How to get hold of the data science talent your business needs
A look at some of the key skills IT leaders should consider when looking for new hires
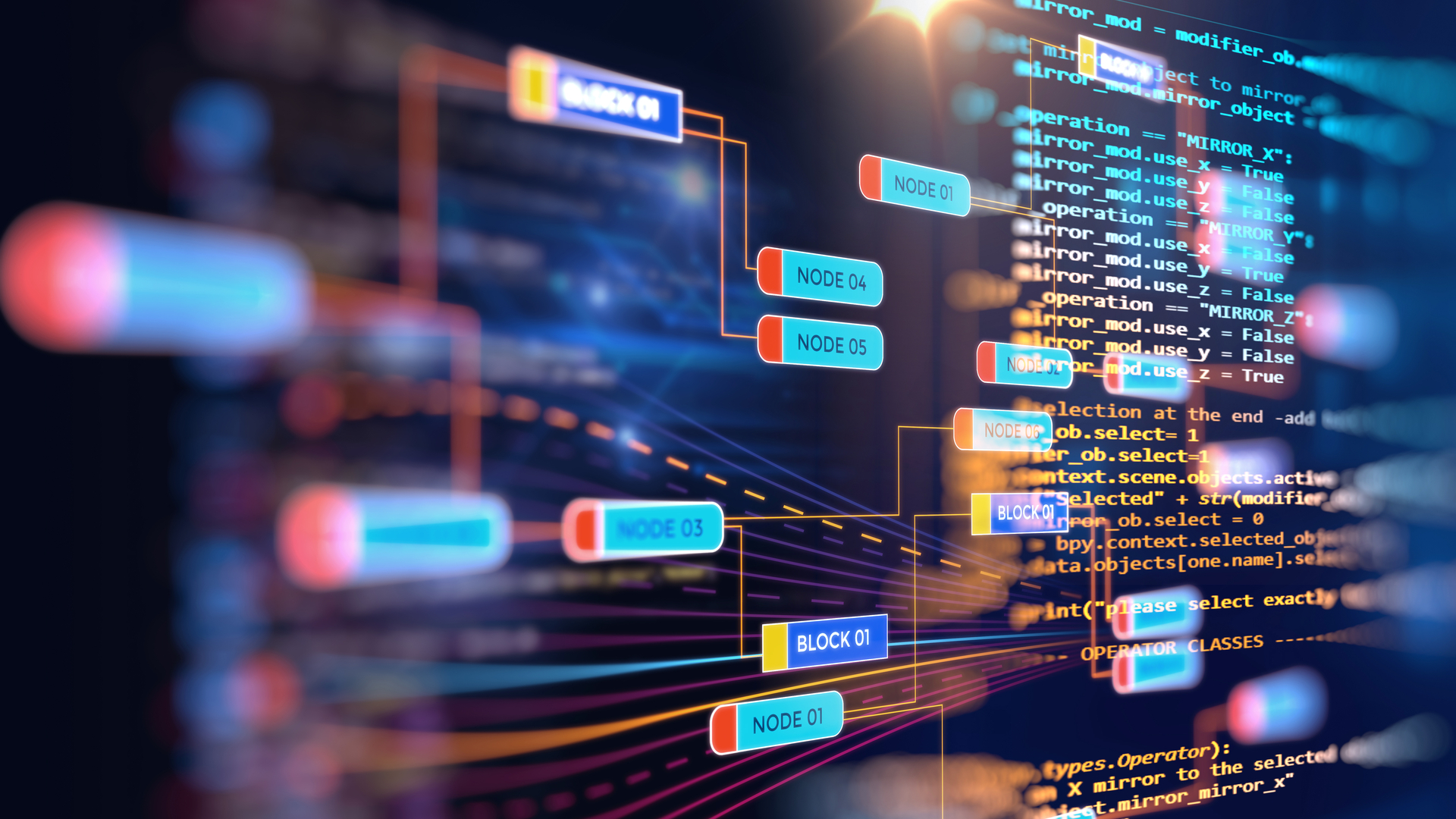
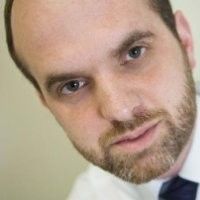
Data science is the talk of the boardroom. Executives in organisations across all sectors are looking for ways to find a business case for the practical application of data science through emerging technology, such as artificial intelligence (AI) and machine learning (ML).
Take Julie Dodd, director of digital transformation and communications at Parkinson's UK, who is using a partnership with tech specialist BenevolentAI to search for drugs that might offer a potential cure to the condition.
The BenevolentAI platform trawls clinical research data and searches for trends that humans might not be able to spot. The results come in the form of suggested drugs, molecules and pathways that could be beneficial in the future.
Dodd says AI reduces the time spent on research from years to weeks, with potentially life-changing impacts for people with Parkinson's. "AI is revolutionary and the impact will continue to grow," she says.
Executives in other industries are keen to explore the potential benefits of data science and emerging technology, too. The number of enterprises implementing AI grew 270% in the past four years, according to analyst Gartner's 2019 CIO Survey.
