What is text mining?
Your business can gain valuable insights by letting AI analytics loose on your emails and documents
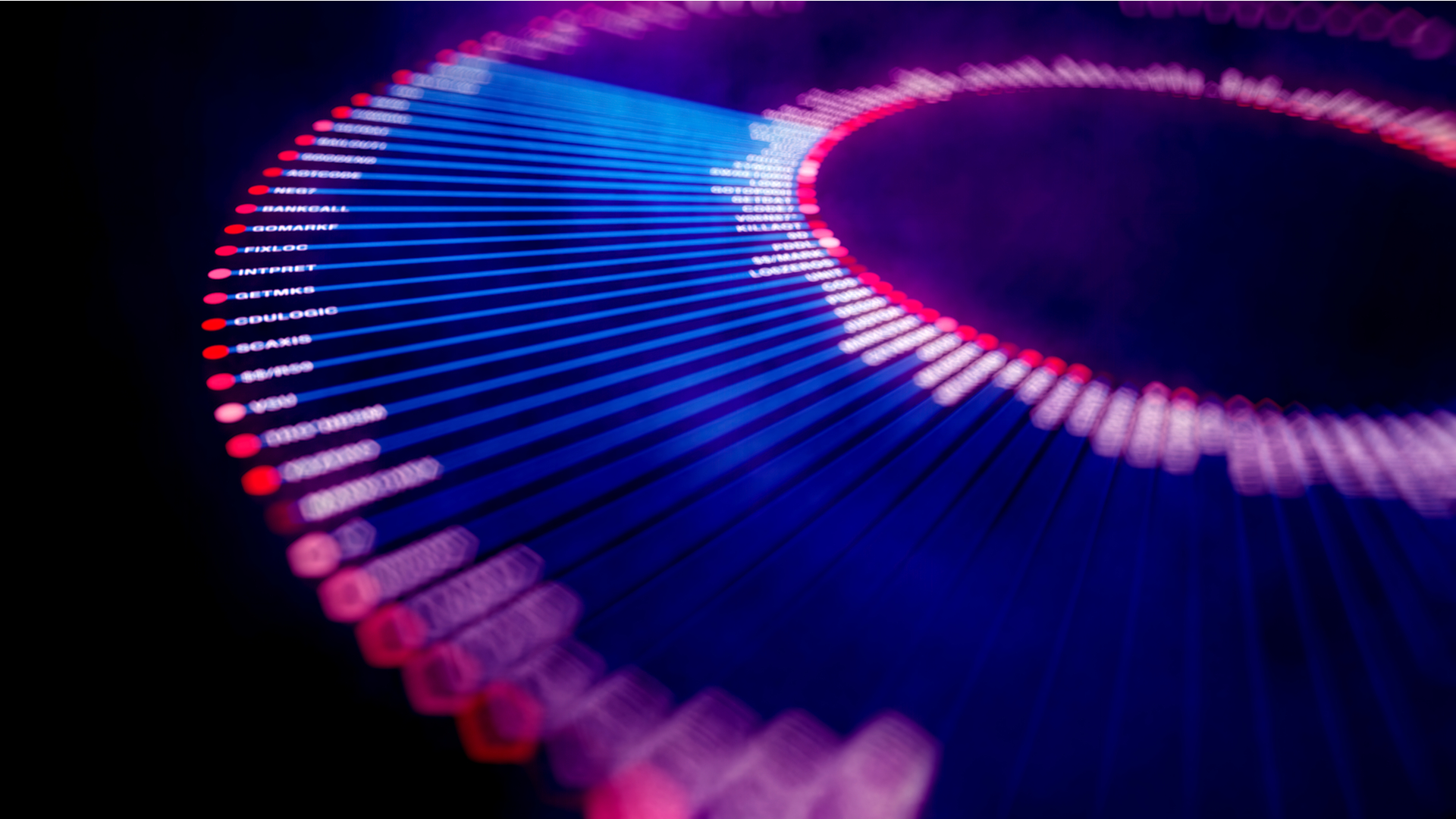
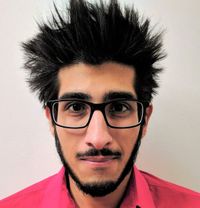
Analytics, the process of deriving information from raw data, is an important practice that businesses have tried to master for as long as such data has been available. In a short space of time, it's evolved from a fairly basic concept to an advanced practice incorporating technologies like machine learning and artificial intelligence (AI).
Text mining is one such evolution, which takes the basic idea of deriving information from data and applying this to vast volumes of documents, letters, emails and written material. As with other conventional analytics, the aim of text mining is to convert raw data into meaningful information which can then be used to support other processes.
