DeepMind algorithm gives ‘memory’ to AI
AI systems are developing memory by playing video games such as Atari
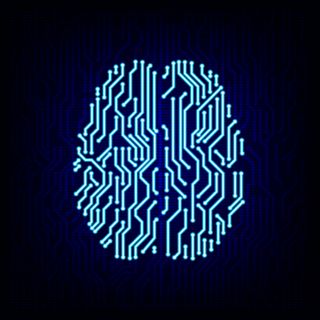
AI is learning memory by playing Atari.
Google-owned DeepMind has created an algorithm that provides the company's machine learning systems with a "memory" by enabling continual learning in neural networks, working with a group of researchers from Imperial College London.
According to DeepMind's recent PNAS paper, the elastic-weight consolidation (EWC) algorithm was created as an approach to overcome "catastrophic forgetting" in neural networks. It was inspired by neuroscience-based theories on the consolidation of learned skills and memories in human and mammalian brains.
To test the algorithm, an agent played back-to-back games of Atari. When EWC was not used, the agent quickly forgot the game it had just played. When the EWC was used, the agent did not forget as quickly.
The findings indicated that the company's AI systems learned to play different games using information it had learned from playing the prior games, highlighting the potential for the systems to develop a memory.
"The ability to learn tasks in succession without forgetting is a core component of biological and artificial intelligence," noted the PNAS paper.
In 2014, DeepMind began training its machine-learning systems to play Atari games. While the systems learned how to play, sometimes outperforming humans, the need for separate neural networks prohibited the systems from remembering how they did so.
Get the ITPro. daily newsletter
Receive our latest news, industry updates, featured resources and more. Sign up today to receive our FREE report on AI cyber crime & security - newly updated for 2024.
"We show that the learning rule can be modified so that a program can remember old tasks when learning a new one," a DeepMind blog post explained. "This is an important step towards more intelligent programs that are able to learn progressively and adaptively."
DeepMind hopes this new algorithm acts as a step forward towards creating programs that can learn more effectively.