Researchers show how hackers can easily 'clog up' neural networks
The Maryland Cybersecurity Center warns deep neural networks can be tricked by adding more 'noise' to their inputs
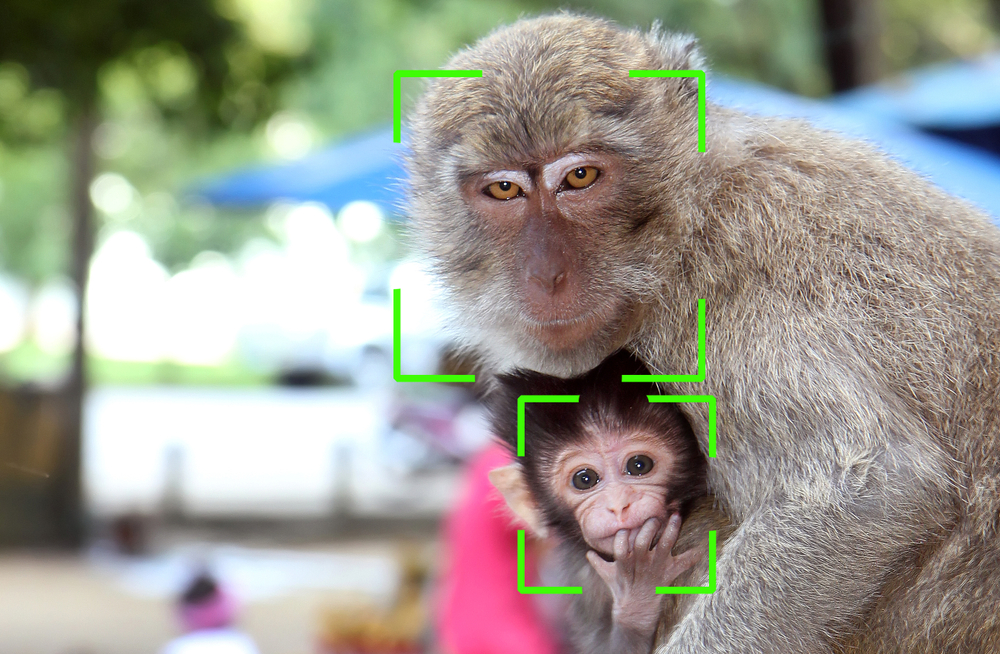
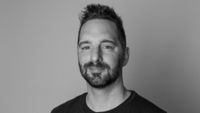
Researchers have discovered a new method of attack against AI systems that aims to clog up a network and slow down processing, in a style similar to that of a denial of service attack.
In a paper being presented at the International Conference on Learning Representation, researchers from the Maryland Cybersecurity Center have outlined how deep neural networks can be tricked by adding more "noise" to their inputs, as reported by MIT Technology Review.
What is an artificial neural network? Speak easy: How neural networks are transforming the world of translation AWS claims to have blocked the largest DDoS attack in history
It specifically targets the growing adoption of input-adaptive multi-exit neural networks, which are designed to reduce carbon footprint by passing images through just one neural layer to see if the necessary threshold to accurately report what the image contains has been achieved.
In a traditional neural network, the image would be passed through every layer before a conclusion is drawn, often making it unsuitable for smart devices or similar technology that requires quick answers using low energy consumption.
The researchers found that by simply adding more complication to images, such as slight background noise, poor lighting, or small objects that obscure the main subject, the input-adaptive model views these images as being more difficult to analyse and assigns more computational resources as a result.
RELATED RESOURCE
The researchers experimented with a scenario whereby hackers had full information about the neural network and found it could be used to max out its energy stores. However, even when the simulation assumed attackers had only limited information about the network, they were still able to slow down processing and increase energy consumption by as much as 80%.
What's more, these attacks transfer well across different types of neural networks, according to the researchers, who also warned that an attack used for one image classification system is enough to disrupt many others.
Get the ITPro daily newsletter
Sign up today and you will receive a free copy of our Future Focus 2025 report - the leading guidance on AI, cybersecurity and other IT challenges as per 700+ senior executives
Professor Tudor Dumitraş, the project's lead researcher, said that more work was needed to understand the extent to which this kind of threat could create damage.
"What's important to me is to bring to people's attention the fact that this is a new threat model, and these kinds of attacks can be done," Dumitraş said.
Bobby Hellard is ITPro's Reviews Editor and has worked on CloudPro and ChannelPro since 2018. In his time at ITPro, Bobby has covered stories for all the major technology companies, such as Apple, Microsoft, Amazon and Facebook, and regularly attends industry-leading events such as AWS Re:Invent and Google Cloud Next.
Bobby mainly covers hardware reviews, but you will also recognize him as the face of many of our video reviews of laptops and smartphones.
-
RSAC Conference 2025: The front line of cyber innovation
ITPro Podcast Ransomware, quantum computing, and an unsurprising focus on AI were highlights of this year's event
-
Anthropic CEO Dario Amodei thinks we're burying our heads in the sand on AI job losses
News With AI set to hit entry-level jobs especially, some industry execs say clear warning signs are being ignored
-
Italy’s ChatGPT ban branded an “overreaction” by experts
News Regulators across Europe will continue to assess the privacy and age concerns of AI models, but Italy's ban may not last
-
Organisations could soon be using generative AI to prevent phishing attacks
News Training an AI to learn a CEO's writing style could prevent the next big cyber attack