Five steps for implementing predictive AI successfully
Predictive AI has a role to play in the data-driven business, but it will only perform well if it is properly implemented
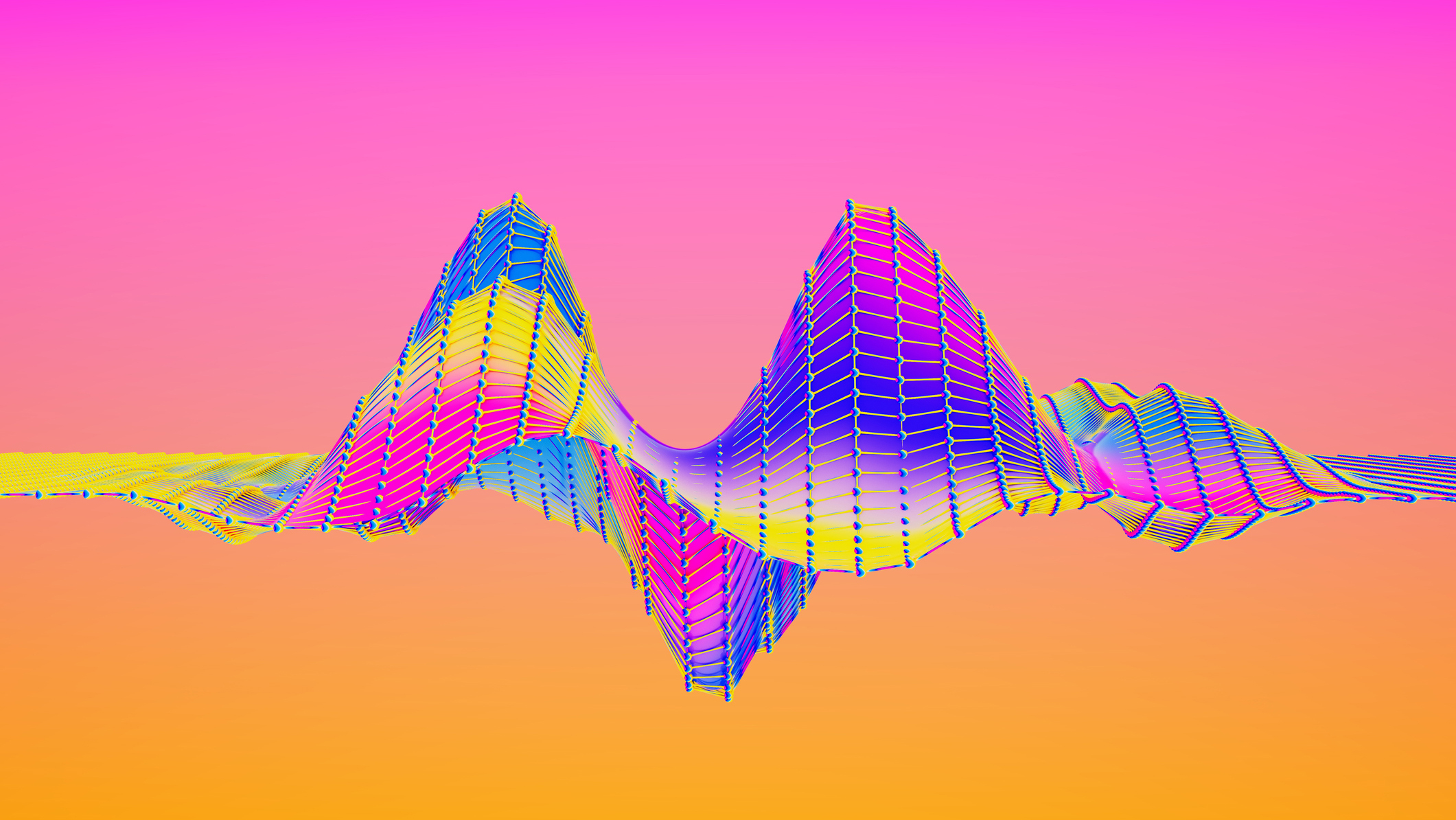
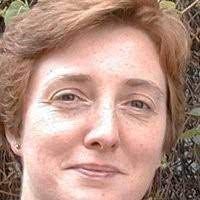
Predictive analytics is not a new concept. Before the arrival of the computer, businesses would examine purchasing trends, profit margins, external factors, and other metrics to make decisions on how best to maximize both profit and responsiveness to customers.
Computers, and spreadsheets in particular, allowed businesses to refine their approach to analytics, learn more, and adjust practices accordingly. In a sense, the advent of computers allowed businesses to become fully data-driven for the first time.
AI adds more computing power as well as the ability to analyze much larger data sets. It can run scenarios and compare outcomes to aid decision making; it’s predictive. At its most sophisticated, predictive AI can help a business do things like work around supply chain issues, predict market trends, fine-tune stock ordering, and help optimize production lines.
