Can you trust AI?
Could biased artificial intelligence systems harm your business – and society itself?
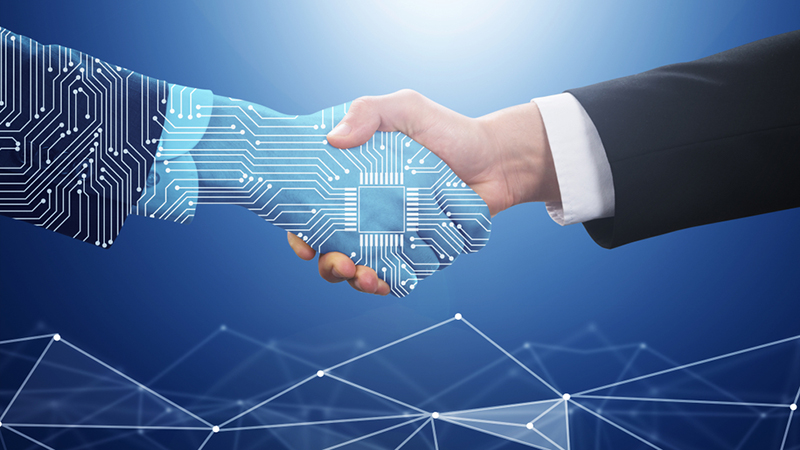
When we talk about untrustworthy artificial intelligence, we don't mean giant robot fascists not in the next few years, anyway. Rather, recent news reports have created the widespread suspicion that AI may come with preloaded, deeply rooted prejudices as part of its character. An example might be the revelation that, last year, Amazon had to scrap an AI-based recruiting tool after discovering that it was applying a sexist bias. Follow the idea to its extreme and the end of personal freedom, the destruction of living standards and worse could be at hand, due to inscrutable imbalances in the digital quanta of fairness.
But is that actually what's going on? Frankly, those of us who have a bit of historical perspective, and some conception of what "AI" really means, are mostly incredulous at the alarmism attached to ideas that are barely out of the research laboratory.
Let's look at another well-known example. Stephen Brobst, CTO of storage and analytics specialist Teradata, is among those who have pointed out how Amazon's US trial of same-day delivery back in 2015 is a classic study in perceived AI bias. It's easy to see why: when the new service was first trialled in the extended suburbs of Atlanta, an algorithm was used to determine which areas would be covered in the trial. The resulting map corresponded almost uncannily with mainly white areas, while majority black zip codes didn't get a look in.
Needless to say, Amazon's selection criteria did not anywhere exclude black people; the computer based its decisions solely on buying habits. However, as Brobst noted, the effect was functionally indistinguishable from racial bias. And it raises a question: if an AI trainer selects for buying habits that correlate with race and social status, are they being racist?
Readers who have some familiarity with AI may already be sputtering loudly at this stage. So let's rewind and take stock of the state of things in the present century. The first point to understand is that "artificial intelligence" is a bad label, not least because it's imprecise. There are at least two distinct architectures within the general idea of AI, namely expert systems and machine learning. Mainstream reporting freely mixes the two up, and overlooks the fact that neither qualifies as "intelligence" in the mainstream sense.
On the contrary, quite a lot of AI is wholly deterministic. Projects labelled as "AI" frequently do nothing more than very rapidly regurgitate preordained precepts, applying no judgment and adding no insight whatsoever. Yet it may be impossible for an outsider to recognise that that is what is happening. Indeed, many people like the idea of AI partly because it allows them to feel that responsibility for difficult or controversial decisions can be handed over to the machine.In truth, when "AI" gets the blame, the real issue is invariably to do with the specifics of the algorithm, or the input. It may feel good to paint objectionable outcomes as reflective of the moral failings of the board members of the company, but it's hardly fair.
Unfortunately, in a culture where almost nobody feels the need to distinguish between different types of AI, or to justify how a given process counts as AI at all, it's very hard to persuade a complainant that your artificial intelligence isn't responsible for what appear to be in-built prejudices. As I've noted, the natural human temptation is to turn things the other way, and blame the machine rather than the personnel.