Text mining has only really been possible thanks to the advent of AI and more specialist technology like natural language processing (NLP), given that to produce effective results you need to trawl through vast quantities of data at pace. If deployed correctly, text mining has the potential to open new insights for your organisation.
How does text mining work?
Before your organisation can take advantage of text mining, any text-based data needs to be structured – in other words, text mining is a secondary process. For example, data contained in streams of uncategorised documents is considered unstructured.
To give this kind of data structure, businesses often deploy relational databases, where the data is organised based on connections between stored items. This would need to involve a variety of processes, such as parsing of text or pattern analysis, before it is considered structured. Once in this form, the data can be translated to something more visually appealing, such as charts, maps and tables.
Unleashing the power of natural language processing is one key method used to structure raw text-based big data. This technology uses data in combination with algorithms to add context to the way machines try to understand spoken language. It essentially tries to replicate the process by which a human might read text, and often serves to understand and define potentially vague words, like 'bow' for example. It's also embedded in most AI-powered virtual assistants, like Apple's Siri or Microsoft's Alexa.
NLP is deployed as part of this process to churn through reams of documentation in a way that would otherwise be too costly and time-consuming for any human, identifying the most relevant and important nuggets of information, based on any particular request.
Get the ITPro daily newsletter
Sign up today and you will receive a free copy of our Future Focus 2025 report - the leading guidance on AI, cybersecurity and other IT challenges as per 700+ senior executives
One important branch of text mining is sentiment analysis, which involves combing through vast quantities of documentation to summarise how certain groups of people, either customers or employees, feel towards a certain issue. This could be used to learn how customers feel toward a brand, such as using text mining on web forums, or can be used to assess worker morale by subjecting internal emails to analysis.
Relationships, patterns and key facts are isolated and then turned into structured data so that AI can conduct further analysis on the data and identify insights based on what was demanded in the first place.
Benefits of sentiment analysis
Once assorted into a more structured format, the data can then be exposed to algorithms designed to give businesses high-quality insights that were impossible to glean through human-led analysis.
Sentiment analysis is one key application of text mining that can give businesses the exact thoughts and feelings about a company, or a particular aspect of a company. The insights could range from customer attitudes towards a brand to the morale of employees within the organisation.
In the former example, the text absorbed into the text mining process might come from online reviews, social media, customer interactions via email, as well as call centre interactions. These can be turned into data points to identify patterns that point to common threads in the way people perceive a certain brand. The information can then be presented in such a way as to devise strategies to solve negative branding and improve standards and practices.
This form of data analytics can also be applied within an organisation to monitor the way that workers interact with each other through workspace applications like Slack or Microsoft Teams, as well as email. This is so that an organisation can determine how employees are feeling towards the leadership, for instance, and use this information to find ways to boost morale or build trust in areas where it may be lacking.
The Enron effect
A now infamous scandal of the early noughties provides a useful case study for demonstrating the power of text mining. Almost a decade after the bankruptcy of energy firm Enron, a text-mining firm, KeenCorp, has managed to sift through troves of emails dating back to the days of the scandal.
The emails in question held correspondence between 150 of the company’s executives, essentially chronicling the downfall of the company. KeepCorp was able to make sense of the vast trove by passing it through an algorithm tailored to track company morale.
By tracking changes in the tone of the messages, KeepCorp’s algorithm was able to pinpoint the exact date when communications started to turn sour; 28 June 1999. This also turned out to be the date that the company’s board had discussed ‘LJM’, a proposal to hide the company’s struggling finances. This is considered to be one of the key moments of Enron’s downfall.
This is just one example of how text mining is able to make sense of enormous volumes of data that may otherwise obfuscate important information.
