Presented by AMD
What is an AI factory and what does it mean for enterprises?
This form of specialized computing infrastructure powers some of our most popular services today
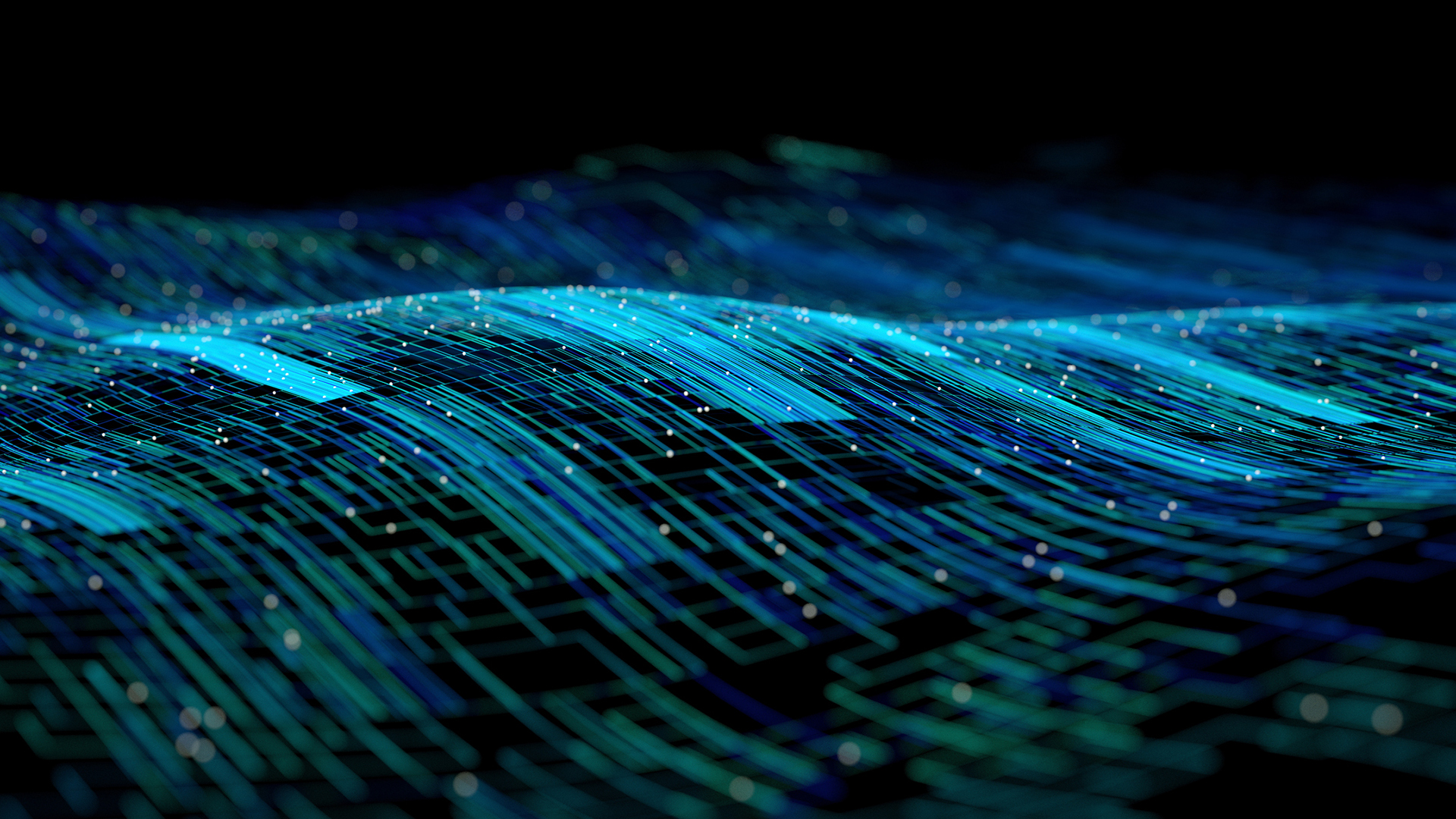
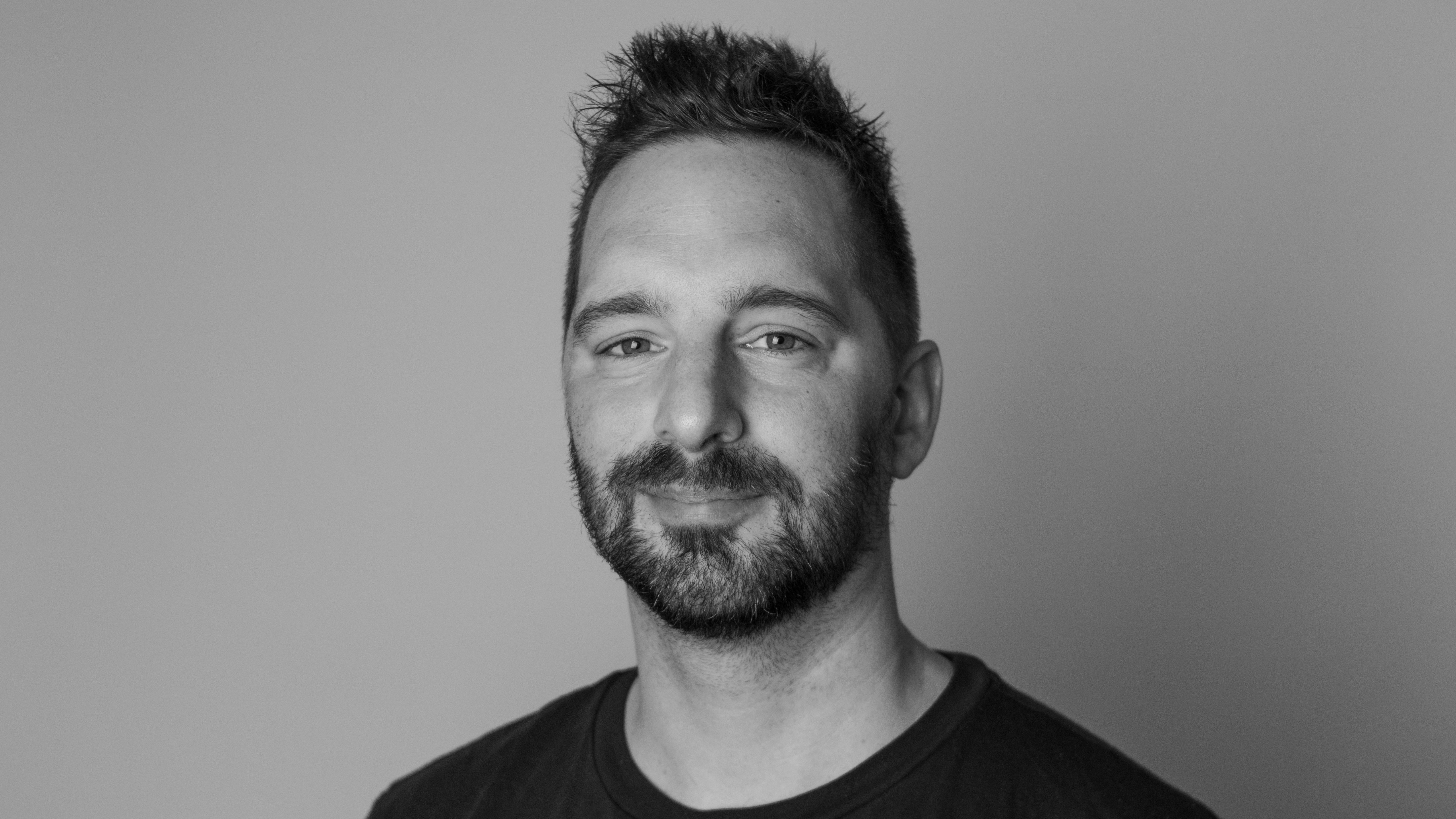
We may not realize how much we already use AI in our day-to-day lives. Some might even say we take it for granted. When we use streaming platforms, apps for transport, or even search engines, algorithms and machine learning are working away in the background.
It may be too bold to say that all companies will become AI companies, but it certainly can be said that AI will be a core part of most businesses in the future. This is because automation and generative AI have already exploded across organizations of all sizes over the last few years, becoming as ubiquitous as the cloud.
It’s the cloud and data-led technologies already in place that have helped to advance AI and its uses in business; with the information we generate and the insights that we draw from it, we now use it to power many of our products and services. Many of these are now done by companies themselves, through innovative AI factories.
What is an AI factory?
An AI factory is a form of specialized computing infrastructure that powers a digital operating model. Decision-making is treated as an industrial process, hence the term ‘factory’. The factory works in a cycle – data comes in, it’s used to run algorithms, and it predicts and improves. The more data available to the algorithms on an ongoing basis, the better they will get, which in turn leads to better services and increased usage.
Much like a traditional factory, where raw materials are transformed into products, in an AI factory, raw data is transformed into intelligence services. In other words, the main output of an AI factory is insight or decisions.
It’s important to draw a distinction between a data center, which runs a mixture of workloads and systems that may include some AI workloads, and an AI factory, which is more specialized and purpose-built for AI development.
There is much more to an AI factory than the collection, storage, and processing of data, however. They don’t simply retrieve data based on training datasets, but generate tailored content via tokens that can be seen as text, images, videos, and research outputs.
