Fighting wildfires with sensors, AI and drones
Wildfires are dangerous to battle, although machine learning predictions and sensor-based alerts could make fighting them safer and more successful
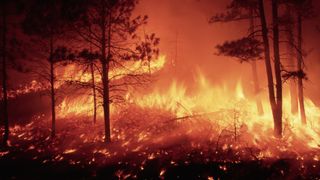
The past few years have seen record wildfires in California, Europe and Australia, with the annual conflagrations worsened by hot, dry conditions caused by climate change. That makes fighting such fires all the more costly – financially and in terms of firefighters’ lives.
Even the UK faces more frequent and more intense wildfires, according to research by the University of Reading. The best solution is to reduce greenhouse gas emissions to rein in climbing temperatures as much as possible, but technologies such as sensors, artificial intelligence (AI) and drones are also lending a helping hand to predict, track and fight fires, helping to reduce the damage and keep firefighters out of harm’s way.
There’s a host of ways firefighters already use such technology. UK company FireAngel uses AI to predict incidents for at-risk people, such as dementia sufferers, while US government agency NIST developed AI to predict flashovers. This is the phenomenon when items in a room ignite all at once, only limited by the amount of available oxygen.
San Francisco startup Qwake Technologies, meanwhile, has developed a heads-up display (HUD) using augmented reality (AR) in helmets to help firefighters see their way through a fire, while 3M’s Scott Sight embeds thermal imaging into helmets. Then there’s our old friend Dubai, who have flaunted – but are yet to use – jet packs for fighting fires in tall towers. They’ve also developed jet skis called “dolphins” for accessing coastal conflagrations.
The best way to fight fires, though, is to predict where they’re going to happen – and spot them as soon as they light up. Machine learning systems to do just that are under development in the UK, and could help firefighters around the world.
Tracking where a fire plans to go
While the UK hasn’t been hit by wildfires at the scale of those seen in Australia, California or in Mediterranean countries, we are home to leading wildfire research. This is particularly true for the Leverhulme Centre for Wildfires, Environment and Society, where Dr Rossella Arcucci, a data scientist from Imperial College, leads efforts to bring machine learning to the wildfire fight.
That’s possible because the Leverhulme Centre for Wildfires has data ready to work with. Some AI systems are data-led, requiring training on massive sets of data. But others apply general models to existing data sets to help make sense of them. The latter is what Arcucci and her team of researchers are developing. “The models we develop are to be applied to lots of different applications, because we start from the point that we have data,” she explains. “The meaning behind the data comes from collaboration with experts in the field. As soon as you have good data and some physical meaning… you can work on the models.”
Get the ITPro. daily newsletter
Receive our latest news, industry updates, featured resources and more. Sign up today to receive our FREE report on AI cyber crime & security - newly updated for 2024.
One project sparked by these ideas is led by Dr Sibo Cheng, using data assimilation and machine learning algorithms to improve and accelerate wildfire forecasting. His aim is to build a system using a recurrent neural network that lets anyone fighting a fire enter a few key bits of information and receive a prediction on how the fire will behave and where it will go next.
While Cheng’s wildfire work is data-led, there isn’t actually enough data on wildfires, as they remain rare enough incidents. To fuel his machine learning project, Cheng uses a physics-based simulator that simulates how fires grow and move. That model is already widely used to study fires, but running it is time consuming, meaning it’s not helpful for fighting active wildfires. “It will take several days or even a week to run a high-fidelity simulation,” he says. “So we’re investigating how to use machine learning to speed up the prediction of wildfires by learning from these physics-based simulations.”
Ten critical factors for cloud analytics success
Cloud-native, intelligent, and automated data management strategies to accelerate time to value and ROI
The system is fed with compressed satellite photos of the fire, as well as key inputs such as time and location, as well as wind direction, local vegetation, slope and elevation. The model then looks at the area that’s already been scorched to understand how it’s propagated so far. A few seconds later, it will make a prediction based on that data, which can be handed to on-the-ground experts to help aid their decision making.
The aim, says Cheng, is to deliver a prediction about any wildfire in almost real-time, to help firefighters make better decisions and ensure evacuations happen effectively. “We’ll be able to predict with some certainty and uncertainty where a fire is most likely to propagate, so we’ll have a smart way to distribute the resources for firefighters,” he explains.
Arcucci adds that such “nowcasting” is important as it helps save lives of firefighters and local residents as well as reduce how forests are impacted by wildfire. Simulating different scenarios gives decision makers all the tools they need to take the best course of action.
Of course, no simulated system is perfectly accurate. So Cheng is pairing the simulator-based model with another technology that will act as a real-time predictive autocorrect for the system. “To make a real-time correction, we’re using a technology called data assimilation, which means that when you make predictions, you observe what is actually happening, and take a sort of smart average between what you simulate and what you observe,” he says. “In that way, you can better adjust your predictive model for the current time, and future predictions that can be more accurate. It’s an iterative process.”
So far, the system hasn’t been used on live fires, but tested on wildfires after the fact, with photos fed into the model from early on during an incident and the predictions compared with the eventual real-life outcome. “It’s not yet perfect,” he says, “but overall we have some quite good results, it’s quite encouraging.”
