A new hugging face vulnerability could spell trouble for AI as a service providers
New research has found issues in Hugging Faces architecture that could put models across the platform at risk
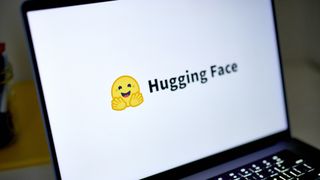
Researchers have raised concerns over vulnerabilities that could compromise AI as a Service providers operating on Hugging Face by uploading custom-made malicious models.
Analysis from Wiz showed researchers were able to run arbitrary code after uploading tampered models to Hugging Face, leveraging this within Hugging Face’s inference API feature to gain escalated control.
Attackers could have a “devastating” impact on the Hugging Face environment were they to successfully utilize these vulnerabilities, the study found, granting them access to millions of private AI models and applications.
Worryingly, Wiz doesn't believe that these findings are in any way unique, with researchers citing this as a likely ongoing challenge for the AI as a service industry.
“We believe those findings are not unique to Hugging Face and represent challenges of tenant separation that many AI as a service companies will face,” the Wiz researchers said.
“We in the security community should partner closely with those companies to ensure safe infrastructure and guardrails are put in place without hindering this rapid (and truly incredible) growth,” they added.
This vulnerability throws up serious concerns for those looking to either use or provide AI as a Service, adding a new and novel AI-related attack path for enterprises to be concerned about.
Get the ITPro. daily newsletter
Receive our latest news, industry updates, featured resources and more. Sign up today to receive our FREE report on AI cyber crime & security - newly updated for 2024.
How does this vulnerability work?
Wiz’s research led them to define two critical risks present in the Hugging Face environment that a theoretical threat actor could have taken advantage of.
In the first instance, termed a “shared inference infrastructure takeover” risk, the researchers leveraged the process of AI inference, in which a trained model is used to generate predictions for a given input.
Wiz found that inference infrastructure often runs “untrusted” models that use the “pickle” format. A “pickle-serialized” model could contain a remote code execution payload, granting a threat actor enhanced privileges or cross-tenant access.
In the other form of attack, termed a “Shared CI/CD takeover” risk, threat actors could compile malicious AI applications with the intent of gaining control over the CI/CD pipeline to perform a supply chain attack, again paving the way for privileges and access.
Attackers could also set their sights on different components through varied methods, attacking, for example, an AI model directly through inputs to create “false predictions.”
Wiz made clear the need for developers and engineers to operate with a heightened sense of caution when downloading models, as untrusted AI models could introduce evident security risks into an application.
Another mitigation approach advised by Wiz Research was to enable IMDSv2 with Hop Limit to prevent pods from accessing the IMDS and obtaining the role of a node within the cluster.
“This research demonstrates that utilizing untrusted AI models (especially Pickle-based ones) could result in serious security consequences,” the Wiz researchers said.
“Organizations should ensure that they have visibility and governance of the entire AI stack being used and carefully analyze all risks, including usage of malicious models, exposure of training data, sensitive data in training, vulnerabilities in AI SDKs, exposure of AI services, and other toxic risk combinations that may exploited by attackers,” they added.
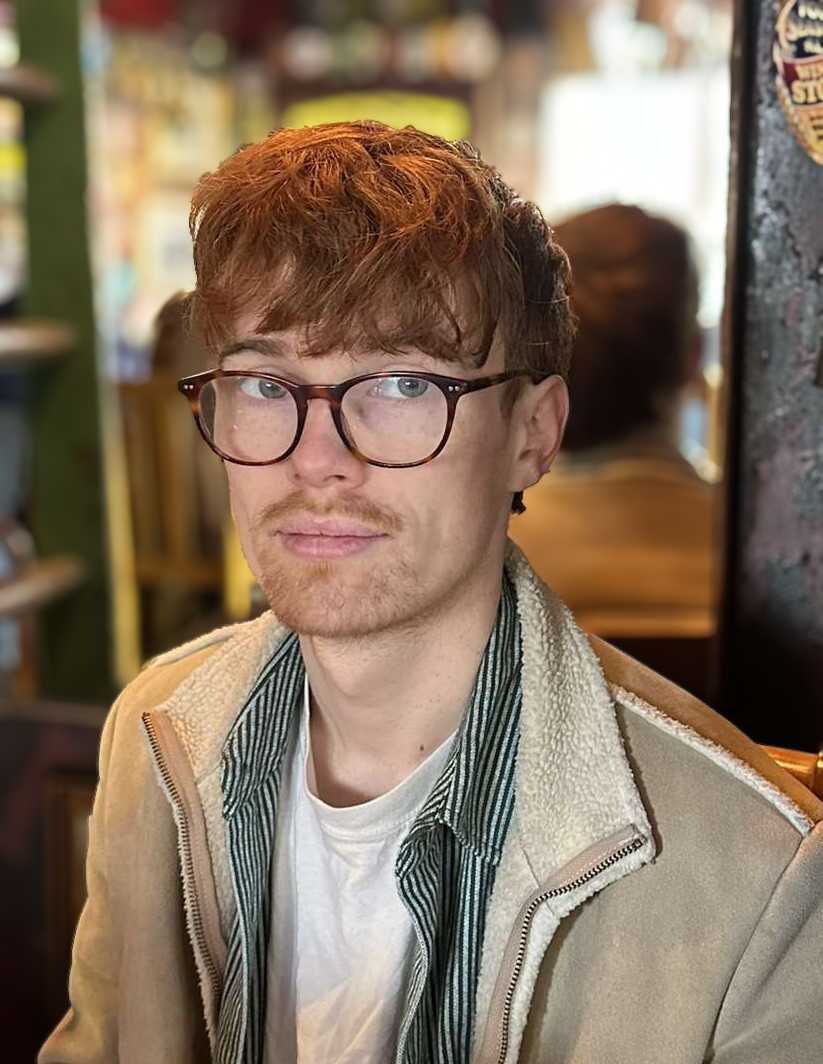
George Fitzmaurice is a staff writer at ITPro, ChannelPro, and CloudPro, with a particular interest in AI regulation, data legislation, and market development. After graduating from the University of Oxford with a degree in English Language and Literature, he undertook an internship at the New Statesman before starting at ITPro. Outside of the office, George is both an aspiring musician and an avid reader.