Get the ITPro daily newsletter
Sign up today and you will receive a free copy of our Future Focus 2025 report - the leading guidance on AI, cybersecurity and other IT challenges as per 700+ senior executives
For an AI factory, you need four core elements. The first is a data pipeline; a process or system that gathers information in a clean and compliant way. Then come predictive algorithms and machine learning; here, predictions about future outcomes, business actions, and potential success are made. The predictions are then tested before being used across the system or business.
For the data pipeline, algorithm development, and experimentation platform to work, you need sufficient software infrastructure. This is the backbone of an AI factory – a system of connected services – hardware, software, and networks – that manage data storage, processing, and its migration.
Servers and GPUs play an integral role here. Working in tandem to process vast amounts of data, run complex algorithms, and train AI models at speed and scale. This infrastructure of server technology will usually be meticulously designed to handle such immense computational power. A high level of computational output is required for training large-scale models and deploying them for real-time inference. Along with an advanced storage system that can manage and retrieve massive datasets, ensuring seamless data flow.
There is no underselling of the importance of GPUs for AI factories (or for AI in general, for that matter). Due to their ability in graphics rendering, GPUs have become one of the most key technologies in the AI era, enabling the training and deployment of AI models thought impossible not so long ago.
One example of an AI factory from a vendor would be the Dell AI Factory. It offers a multitude of hardware options, with PowerEdge XE7745, PowerEdge R6725, and PowerEdge R7725 servers featuring AMD 5th Generation EPYC processors recently added to the lineup.
A stream of AI
For businesses, investment in AI factories is a way to rapidly turn AI from a long-term research project into a driver of competitive advantage. In the same way that an industrial factory directly contributes to revenues, an AI factory takes data as the raw materials, with the finished product being intelligence on a massive scale.
One of the most famous examples of an AI factory is Netflix. The streaming service is essentially one giant AI factory, built with multiple machine learning algorithms that work with user data to provide recommendations and various improvements across the platform.
Netflix constantly captures user data and runs predictions and tests on it. It does so until it has a positive outcome, like a tailored list of recommendations or better, more enticing images and clips. It tracks what users watch and for how long. It takes in data on their behaviors while browsing content, such as how long they watched a trailer, what images they clicked on, what types of video clips they preferred and the devices they used. All this data is processed and used not only to recommend films and shows, but also for adapting thumbnail images and preventing customers from spending too long deciding what to watch or, worse, leaving the platform completely.
While Netflix may be the ur-example of an AI factory, they are now widely used across different sectors and industries, like search engines, transport apps, e-commerce sites, and even actual factories. The adoption is only going to increase as more and more companies adopt AI and see the potential.
Looking at these examples, AI factories can bring a wealth of benefits to small and large businesses alike. From transforming their raw data into revenues to boosting performance across their entire operation, AI factories provide a secure and adaptive ecosystem.
What’s more, an AI factory ensures a cost-effective way to scale the business by allowing companies to expand their AI capabilities and ultimately their outputs – and, yes, revenues.
Bobby Hellard is ITPro's Reviews Editor and has worked on CloudPro and ChannelPro since 2018. In his time at ITPro, Bobby has covered stories for all the major technology companies, such as Apple, Microsoft, Amazon and Facebook, and regularly attends industry-leading events such as AWS Re:Invent and Google Cloud Next.
Bobby mainly covers hardware reviews, but you will also recognize him as the face of many of our video reviews of laptops and smartphones.
-
Why DragonForce is growing in prominence
News Unusually low profit share demands and broad infrastructure could rocket the group into infamy
-
Venture capital's AI obsession is 'sucking the oxygen out of the room' for other startups
News Investors are growing concerned about the rise of 'zombiecorns' in the AI startup space
-
5th Gen Amd Epyc™ Processor Architecture
whitepaper
-
Advance Your Enterprise Ai Journey
whitepaper
-
How To Get Up To 20% More Ai Performance From High-performance Gpus
whitepaper
-
Maximize Gpu Efficiency With Amd Epyctm High-frequency Processors
whitepaper
-
What is exascale computing? Exploring the next step in supercomputers
60 years after the birth of the first supercomputers, we are entering a new era
-
What do you really need for your AI project?
Supported content From GPUs and NPUs to the right amount of server storage, there are many hardware considerations for training AI in-house
-
AMD reveals MI300 series accelerators for generative AI
Supported editorial MI300X is the star of the new family of processors with AMD ‘laser-focused’ on data center deployment
-
AMD and Microsoft cement relationship with cloud collaborations
The products offer Azure customers the possibility of running high intensity or AI workloads on powerful infrastructure, without having to house or maintain it themselves