AI risks harming scientific research, study warns
The Royal Society warns that results can be unreliable or untrustworthy, and poor at solving real world problems
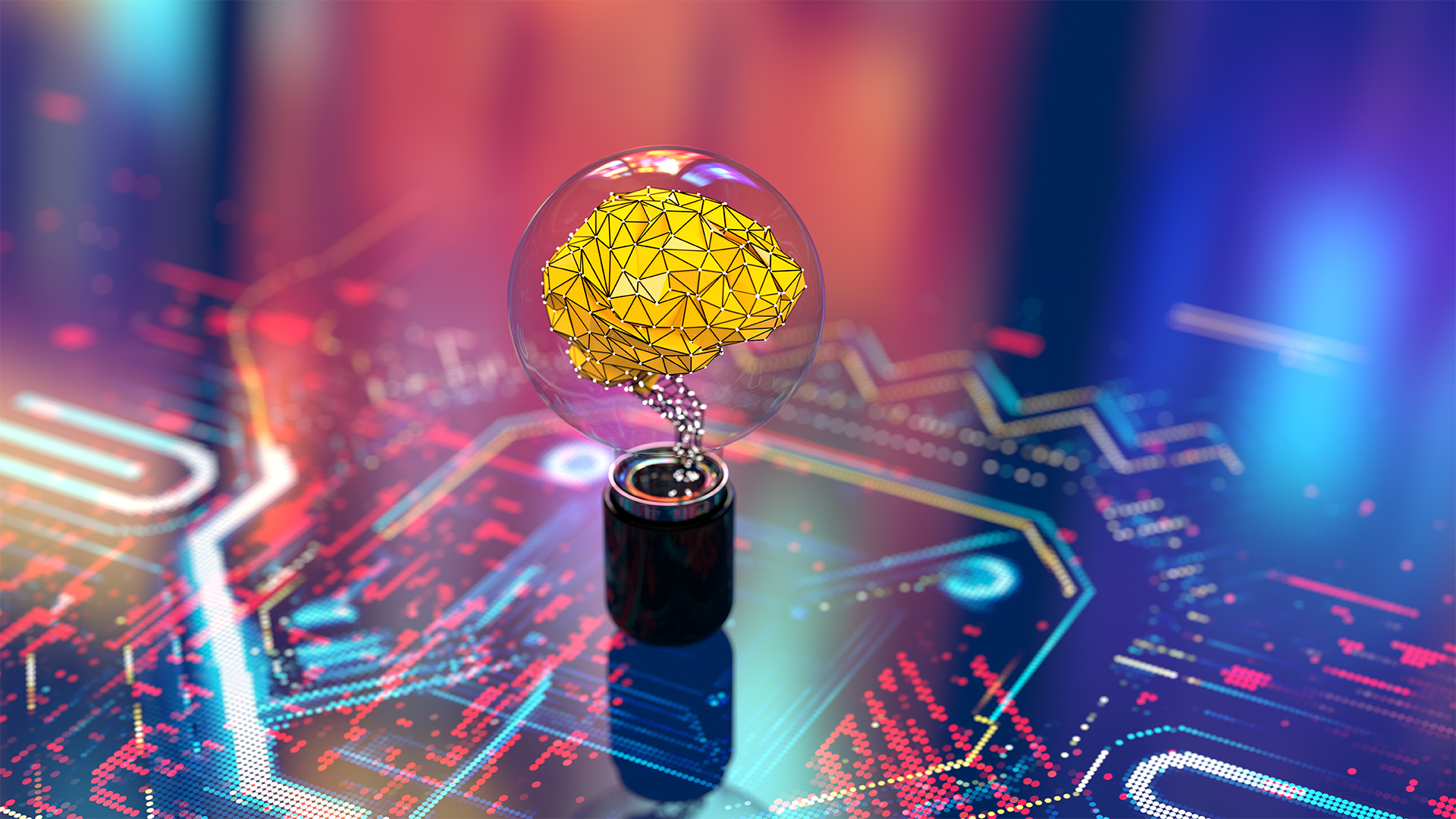
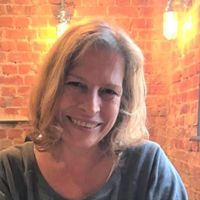
Overdependence on ‘opaque’ AI systems in research could make scientific findings less reliable and less useful in the real world, a report by the Royal Society has found.
The complexity and ‘black box’ nature of sophisticated machine learning models mean their outputs can't always be explained by the researchers using them.
This, researchers warned, can make them unreliable or untrustworthy, and limits their usefulness in terms of solving real world challenges.
"This report captures how the rapid adoption of AI across scientific disciplines is transforming research and enabling leaps in understanding that would not have been thinkable a decade ago," said Professor Alison Noble, vice president of the Royal Society and chair of the report’s working group.
"While AI systems are useful, they are not perfect. We should think of them almost like a scientific peer; they can offer valuable insights, but you would expect to be able to verify them yourself – and that isn’t always the case with current AI studies."
Challenges identified by the report include reproducibility, in which other researchers can't replicate experiments carried out using AI tools; interdisciplinarity, where limited collaboration between AI and non-AI disciplines can lead to a less rigorous uptake of AI across domains; and the environmental costs of the high energy consumption required to operate large compute infrastructure.
The report warned there are other factors too - such as pressure on scientific researchers to be ‘good at AI’ rather than ‘good at science’. Changing incentives across the scientific ecosystem, the report suggested, are pushing them to use advanced AI techniques at the expense of more conventional methodologies.
Sign up today and you will receive a free copy of our Future Focus 2025 report - the leading guidance on AI, cybersecurity and other IT challenges as per 700+ senior executives
However, the report also details the opportunities for the use of AI in scientific research, used particularly in fields such as medicine, materials science, robotics, agriculture, genetics, and computer science.
The main techniques include artificial neural networks, deep learning, natural language processing (NLP), and image recognition.
The report noted that while China and the US lead on, respectively, the number and value of patents filed, the UK is well positioned for growth. While it ranks tenth globally on patents, the UK holds second place in Europe behind Germany, and has a 14.7% share of the AI life sciences market.
"Science is constantly enjoying the introduction of disruptive new methodologies, from the microscope to computers, but adoption can be limited by things like cost, availability of the technology, and skills," said Dr Peter Dayan, director of the Max Planck Institute for Biological Cybernetics and a member of the working group.
"Now machine learning is set to transform vast swathes of research, we need to ask what that requires in terms of access. The report's recommendations look at how we can achieve that confluence of high-quality data, processing power and researcher skills in a suitably equitable manner."
This involves establishing ‘open science’, environmental, and ethical frameworks for AI-based research to help make sure that findings are accurate, reproducible and support the public good.
There should also be investment in ‘CERN-style’ regional and cross-sector AI infrastructure, to ensure all scientific disciplines can access the computing power and data resources to conduct rigorous research and maintain the competitiveness of non-industry researchers.
Finally, the report recommends, scientific researchers should be encouraged to become more AI-literate, and they should collaborate with developers to make sure their tools are accessible and usable.
Emma Woollacott is a freelance journalist writing for publications including the BBC, Private Eye, Forbes, Raconteur and specialist technology titles.
-
New chapter, same partners: Keeping the channel aligned with change
Industry Insights How to maintain strong channel partnerships amid evolving strategies and market change
-
Palo Alto Networks snaps up CyberArk in identity security push
News The acquisition marks the latest in a string for Palo Alto Networks