But recognising the importance of AI and ML is just part of the challenge. As much as 47% of European organisations are struggling to fill their data science roles, according to O'Reilly Media. So, what skills should IT professionals develop in order to meet market requirements and how can digital leaders source the talent they need?
Language vs thinking
Mohammad Shokoohi-Yekta, who was until recently a senior data scientist at Apple, and who now teaches a course at Stanford University called 'An Introduction to Data Science', says his experience suggests candidates are eager to upskill in a number of key languages.
Get the ITPro daily newsletter
Sign up today and you will receive a free copy of our Future Focus 2025 report - the leading guidance on AI, cybersecurity and other IT challenges as per 700+ senior executives
"Python and R -- I have classes in both of these," he says. "R is the most powerful for data visualisation, data analysis and training machine-learning models; Python is more general, is good for many tasks and is good for getting stuff into production quickly."
Claus Bentsen, executive director at pharmaceutical giant Astra Zeneca, who joined Shokoohi-Yekta on a panel at the recent Big Data World event in London, says his firm is interested in people with capabilities in R. However, he warns CIOs and would-be data scientists not to focus solely on languages.
"What matters is that you learn something and you become good at it," he says. "Make sure you get an understanding of an object-oriented paradigm and how it works. Being able to think functionally about programming is also important. In some ways, it's more important to have those thinking paradigms around computer science rather than developing a favourite tool."
Bentsen says he has seen people who've had other titles, such as statistician or mathematician, now wanting the data scientist title in order to meet the demand for AI and ML. That can make recruitment difficult -- and Bentsen says Astra Zeneca filters candidates to focus on quality.
"When we're looking for specific skills in teams, I run multiple tests. Rather than programming, I focus on maths and statistical capabilities. Some people can talk really well and the only way to see if they're good is to test them," he says.
That's a sentiment that chimes with Richard Freeman, who is lead data and machine-learning engineer at fundraising specialist JustGiving. He fears the term data scientist is "over-used" and the market could become flooded with wrongly badged talent. Freeman says his firm focuses on aptitude and passion -- and he advises other digital leaders to take a similar approach.
"I look at history and what someone has done. Are they a strong developer who wants to move into data science or machine learning? Look at the history of your candidates," he says, suggesting experience in coding is not necessarily the only route to data science success.
"We have people with a psychology background on our data science team. The reason they're good is that they're passionate about learning. Always go for passion -- you need to see that fire and they need to love data science. It's good to have a mix, but someone who's junior and will learn is often better than someone that's experienced and expensive."
Nurturing talent
The search for data science talent is something that might soon be an issue for Kieran Delaney, IT director at brewing specialist Innserve. His firm is beginning to make its first tentative steps towards engaging with ML and AI.
Delaney says the firm is lucky to benefit from having some "clever developers", yet these problem-focused experts are not data scientists. He believes the way to source talent is related to the accessibility of the toolsets the business uses -- and CIOs now have more options than ever before.
"One of the things we've seen with Azure is that Microsoft is trying to make AI and ML as easy as possible," he says. "You don't need to be an expert -- a developer with a low level of experience can begin to start using the technology and get something workable out."
Gary Foote, CIO at Rich Energy Haas F1, is another digital leader who is only just beginning to investigate how his Formula 1 racing team might make the most of emerging technologies, like AI and ML. His impression is that ML is best seen as a way to allow people to concentrate more on what they do best.
"Take a generic race engineer -- part of their job will be something that machine learning can do. If I can deploy machine-learning technology, then I can allow that engineer to focus on some of the areas that he can add value where machine learning can't," says Foote.
As an example, he refers to the huge amounts of images generated by processes around the business, particularly during testing and race weekends. These images have to be analysed -- and that can be a time-intensive process.
Foote says the power of machine learning might allow his team to trawl thousands of images quickly. Engineers could use these results to find key data points to help the team make crucial business decisions. "Then, all of a sudden, maybe a one-hour job becomes a ten-minute job," he says.
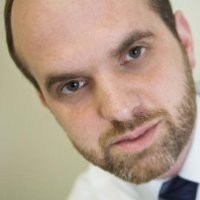
Mark Samuels is a freelance writer specializing in business and technology. For the past two decades, he has produced extensive work on subjects such as the adoption of technology by C-suite executives.
At ITPro, Mark has provided long-form content on C-suite strategy, particularly relating to chief information officers (CIOs), as well as digital transformation case studies, and explainers on cloud computing architecture.
Mark has written for publications including Computing, The Guardian, ZDNet, TechRepublic, Times Higher Education, and CIONET.
Before his career in journalism, Mark achieved a BA in geography and MSc in World Space Economy at the University of Birmingham, as well as a PhD in economic geography at the University of Sheffield.
-
NHS set for huge tech investment boost in latest spending review
News The government spending review will fund the NHS App, the single patient record, the Federated Data Platform, and other initiatives
-
Everything we know so far about the United Natural Foods cyber attack
News The attack on United Natural Foods, a major US distributor, severely disrupted systems