What is data analytics?
A guide to data analytics, the business-critical process of turning raw data into actionable information
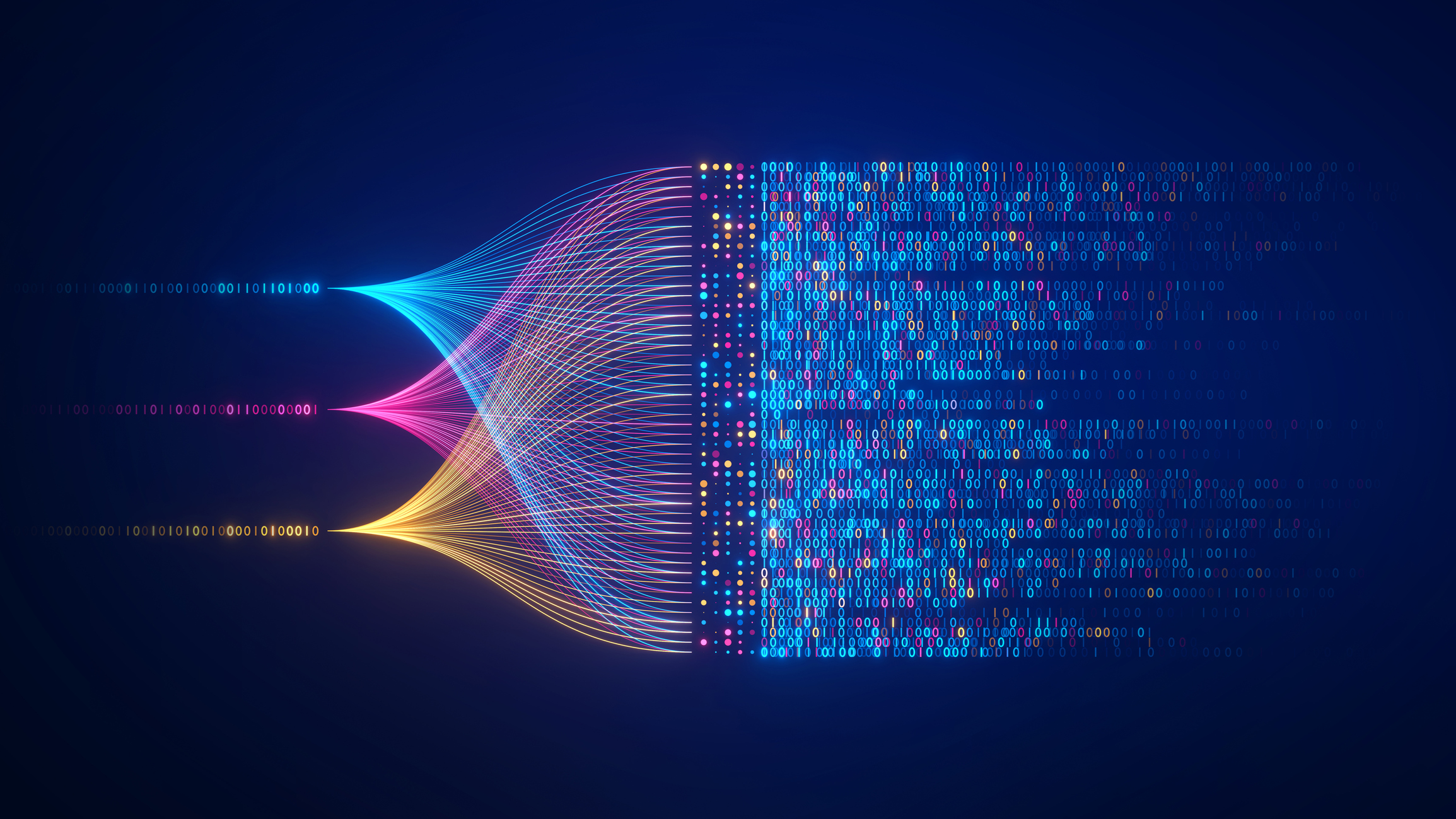
Organizations are becoming more data-driven - based on the recognition that information about products, services, customers, and employees is one of a business’ most valuable assets. As such, data analytics can help firms understand their operations, the market in which they operate, and what makes for well-grounded decisions.
But what is data analytics? There are a few different interpretations and deployments that we will go over below.
In the simplest of terms, it’s the study of data sets to reach conclusions about the information held within. These days, this is often achieved with purpose-built systems. While organizations can use data analytics to make more informed decisions, academics can also use it to study scientific simulations, theories, and hypotheses. One use case could be applying an algorithm to a data set to look for trends that could aid a business decision.
Unlike big data analytics, which starts with high-volume raw data and needs specialist information processing to gain insights, data analytics focuses on what the analyst already knows and what conclusions they can draw from existing data sets.
Data analytics can also refer to the qualitative and quantitative methods and procedures used to improve productivity, identify strengths and weaknesses, streamline internal processes, and cut costs. Data can be extracted and classified to identify and analyze data and patterns, and practices vary according to business requirements.
Changing data enables methodical decision-making. For example, social networking websites can gather data on user preferences and interests and divide them according to criteria, such as age, gender, or location. Thorough analysis can reveal important user and customer patterns and enable the social network's configuration of content, layout, and general strategy.
The term data analytics can also refer to the applications used to process data into actionable information. This can range from business intelligence to reporting and online analytical processing. Data analytics is somewhat comparable to business analytics, although the latter is concerned with business uses, while data analytics has a broader emphasis.