However, as with most systems, it’s a case of ‘garbage in, garbage out’. Predictive AI is only as good as the information it has to work with. So how does a business ensure that it is implementing predictive AI well, and getting the most out of the technology? We offer five steps to successfully implement predictive AI.
Step 1: Ask the right questions
It isn’t always ideal to ask predictive AI to draw conclusions for the future based on experience from the past. Clare Walsh, director of Education at the Institute of Analytics offers this example:
Imagine using predictive analytics to refine recruitment. You may want to look at the characteristics of people you have previously promoted, compare those to the characteristics in new CVs, and then use that information to secure new hires. After all, if you already have successful people in your business, it makes sense that you would want to replicate that, surely?
“If there is anything undesirable in that historic data set, such as a tendency to avoid female or ethnic minority applicants, then the machine will notice that and include it as a reason to reject people,” explains Walsh. “And these machines get incredibly clever and focused - even if you try to mask the gender, they will look for other clues in the style of language. You’d better have a sceptical human overseeing that process.”
Get the ITPro daily newsletter
Sign up today and you will receive a free copy of our Future Focus 2025 report - the leading guidance on AI, cybersecurity and other IT challenges as per 700+ senior executives
So how do you ask the right questions? One approach is to question your existing answers. Look around in the wider world to find data that might improve your questions. On the CV point, rather than asking questions about people you’ve hired, maybe try to find out why you aren’t hiring people from a broader spread of backgrounds. If your existing systems include racial hiring biases or fail neurodiverse candidates, you’d better find out how to fix this. Predictive AI can help you change your approach and enrich your workforce, but only if you instruct it to.
Step 2: Identify the right third party data sources
The value of predictive AI is dependent on the data. If you want robust insights, it’s up to you to provide rich datasets. Companies will have their own internal data, but it’s critical to incorporate other sources, including open source and third-party data. These help provide greater depth and context.
Naturally, these external data sets need to be fit for purpose. Historic data, in particular, can be riddled with biases. Dr Walsh suggests a starter list of questions that can be utilized whenever you are interrogating third party data:
- How was it trained?
- How was it tested?
- Can I inspect the training data and the performance figures in detail?
- Can I run a test on my own data to see how well the trained algorithm generalizes to my data set?
- What country was the data pooled from?
- How old was the data?
- When was it last refreshed?
Step 3: Scrutinize, sanitize, standardize
Wherever data originates, it has to be usable by your AI implementation, and some prep work may be required before it is usable.
“Text analytics that enrich and annotate raw data allow you to extract the keywords, concepts and terms you want the predictive model to recognise,” Mirit Eldor, managing director of Elsevier Life Sciences Solutions. “This process also harmonises synonymous terms, for example ‘heart attack’ and ‘myocardial infarction’ in electronic patient records, so they are identified as the same entity when predictions are calculated.”
While coming from a medical perspective, the sentiment holds for other sectors too. “Ask whether the data accurately represents the problem space and if it encompasses a broad enough spectrum to make meaningful predictions,” suggests Mike Mason, chief AI officer at tech consultancy Thoughtworks. “Then, proceed with data cleaning to address errors and inconsistencies, and deduplication to remove any repetitive information.
“Additionally, consider anonymization to protect privacy and, where beneficial, enrich the data with external sources to enhance model accuracy.”
Step 4: Test and challenge
With the data prepared and questions decided, you might think you’re ready to get meaningful, useful, accurate answers. Maybe you are. But it’s important to test and challenge.
RELATED WHITEPAPER
“Firms shouldn’t simply accept their AI’s predictions at face value,” explains Mason. “Just like with any complex system, rigorous testing throughout its lifecycle is crucial. This uncovers potential issues, improves performance, and builds trust through transparency.”
Mason also advocates for the testing of testing. “As AI becomes increasingly pervasive in the enterprise tech stack, so too do the risks of inadequate testing,” he explains. “So you need to be attuned to falls in performance, unexpected outputs that you intuitively feel are flawed, decisions made on the basis of outputs that you feel could have been better decisions.”
Step 5: Realize the job is never done
As your needs change over time, and as you accumulate more data, you’ll want to examine it differently, add to it, change it. The first four steps are cyclical; you’re always going to want to use your predictive AI to look into the future, and so new capabilities and refined data will always be required.
To this end, you’re going to need to add new data sets, grow your expertise, and continue to test and challenge. Once predictive AI is part of your setup, the process will become an integral part of your business objectives.
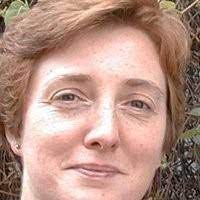
Sandra Vogel is a freelance journalist with decades of experience in long-form and explainer content, research papers, case studies, white papers, blogs, books, and hardware reviews. She has contributed to ZDNet, national newspapers and many of the best known technology web sites.
At ITPro, Sandra has contributed articles on artificial intelligence (AI), measures that can be taken to cope with inflation, the telecoms industry, risk management, and C-suite strategies. In the past, Sandra also contributed handset reviews for ITPro and has written for the brand for more than 13 years in total.