Arcucci notes, however, that what a mathematician deems imperfect is different from what’s useful in the field. If the predictions are out by a few metres here and there, that’s mathematically imperfect and will be tweaked in the algorithm – but it’s still incredibly useful data to firefighters on the ground. “For us mathematicians, we say ‘oh, it’s not perfect’ – but for people working in the field, it may be perfect,” she says.
Next, the team hopes to set up a user interface to make it easier to input the data for anyone, so firefighting teams don’t need Cheng to make use of the system. Plus, there’s work to apply the idea more widely by using social media. While the machine learning model works in near real-time, it requires satellite photos – and those take hours to download and process. To help remove that half-day delay, another Leverhulme researcher is looking at pulling in social media photos to spot where fires are happening, supplying images as well as geolocation. That requires smart filtering – someone posting a hot new outfit and declaring “I’m on fire today,” shouldn’t spark a call to emergency services – but if such a system can be made to work it could become an additional fire alert system globally.
Fire sensors by drone
What if we didn’t wait for a human to spot – and then tweet about – a fire? That’s the idea behind a system designed by Izzet Kale, head of the department of engineering at the University of Westminster. “Once you’ve spotted [a fire], it’s too late – you need to identify it very quickly and very early,” he says.
His solution is to drop hundreds of sensors across a fire-prone forest, pull in the data they collect in real time, and analyse it to spot if a fire has started by watching for rising temperatures or specific gases. To avoid unnecessary firefighter callouts, the system will automatically deploy a drone to fly over any potential fires to snap photos to confirm the sensor’s data indicates a new fire and isn’t just caused by an unseasonable sunny spot.
The research came out of a wider project into wildfires funded by the EU several years ago, that looked at detecting and better understanding fires; necessary as Mediterranean countries suffer worsening effects from climate change sparking dangerous fires in dry landscapes. “We’ve seen this every summer in Spain, in Portugal and Greece, with lives lost,” Kale says.
The sensor system’s aim is to spot fires as soon as they start, as well as to give information to firefighters on where and how to battle it. Plus, says Kale, the information gathered can shape evacuation efforts, so people aren’t accidentally sent into zones that are about to become dangerous. “For example, I think it was the Spanish fire last summer, lots of people were not killed because of the fire but because of smoke poisoning, because they drove into an area trying to escape fire where there was poisonous smoke,” he says. “Had they had an advanced sensor network, they would have known what the wind direction was, what the wind speed was, what the gas concentrations were, and which direction the smoke was going to go or was going in real-time. So you could actually have crowd control in this.”
The low-power wireless ground sensor nodes, as they were called, are set-and-forget. They have long battery life and harvest energy from their surroundings, Kale explains, waking up now and then to take readings of temperature, oxygen and carbon dioxide levels, humidity and other parameters, sending the data back to a server. The sensors also always collect their location, in case they’re moved by an animal.
Each sensor has a range of about 200 metres, though that depends heavily on local landscape; a large flat area will have better coverage. Installing them by hand was naturally onerous, as the aim is to cover as much of a forest as possible to spot fires not seen initially by humans. To help, the team miniaturised the sensors and equipment as much as possible, tucking them inside tiny balls – and then shot them out of drones. “The drones will drop them at any location on a map, and the sensor will active and go live,” says Kale.
The data collected by the sensors was augmented by intelligent systems: it was compared to a profile for the area built up over time, to help spot false alarms from sudden temperature changes. Basically, the sensor collects enough data to be able to spot when something goes wrong. “We can put some intelligence into it and distinguish between something that is a false alarm and something that is real,” Kale says.
Plus, there’s the drones. Once a threshold is met by sensor data, a drone is automatically sent out with the coordinates to fly over and collect photos as well as take chemical analysis of the local terrain, to better estimate how the local plants will burn. That data would then be passed to local emergency services. “Here is the biomass burning, here is the map of where the fire is now, and where it will be in five minutes,” he says.
Won’t the sensors burn up in case of a fire? Yes, some do – but you’ll know there’s a fire there, at least. “We make these out of high-temperature materials,” Kale tells us. “As a fire went, some of them died, and some of them continued to give information. When the fire comes to it and it stops working, you know that the fire is on it.”
At this point, firefighters could be deployed to the scene, but wildfires are dangerous and difficult to fight, so researchers came up with a system to fight wildfires by dropping biodegradable pellets from a cargo plane to smother it. “It was precision bombing with coordinates,” he says of that aspect of the project.
Did it work? “It wasn’t flawless, but it was almost foolproof in the sense that our sensors on the ground augmented information,” says Kale. “Once it decided it was a fire, we had a visual very quickly, and that was 100% accurate – you can’t really go wrong.”
So far, trials have happened in Greece, Spain and Israel, and Kale is hoping to commercialise the system to roll it out more quickly – especially as fires worsen each year. “The world has to move in the direction of knowing exactly what they’re fighting, what they’re dealing with,” he said. “It will save lives.”