Data quality issues in big data
To understand data quality issues in Big Data, you need to look at its main features
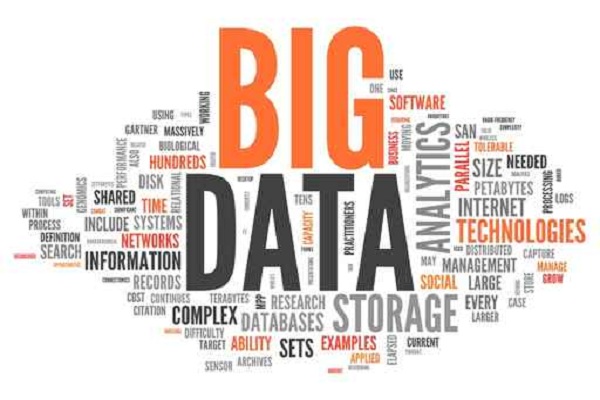
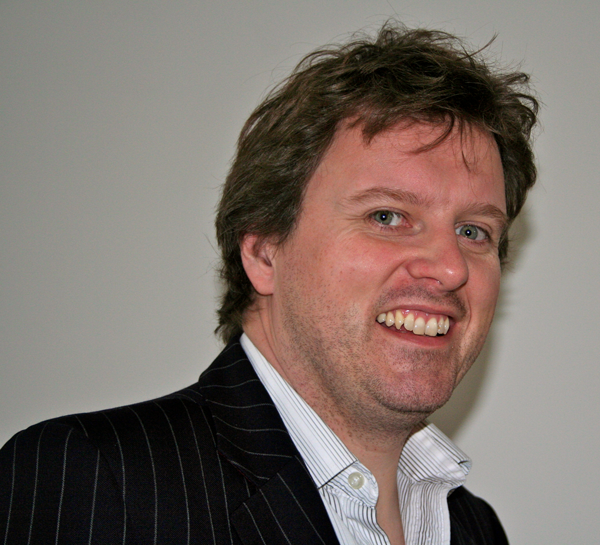
Data quality issues are as old as databases themselves. But Big Data has added a new dimension to the area; with many more Big Data applications coming online, the problem of bad data quality problems can be far more extensive and catastrophic.
If Big Data is to be used, organisations need to make sure that this information collection sticks to a high standard. To understand the problems, we need to look at them in terms of the important aspects of Big Data itself.
Velocity
The speed at which data is generated can make it difficult to gauge data quality given the finite amount of time and resources. By the time a quality assessment is concluded, the output could be obsolete and useless.
One way to overcome this is through sampling, but this is at the expense of bias as samples rarely give a truthful picture of the entire dataset.
Variety
Data comes in all shapes and sizes in Big Data and this affects data quality. One data metric may not suit all the data collected. Multiple metrics are needed as evaluating and improving data quality of unstructured data is vastly more complex than for structured data.
Get the ITPro daily newsletter
Sign up today and you will receive a free copy of our Future Focus 2025 report - the leading guidance on AI, cybersecurity and other IT challenges as per 700+ senior executives
Data from different sources can have different semantics and this can impact things. Fields with identical names, but from different parts of the business, may have different meanings.
To make sense of this data, reliable metadata is needed (e.g. sales data should come with time stamps, items bought, etc.). Such metadata can be hard to obtain if data is from external sources.
Volume
The massive size and scale of Big Data projects makes it nigh-on impossible to undertake a wide-ranging data quality assessment. At best, data quality measurements are imprecise (these are not absolute values, more probabilities).
Data quality metrics have to be redefined based on particular attributes of the Big Data project, in order that metrics have a clear meaning, which can be measured and used for evaluating the alternative strategies for data quality improvement.
Value
The value of data is all about how useful it is in its end purpose. Organisations use Big Data for many business goals and these drive how data quality is expressed, calculated and enhanced.
Data quality is dependent on what your business plans to do with the data; it's all relative. Incomplete of inconsistent data may not impact how useful the data is in achieving a business goal. The data quality may good enough to ignore improving it.
This also has a bearing on the cost vs benefit of improving data quality; is it worth doing and what issues need to take priority.
Veracity
Veracity is directly tied to quality issues in data. It relates to the imprecision of data along with its biases, consistency, trustworthiness and noise. All of these effect data accountability and integrity.
In different organisations and even different parts of the business, data users have diverse objectives and working processes. This leads to different ideas about what constitutes data quality.
Rene Millman is a freelance writer and broadcaster who covers cybersecurity, AI, IoT, and the cloud. He also works as a contributing analyst at GigaOm and has previously worked as an analyst for Gartner covering the infrastructure market. He has made numerous television appearances to give his views and expertise on technology trends and companies that affect and shape our lives. You can follow Rene Millman on Twitter.
-
M&S suspends online sales as 'cyber incident' continues
News Marks & Spencer (M&S) has informed customers that all online and app sales have been suspended as the high street retailer battles a ‘cyber incident’.
By Ross Kelly
-
Manners cost nothing, unless you’re using ChatGPT
Opinion Polite users are costing OpenAI millions of dollars each year – but Ps and Qs are a small dent in what ChatGPT could cost the planet
By Ross Kelly
-
Datadog Database Monitoring extends to SQL Server and Azure database platforms
News The tool offers increased visibility into query-level metrics and detailed explanation plans
By Praharsha Anand
-
Oracle and Microsoft announce Oracle Database Service for Azure
News Azure users can now easily provision, access, and monitor enterprise-grade Oracle Database services in Oracle Cloud Infrastructure
By Daniel Todd
-
Elastic expands cloud collaboration with AWS
News Partnership aims to ease migration to Elastic Cloud on AWS, as well as simplify onboarding and drive go-to-market initiatives
By Daniel Todd
-
Manage the multiple database journey
Whitepaper Ensuring efficient and effective operations across multiple databases
By ITPro
-
Automating the modern data warehouse
Whitepaper Freedom from constraints on your data
By ITPro
-
Freedom from manual data management
Whitepaper Build a data-driven future with Oracle
By ITPro
-
Oracle’s modern data platform strategy
Whitepaper Freedom from manual data management
By ITPro
-
Oracle autonomous database for dummies
Whitepaper Freedom from mundane, manual database management
By ITPro