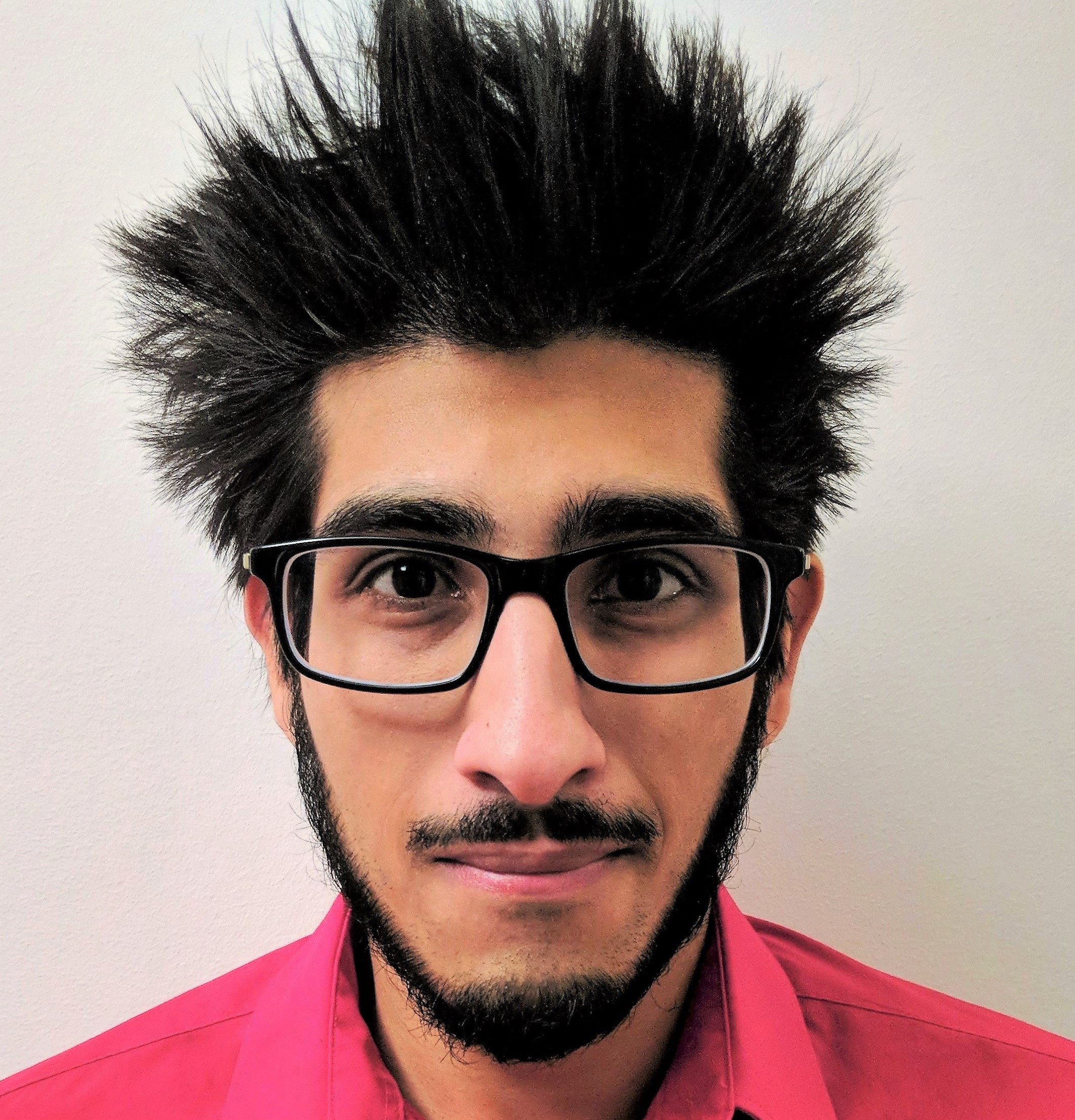
Keumars Afifi-Sabet is a writer and editor that specialises in public sector, cyber security, and cloud computing. He first joined ITPro as a staff writer in April 2018 and eventually became its Features Editor. Although a regular contributor to other tech sites in the past, these days you will find Keumars on LiveScience, where he runs its Technology section.
-
RSAC Conference 2025: The front line of cyber innovation
ITPro Podcast Ransomware, quantum computing, and an unsurprising focus on AI were highlights of this year's event
-
Anthropic CEO Dario Amodei thinks we're burying our heads in the sand on AI job losses
News With AI set to hit entry-level jobs especially, some industry execs say clear warning signs are being ignored
-
Learning and operating Presto
whitepaper Meet your team’s warehouse and lakehouse infrastructure needs
-
Green Quadrant: Enterprise carbon management software 2022
Whitepaper Detailing the 15 most prominent carbon management software vendors to see if they fit your requirements
-
Gartner: Data analytics teams failing to deliver benefits despite rising budgets
News Human-related challenges, such as lack of talent, were highlighted as key impediments to data strategy success
-
insideBIGData: Guide to energy
Whitepaper How big data can help energy companies manage intense disruption
-
Robotic process automation
Whitepaper A no-hype buyer's guide
-
The increasing need for environmental intelligence solutions
Whitepaper How sustainability has become a major business priority and is continuing to grow in importance
-
Four steps to better business decisions
Whitepaper Determining where data can help your business
-
2022 Magic Quadrant for data integration tools
Whitepaper Using research to evaluate suitable vendors for their existing and upcoming data integration use cases