuring familiar faces – deepfakes – have the potential to scramble our perception of what is real. As the technology has improved and refined, though, the deepfake detectors have been fighting back.
Now, Intel has invented what may be a significant step forward in helping us separate living, breathing humans from artificial intelligence (AI) puppets.
Intel calls its creation FakeCatcher, and it works by taking a very close look at the blood flow in our faces, using a technique called photoplethysmography (PPG).
“I said, there should be some priors that we can trust in real videos. What are those priors?” says Dr Ilke Demir, senior staff research scientist at Intel Labs, who invented the system. “And then I saw an MIT paper about finding blood flow from videos.”
“We first find the face and, from the face, we find the facial landmarks,” says Demir. “From the facial landmarks, we extract the region of interest.”
The system then uses Intel’s OpenVino deep learning toolkit to correct for geometry – overlaying a grid on the face to carefully analyse the minute changes in colours of blood vessels under the skin, every 64 or 128 frames. “From each grid cell, we extract the PPG signal,” Demir adds, explaining how PPG is a particularly effective tell a human is real because deepfake software cannot yet correct for PPG.
“It is such a subtle signal that is correlated everywhere on our face. So, it is almost impossible to replicate,” she says.
Get the ITPro. daily newsletter
Receive our latest news, industry updates, featured resources and more. Sign up today to receive our FREE report on AI cyber crime & security - newly updated for 2024.
Achieving transformative business results with machine learning
How seven leading organisations are using machine learning to resolve key challenges and reveal new opportunities
According to Intel, the FakeCatcher system is so robust it can detect deepfakes in 96% of cases, and in real-time. Consequently, it’s conceivable that future videoconferencing software can pop up a warning if it believes you’re speaking to a fraudster.
It even works if the faker tries to be clever and get around it by turning on a face-smoothing filter, for example. “The smoothing operator is actually a linear operator,” says Demir. “So even if you smooth your face, the signals are still correlated for the real video.” Even though a smoothed face might have a different PPG score for each cheek, the two figures will still be correlated on a real video – so even if our cheeks are read as different colours, the difference between the two should remain fixed.
The system works on heavily compressed videos too – at least, to a point. “We saw that if we train the model only on non-compressed videos, and then test on compressed videos, the accuracy drops,” says Demir. “But if we add compressed videos into our training set, and then train it on a mix of non-compressed [and] compressed videos, then the accuracy again is trustable on par with our original results.”
In fact, the only real bête noire of FakeCatcher, according to Intel at least, are situations where the light hitting a subject’s face is constantly changing, as it makes it hard to measure the colours. If that ever happened, though, you would probably suspect something strange was happening when you saw the fraudster on Zoom running a strobe light in the background during your call.
It seems beating FakeCatcher is going to be tricky for the deepfakers. “Because of the nature of PPG extraction, you cannot backpropagate,” says Demir.
Teaching a machine learning algorithm how to account for PPG would also be hard because the training data isn’t widely available. “If you want to approximate it somehow, you need a very large PPG data sets and that doesn't exist yet,” says Demir. “There are like 30 people or 40 people datasets, which are not generalisable to the whole population, so you cannot use it in a deep learning setting to approximate PPG signals.”
Even if such a large dataset were to exist because, say, a hospital released a raft of data from patients, Demir argues Intel can upgrade its model to work probabilistically based on correlations in the PPG data – which would mean the deepfake would need to be even more flawless to pass detection. It really does seem conceivable that PPG detection might be the technology that stops deepfakes in their tracks.