Dystopian tech has failed to live up to the hype
Cambridge Analytica overselling its micro-targeting capabilities is just the latest example of intrusive technologies falling short of expectations
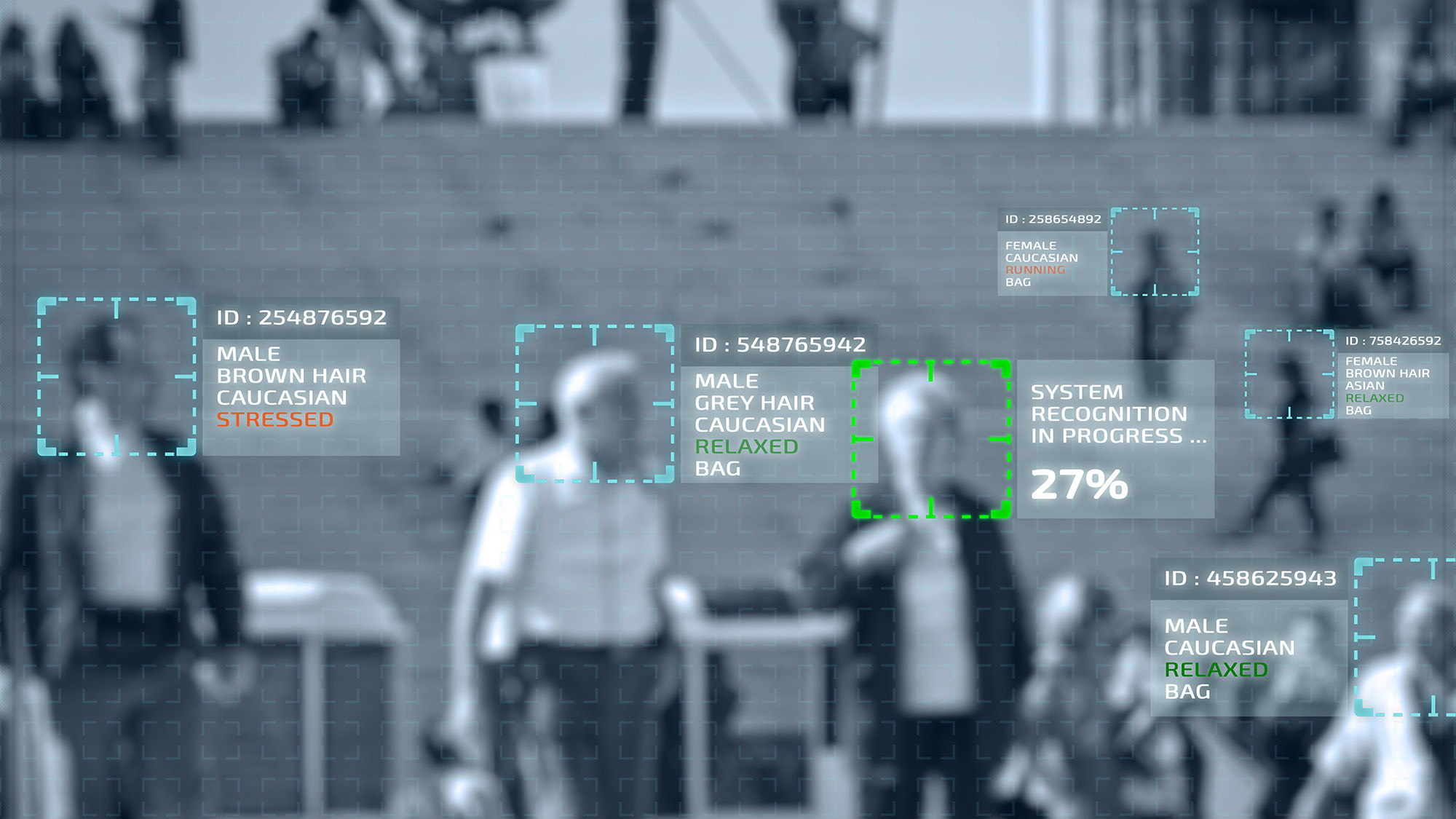
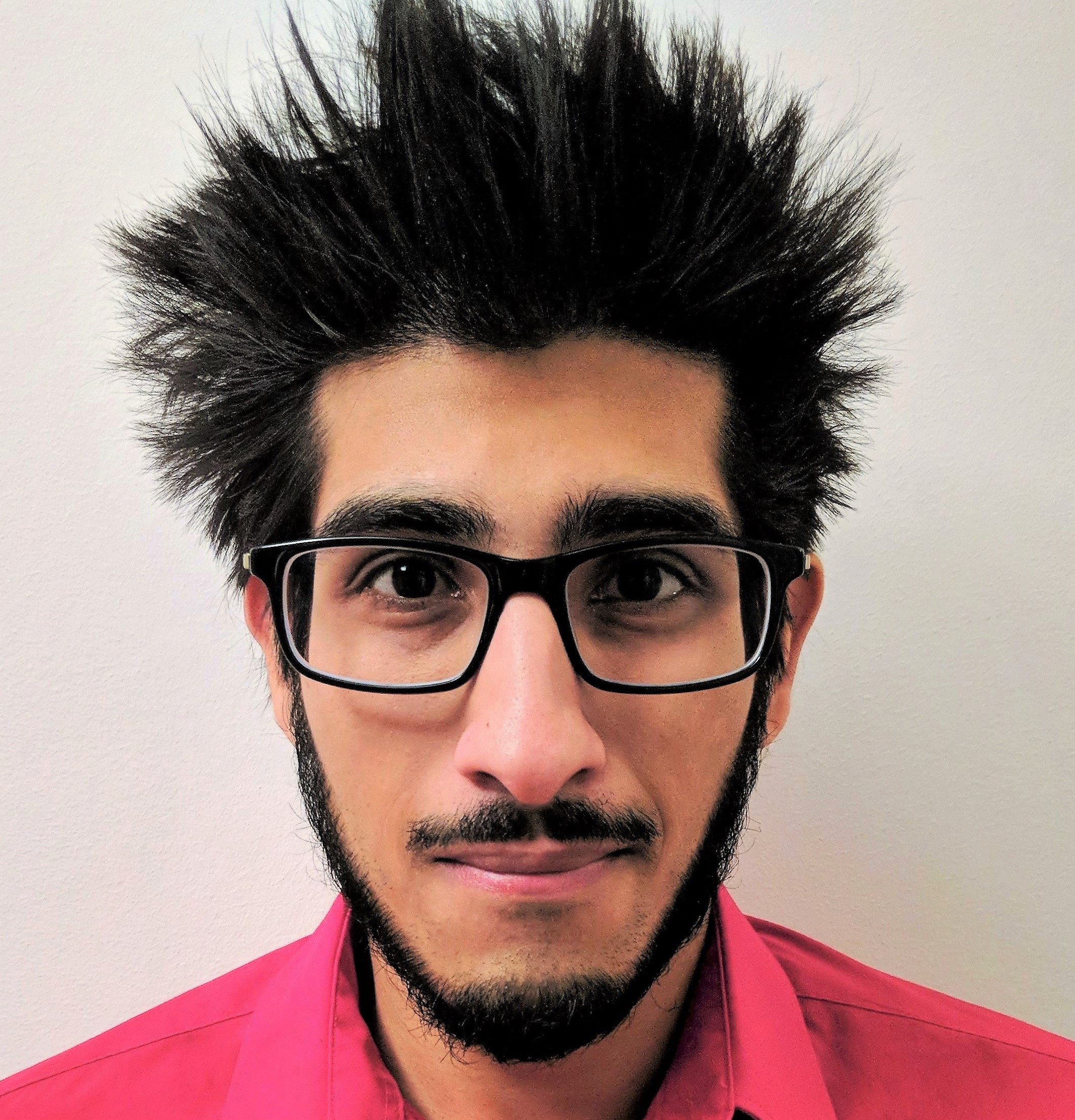
The Cambridge Analytica scandal was arguably the most significant watershed moment for the tech industry in recent times. Five years on from the initial disclosures, we can see the ways in which data protection and privacy have become major issues. It’s true not just for businesses, but for users too, who’ve often been apathetic to their data footprint.
The now-defunct company’s micro-targeting technology, powered by algorithms, sounded highly alarming, although analysis by the Information Commissioner’s Office (ICO) suggests its efficacy was greatly exaggerated. It could be argued that this immense focus on privacy and data protection, of which GDPR was a key part, was formed on the basis of an illusion. Although the nature of such data-scraping and analytical capabilities were widely-considered ‘dystopian’, especially when applied in a political context, and such practices have (publicly, at least) been shunned, Cambridge Analytica’s tools, in reality, hardly made a difference when deployed in the real world.
Rage against the machine
Rather than being an exception, this scandal might be part of a wider pattern in which controversial ‘dystopian’ technologies prove to be rather underwhelming. After all, intense opposition has since grown against similarly worrisome systems.
The use of facial recognition in law enforcement, for example, attracted major backlash following the Black Lives Matter movement, with tech firms quick to suspend their projects. In the UK, meanwhile, such technology has been trialled by a handful of forces for many years. While its use hasn’t attracted the same level of notoriety, there’s still considerable opposition from civil rights groups, including Liberty, with the ICO even stepping in last year to curb its use in law enforcement due to data protection concerns.