Get the ITPro daily newsletter
Sign up today and you will receive a free copy of our Future Focus 2025 report - the leading guidance on AI, cybersecurity and other IT challenges as per 700+ senior executives
Who's really to blame?
If you go looking for informed responses to bias, you find some interesting things. For example, IBM has produced numerous videos and publications about the potential dangers of bias in AI. There's a focus on ideas such as fairness and trust, and ensuring that artificial intelligence acts "in concert with our values", but ultimately the tech giant acknowledges that as things currently stand human biases are very likely to find their way into AI algorithms. Consequently, as veteran programme manager Rattan Whig discusses in a wide-ranging blog post, the blank slate of a machine-learning AI can't help but reflect the cognitive patterns of its trainers.
And let's be clear: 99% of the time, we're not even dealing with a blank slate. Very few businesses have any use for the kind of AI that is trained in a black box, starting from a state of virginal grace. Potentially, those sorts of systems might be trained to spot a flaw in a manufacturing process by staring at millions of frames of video every day, exercising intelligence in much the same way that a nematode worm can make its way through life using a network of under 100 neurons as a "brain".
What those systems don't do, any more than a nematode worm can, is spot poor credit behaviour in a portfolio of customers, or identify underperforming stocks. That's the province of the other kind of AI, the kind that's put together painstakingly and over long development cycles by old-fashioned humans codifying their own knowledge. So it's hardly surprising when misapprehensions and biases get baked into the process.
A bridge too far
I've done my best to squeeze the mistakes commonly made by AI pundits into the shortest, least respectful summary I can manage. But I'm not finished, because I also want to talk about overreach.
What I mean by that is just how big the gap is between how AI technologies are represented and what actually exists. If we want to meaningfully blame an AI for anything, we have to argue that it has some grasp of the underlying meaning of the data it's sifting through. Think of an advertising system that monitors what you search for and serves up adverts that match those terms; that might appear intelligent, but really it's just dumb token matching. The computer has no idea what words such as "rugby kit" or "ticket to Swansea" actually mean in themselves.
One day we will get there, but it's a very long road. And it's not a road paved with gentle, incremental software releases: it's going to require more than one fundamental, world-shaking breakthrough.
Bear in mind that there's a lot more to "understanding" than simply being able to look up a word in a database and enumerate its connotations and connections. If we really want an artificial intelligence to be free of bias, it needs to be able to recognise and neutralise bias in its input. Detecting something that deep and subtle is going to require a machine that works with the same symbols as the human brain, and a good deal faster. There's nothing like that out there right now: to be honest, I doubt we'll see such a thing before we have defeated global warming.
So what should you do?
All this may seem very abstract and academic, but for you as a techie business "influencer" it's actually good news. Because the upshot is that the bias problem is far simpler and more comprehensible than alarmist news reports would have you believe. You don't need to worry about what's going on inside the inscrutable digital mind of a malevolent AI.
The issue, in reality, comes down to the programmer, and the sources of things such as keyword libraries things you can have an opinion about, and influence directly if you perceive that they are steering you in the wrong direction. It's not as if it would ever be in your interest to indulge a biased AI anyway: businesses are engines for making money, and biases by their nature needlessly exclude market sectors.
Unfortunately, the woolly idea of an evil AI makes good headlines, and the speed of the internet means that when an incidence of bias is unearthed, protests and outrage can easily spread far more quickly than a fix can be implemented, tested and deployed. None of this helps anyone to make informed and helpful choices about what type of AI or learning database they should be using, or what steps they should be taking to improve its accuracy and usefulness.
Perhaps the way forward is for the protesters to turn auditors: if we could get the media to stop lazily obfuscating and muddling the very idea of AI, those who have expressed outrage at the ills of seemingly biased AI might start to realise how such perverse outcomes come to pass. They could then become part of the solution, helping to both discover and document instances of poor programming or ill-chosen data and all of us, both businesses and society at large, would benefit.
-
RSAC Conference 2025: The front line of cyber innovation
ITPro Podcast Ransomware, quantum computing, and an unsurprising focus on AI were highlights of this year's event
-
Anthropic CEO Dario Amodei thinks we're burying our heads in the sand on AI job losses
News With AI set to hit entry-level jobs especially, some industry execs say clear warning signs are being ignored