What is natural language processing?
Through natural language processing, AI developers are able to create systems capable of meaningful conversation
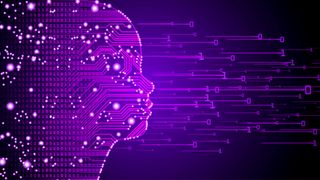
Natural language processing, a form of machine learning, is one of the hardest tasks researchers have faced in creating advanced AI systems. Yet it has the potential to greatly improve people’s lives, including how they access information or carry out everyday administrative tasks.
The premise sounds simple – natural language processing, or NLP, allows humans to make requests of a system as if they were talking to another human. In reality, however, humans and machines interact with data in very different ways.
For example, if you saw someone you live or work with heading outside you might ask where they’re going. If they’re going to the shops, they could answer in any number of ways, from “I’m just headed to the shops” to “nipping to the supermarket” to “off to buy some bread”. As a human, abstract thinking and experience will distil all of this information down to deliver the same information.
A computer, however, doesn’t have the benefit of either of these things, making it hard for it to understand these variations on a theme. Instead, it relies on pre-set instructions to carry out a defined operation.
Natural language processing seeks to overcome these limitations, making data sorting and retrieval easier for both parties.
One common use case for natural language processing now is telephone switchboards. If you make a call to a bank, for example, it’s not unusual to be met by an automated voice welcoming you to the bank’s customer service line and asking “what would you like to do today?” or something similar.
Thanks to natural language processing, you don’t have to say an exact phrase – “make a payment” and “pay someone” would be interpreted the same way.
Get the ITPro. daily newsletter
Receive our latest news, industry updates, featured resources and more. Sign up today to receive our FREE report on AI cyber crime & security - newly updated for 2024.
This is a significant improvement on existing automated systems that relied on a menu requiring either an exact phrase or keypad input.
Although it might seem strange at first, automating customer service is a natural progression of implementing AI in our daily lives. In fact, it brings in a bevy of benefits, from reducing workload of staff members to even contributing to a safer working environment by limiting the amount of verbal abuse received from frustrated customers.
Natural language processing: It's a complex problem
However, having an effective conversation or reading text is rife with nuances, inferences and judgements. It's one thing to break a language up into nouns, verbs, adjectives and the rest, but language is about far more than the various mechanisms that structure a sentence - and it's this added complexity that makes it difficult for computers to interpret and mimic human interaction.
The same word can have different meanings depending on the context in which it is used. Consider this example: the 'aeroplane banked to the left', or 'I banked the cheque you gave me'. How about 'I stood at the bow of the ship' compared to 'Mark tied his shoelaces into a bow' - not to mention the act of bowing to an audience or the device used to shoot an arrow.
Knowing how 'bow' is used in each of those sentences depends on the context provided by the surrounding words. Yet sometimes the context of the surrounding words doesn't help either. For example, consider "I saw the hiker on the path with binoculars". Who has the binoculars - me or the hiker?
The English language is heavily reliant on context, something that non-English speakers have difficulties with, never mind a machine. And that's before we start factoring in various highly-personal traits that characterise the person speaking, such as irony, sarcasm and humour, which even us humans can find difficult to infer from sentences alone.
Natural language processing: What is it capable of?
Natural language processing helps overcome any problems associated with understanding spoken language by combining data and algorithms to create context. There are two main aspects to how this works - syntax and semantics.
Syntax is by far the easiest of the two to apply, given that it deals with clearly defined rules. This includes dictionary definitions, the structure of sentences and, most notably, parsing, which relates to understanding what the sentence actually means. With the 'binocular' example above, parsing helps to identify who they belong to by using earlier or later sentences.
With natural language processing, algorithms are able to apply a number of different syntactic processing techniques to help break down these rules. These include: 'word segmentation', which dissects large chunks of text into distinct sections; 'stemming', which involves refining an inflected word to its root form; and morphological segmentation, which involves breaking a word down into its smallest meaningful units, such as 'in', 'come', '-ing' for 'incoming'.
Semantics, however, is far less structured and usually the more difficult to interpret. This aspect involves understanding the meaning of individual words within the broader context of the sentence. As with the examples above, this is understanding the various and most appropriate uses of the word 'bow'.
Semantic analysis can include 'named entry recognition', which involves figuring out which pieces of text map to proper names, and then determining what type of name it is, whether that's a person's name or the name of a location. Systems can rely on the convention of capitalising the first letter to easily identify a given name, however, this is also true of the first letter of a sentence and many organisation names do not capitalise every letter. What's more, capitalisation rules are not uniform across languages - in German, every noun is capitalised, for example.
This is symptomatic of semantic analysis in general – although there are techniques available, there are a number of hurdles still left to overcome.
Natural language processing and generative AI
Another place people will increasingly be encountering natural language processing is when they interact with generative AI, such as ChatGPT or Microsoft Copilot.
While GenAI and NLP aren’t the same thing, AI chatbots use natural language processing to interpret users’ requests and generate a unique response. This takes the NLP journey a step further and enhances the experience of the person interacting with the chatbot.
With the excitement, interest and – importantly – investment in advanced AI that has been generated since the launch of ChatGPT in November 2022, the capabilities of NLP will doubtlessly increase in the future.
Dale Walker is the Managing Editor of ITPro, and its sibling sites CloudPro and ChannelPro. Dale has a keen interest in IT regulations, data protection, and cyber security. He spent a number of years reporting for ITPro from numerous domestic and international events, including IBM, Red Hat, Google, and has been a regular reporter for Microsoft's various yearly showcases, including Ignite.