It’s not just questions over data protection and human rights that afflict facial recognition technology, either. Findings published last year by the University of Essex show that these systems have mistakenly flagged a sizable portion of innocent people as wanted suspects, according to a Sky News report. From a sample of 1,000 cases based on Met Police usage, the research found that 81% of those identified as ‘suspects’ were actually innocent. This is in addition to research from Big Brother Watch in 2018, which found the technology was misidentifying innocent people at a frightening rate. The technology simply isn’t good enough to be used for its actual purpose.
Liberty and Big Brother Watch, alongside campaign group Foxglove, have also railed against the use of AI-powered algorithms by government agencies, most recently the system used to determine this year’s A-Level results after COVID-19 disrupted exams. Although the notion of a machine determining the futures of hundreds of thousands of students may seem dystopian, the project was ultimately a failure and the system was ditched in favour of teacher-informed centre assessed grades.
Smashing the black box
All of these incidents and more have led to a mistrust of AI in general and the use of ‘black box’ algorithms in particular. For professor Andy Pardoe, founder and MD of AI investment firm Pardoe Ventures, however, examples of projects that ultimately end in failure, such as the much-maligned A-Level algorithm, are far more to do with development and application, rather than the technology itself.
Get the ITPro daily newsletter
Sign up today and you will receive a free copy of our Future Focus 2025 report - the leading guidance on AI, cybersecurity and other IT challenges as per 700+ senior executives
“Typically, it is not the underlying technology itself that is at fault, but ultimately a lack of human intelligence that delivers a poor implementation of the technology,” Pardoe tells IT Pro. “Such shortcomings can be caused by confusion about requirements, lack of clarity on the desired outcomes, limited understanding of the technology being used, speed over quality, and not enough time for testing.
“The consequence of this manifests as unexpected or unintended analytical results and the finger of suspicion points firmly at the technology, but the reality is a combination of technical implementation and misaligned data used with the algorithm. While it’s easy to criticise in retrospection, the [A-Level results scandal] was the perfect storm of having to put in place an analytical solution rapidly without a comprehensive set of data that would allow the appropriate algorithmic adjustments to the predicted grades.”
Although the fiasco represents one of the loudest recent public AI failures, there are countless further examples. The infamous Tay experiment, in which an automated Twitter account began spouting messages of hate, for example, forced Microsoft into rethinking its approach to AI entirely. Even machine learning systems deployed in widely-used social media platforms, such as Facebook, are in a constant state of flux – seemingly tweaked by engineers on a trial-and-error basis. The most significant change to the firm’s Newsfeed algorithm, for example, arrived following the 2016 US presidential election only after it was found to have inadvertently amplified extremist content and widely-consumed fake news.
Many of these problems, especially when algorithms are used for decision-making, stem from poor data and limited diversity in datasets, according to Dr Nick Lynch, consultant and investment lead with the Pistoia Alliance. “Algorithms used in recruitment, for instance, are known to favour white men in many cases,” he explains. “In medicine and healthcare specifically, there is a heavy bias towards white men in clinical trials; adult males dominate the clinical trial population, and around 86% of participants are white. And when these data are used to “teach” an algorithm that informs decisions in drug discovery and healthcare, there is a risk of inaccurate and even harmful outcomes.”
Failure to launch
With many of these ‘dystopian’ systems hardly living up to the hype, many among us may ask whether we actually have anything to worry about. Yet, despite the ICO’s judgement that Cambridge Analytica’s tools weren’t as powerful as its clients were led to believe, there’s no doubting the intent. There are clearly entities determined to harness the power of data to manipulate voters, and, therefore, the political process.
While it may be tempting for the privacy-conscious to rest in the comfort of knowing that technologies such as facial recognition are failing to get off the ground, their failures are causing more damage in the short-term. Highly experimental deployments in a largely unregulated space has the makings of a disaster. This may apply to facial recognition misidentifying individuals, and subsequently dragging them through a needless legal ordeal, or a now-defunct A-Level algorithm causing untold mental distress to thousands of students. Regardless, had the A-Level algorithm actually worked as intended, it would have raised further ethical questions as to whether it’s acceptable for a machine to play such a crucial role in determining the futures of students.
Cambridge Analytica may not have been as potent as many claimed, but even the demand for such a service should frighten us. As reported by Channel 4 News, ahead of the recent US presidential election microtargeting of black and ethnic minority voters was used to try and dissuade them from casting their ballot. The clear appetite for the use of AI-powered technologies, as well as the nature of progress in this field, suggests it’s only a matter of time before ‘dystopian failure’ turns into success.