Get the ITPro daily newsletter
Sign up today and you will receive a free copy of our Future Focus 2025 report - the leading guidance on AI, cybersecurity and other IT challenges as per 700+ senior executives
Data analytics techniques
There are various data analytics techniques to consider. The popular ones are:
Descriptive analytics | Aims to summarize and describe what has happened based on historical data |
Diagnostic analytics | Investigates past performance to identify the root causes or determine why something happened |
Predictive analytics | Uses statistical models, machine learning, and data mining techniques to predict future outcomes or behavior based on historical data |
Prescriptive analytics | Builds on predictive analytics by recommending actions to take advantage of the predictions and achieve specific objectives |
Real-time analytics | Enables instantaneous analysis of data streams as they are generated, facilitating immediate decision-making |
Text analytics | Focuses on extracting insights from unstructured textual data |
Big data analytics | Tackles the challenge of analyzing massive volumes of structured and unstructured data from diverse sources |
The decision on which data analytics technique should be used depends upon the context and type of data being analyzed.
Examples of data analytics
Data analytics finds applications across a wide range of domains and industries. Here are some notable examples:
- Business Intelligence: Companies leverage data analytics to gain insights into customer behavior, market trends, sales patterns, and operational efficiencies, enabling data-driven decision-making for optimizing processes, reducing costs, and increasing revenue.
See: Five steps for implementing predictive AI successfully
- Healthcare: Analytics is used to predict disease outbreaks, identify high-risk patients, optimize treatment plans, reduce hospital readmissions, and improve overall patient care. Analyzing electronic health records and genomic data can lead to personalized medicine and better health outcomes.
See: How digital experience management helped an NHS trust improve productivity
- Marketing and Advertising: By analyzing customer data, web analytics, social media sentiment, and purchase histories, companies can segment audiences, personalize marketing campaigns, optimize advertising spend, and enhance customer experiences.
See: The future of AI in advertising and media
- Fraud Detection: Financial institutions and e-commerce platforms use analytics to detect fraudulent transactions, money laundering activities, and cyber threats by identifying anomalies and patterns in large datasets.
See: How Bank of America uses AI to improve customer service and take on competitors
- Supply Chain Optimization: Analytics helps organizations optimize inventory levels, streamline logistics, and minimize transportation costs by analyzing data from various stages of the supply chain.
See: Connecting the supply chain data dots
- Sports Analytics: Teams and leagues analyze player performance data, injury risk factors, and game strategies to gain competitive advantages and make informed decisions about player acquisitions, training regimens, and in-game tactics.
See: How LaLiga championed big data to transform data analytics in sport
Processes and methods of data analytics
Most of the work in data analytics occurs in the collection, preparation, and integration of data. Then there is the development, testing, and revision of analytical models to ensure the production of precise results.
The process starts with data collection, where data scientists ascertain the data they need for a specific analytics application. Data from various sources may be brought together, converted into a common format, and uploaded into an analytics system, such as a data warehouse, Hadoop cluster, or database.
Once the data is ready, any quality problems need to be fixed, as this could affect the veracity of analytics applications. More data preparation work is carried out to organize data for analytics.
Finally, after a model is tested and revised, it is put into production mode with a full data set so that data can address a specific problem.
The future of data analytics
Innovations and prevailing trends, such as the proliferation of IoT, have greatly accelerated the data capture process. However, due to data quality issues and a lack of resources, organizations still struggle to fully utilize data analytics.
Traditionally, the limitations of core legacy IT infrastructure restrained data analytical capabilities. The more fragmented the technology, the less applications and data are able to meld together. As a result, demand is growing for tools that can create seamless links across multiple data sources, enabling a homogenous data strategy.
That's where cloud technology comes in. For example, in many aspects of IT, the cloud is transforming data analytics, enabling tools to draw from a multitude of data sources hosted off-premise. The cloud, however, is of little use if advancements are not made to analytical tools themselves. Fortunately, progress is visible when considering that data analytics initially supported the decision-making process, but tools are now increasingly capable of making better decisions independently. Expect this two-pronged approach to shape development in data analytics for many years to come.
Still, we are only at the beginning of the data age. With so many possibilities, it's difficult to foresee precisely which direction data analytics will steer. Over time, it's likely that tools will develop somewhat paradoxically to solve the data quality issues that are presently restricting their use. And, as with many areas, current professions in the sector may gradually become obsolete.
-
What is polymorphic malware?
Explainer Polymorphic malware constantly changes its code to avoid detection, making it a top cybersecurity threat that demands advanced, behavior-based defenses
-
Outgoing Kaseya CEO teases "this is just the beginning" for the company
Opinion We spoke to Fred Voccola who remains a key figurehead at the firm as it enters its next chapter...
-
Put AI to work for talent management
Whitepaper Change the way we define jobs and the skills required to support business and employee needs
-
More than a number: Your risk score explained
Whitepaper Understanding risk score calculations
-
Four data challenges holding back your video business
whitepaper Data-driven insights are key to making strategic business decisions that chart a winning route
-
Creating a proactive, risk-aware defence in today's dynamic risk environment
Whitepaper Agile risk management starts with a common language
-
How to choose an HR system
Whitepaper What IT leaders need to know
-
Sustainability and TCO: Building a more power-efficient business
Whitepaper Sustainable thinking is good for the planet and society, and your brand
-
What is small data and why is it important?
In-depth Amid a deepening ocean of corporate information and business intelligence, it’s important to keep things manageable with small data
-
Microsoft's stellar cloud performance bolsters growth amid revenue slump
News The tech giant partly blames unstable exchange rates and increased energy costs for the slowdown