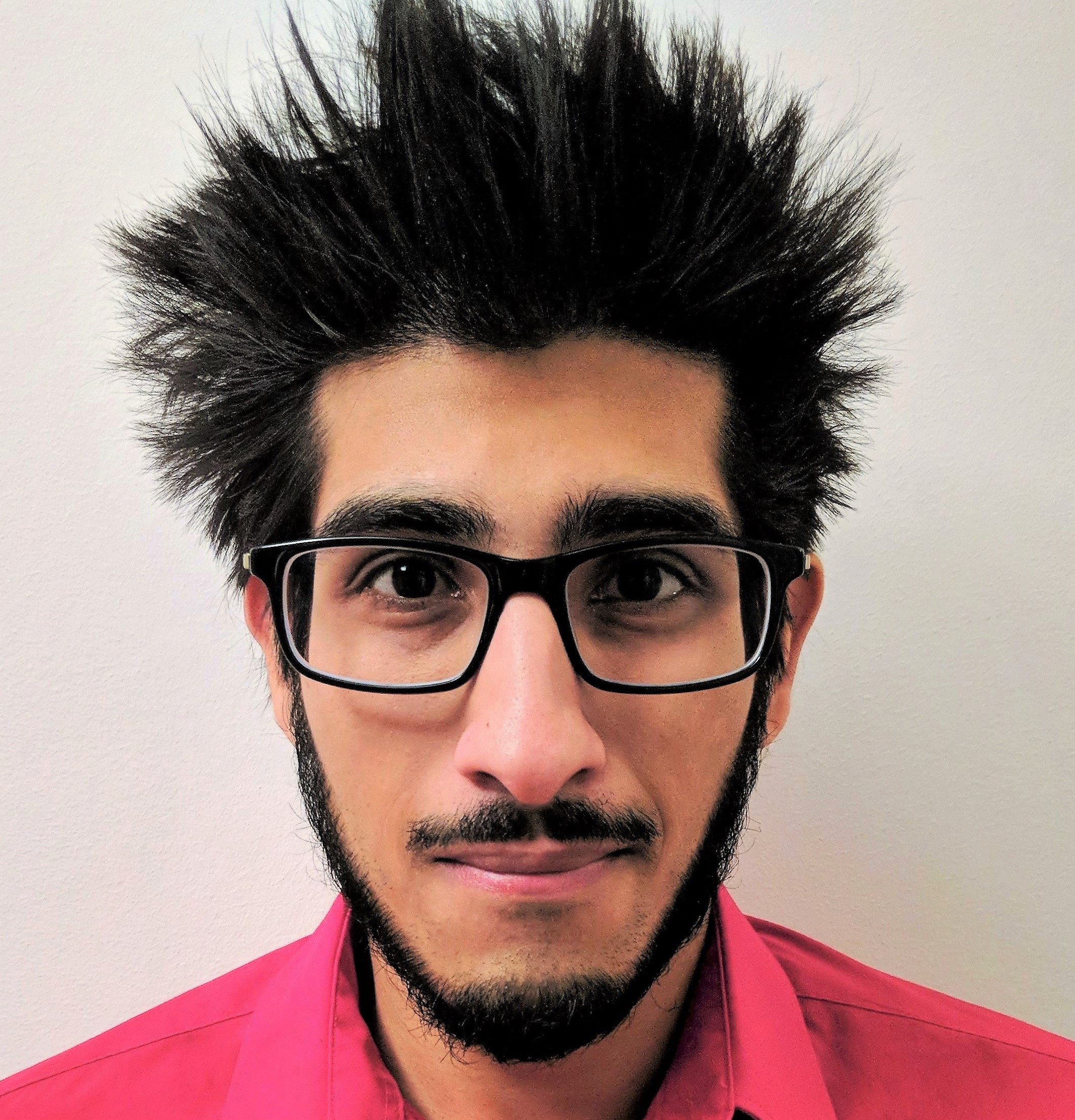
Keumars Afifi-Sabet is a writer and editor that specialises in public sector, cyber security, and cloud computing. He first joined ITPro as a staff writer in April 2018 and eventually became its Features Editor. Although a regular contributor to other tech sites in the past, these days you will find Keumars on LiveScience, where he runs its Technology section.
-
What is polymorphic malware?
Explainer Polymorphic malware constantly changes its code to avoid detection, making it a top cybersecurity threat that demands advanced, behavior-based defenses
-
Outgoing Kaseya CEO teases "this is just the beginning" for the company
Opinion We spoke to Fred Voccola who remains a key figurehead at the firm as it enters its next chapter...