How AI is changing patch management
With the number of vulnerabilities surging, patch management is becoming complex and time-consuming
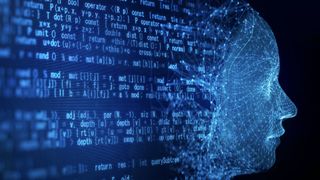
At a time when major tech companies seem to be releasing a constant stream of fixes for already exploited security flaws, patch management and vulnerability management have become a time-consuming and resource-hungry process.
It’s often impossible to keep up with the sheer scale of flaws and zero-days. Businesses need to be able to effectively monitor for available patches, prioritize which are most important, and apply the fix in a timely manner.
Adding to the complexity, some patches can fix one thing and break another, making it integral that they’re tested before they’re applied – especially on mission-critical business applications.
Enter artificial intelligence (AI), which advocates say is already starting to revolutionize the patch management process. In the future, it’s only going to get better, with the ability to predict vulnerabilities before they are even reported. So how is AI changing patch management now, and how will it develop in the future?
The problem with patch management
Patch management is a growing challenge made worse by the sheer number of fixes being issued each week. It’s often difficult to work out which patches need to be applied, how quickly, and where, says Jeff Schwartzentruber, senior machine learning scientist at eSentire. The workload of a proper patch management program is “increasing exponentially” as organizations expand the number of endpoints and variety of software resources they use on a daily basis.
Businesses often end up in a situation where there is too much data to sift through and the information they do have doesn’t offer enough insight, says Chris Goettl, VP of security products at Ivanti. Adding to this, firms need to fix issues without breaking the environment. “These things seem straightforward, but the need to respond to threats faster while trying to reduce the impact on the business requires a lot of time investment,” Goettl points out.
Discover why AI/ML is crucial to cyber security, how it fits in, and its best use cases.
DOWNLOAD FOR FREE
It, therefore, becomes a case of balancing patches to stay secure with maintaining system stability, says Dan Smale, senior service owner, Fasthosts ProActive. “This can be especially daunting for businesses with a large and complex IT infrastructure.”
Get the ITPro. daily newsletter
Receive our latest news, industry updates, featured resources and more. Sign up today to receive our FREE report on AI cyber crime & security - newly updated for 2024.
It is with these challenges in mind that many companies are starting to turn to AI and its subset machine learning to help with patch management. Among the benefits, the technology can be used to detect patches, prioritize them, and apply the fixes quickly when required. The resulting efficiency boost has the potential to enhance overall security. Because the technology allows firms to detect flaws earlier, it reduces the chance vulnerabilities will be exploited in real-life attacks.
How to automate patch management
If there’s lots of data, there is a high probability machine learning can leverage it for operational improvement, says Schwartzentruber. “Machine learning algorithms can understand the complex relationships between competing variables and recommend a patch schedule tailored to the specific organization,” he explains.
At the same time, AI tools can help mitigate compatibility risk by intelligently automating deployment testing and reducing IT resource burden, says Schwartzentruber. Meanwhile, algorithms such as User Entity Behaviour Analytics (UEBA) can identify issues associated with a recent patch change and support automated roll-back if required.
Most vulnerability products ingest from multiple sources and will typically implement an optimization algorithm to prioritize patches, says Schwartzentruber. “In doing so, they give organizations a more holistic view of their patching posture and can provide more tailored and immediate recommendations versus the analysis of a single variable, such as CVSS score.”
AI technology can provide up-to-date and real-time threat reports, allowing security teams to “quickly analyze and act on” issues, says Hasit Trivedi, CTO of digital technologies and global head of AI at consultancy firm Tech Mahindra.
AI-driven patch management solutions are able to assess endpoint and user profiles so only the relevant patches are applied at the right time, says Trivedi. This ensures minimal impact on users and limits overall business disruption.
He adds vulnerability management is the area in which AI is already “adding the most value”. “AI can offer a more proactive approach to risk-based vulnerability management by helping with monitoring and detection of vulnerabilities – even before they are reported,” he says.
Meanwhile, generative AI is being used to create synthetic test data for the patches and can help summarise logs and assist engineers as a “copilot”, Trivedi explains.
The risks in automating patch management
Change is starting to happen, but the use of AI in patch management has only just begun. Today, AI is helping organizations understand and prioritize their environment and patching strategies, says Schwartzentruber. In the future, it will help reduce the time to carry out updates and identify controls for assets that cannot be patched, he says.
Generative AI is changing the way vulnerabilities are being monitored and this capability will improve further, Trivedi says. “Patches are being developed and software is becoming more intelligent to have self-defense and self-patching capabilities, eliminating the need for a centralized patch management process.”
Further down the line, AI will be integrated with self-healing automation to fix impacts caused by patching, says Goettl. “If an update causes an issue, automation will kick in to immediately work to resolve it. Predictive algorithms will be utilized to look ahead and determine what is most likely to be exploited next and result in mitigation or remediation occurring faster before a vulnerability becomes actively exploited.”
The potential is vast, but there are challenges to consider before using AI in the patch management process. The widespread use of any new technology comes with “a steep learning curve”, says Smale. “There’s a need for new skills to understand how, where and when to apply AI for patch management.”
There are also ethical considerations when the technology is being used to make autonomous decisions about patch prioritization and implementation. At the same time, it’s important to accept that AI is not perfect, he says. “Its predictions aren’t always accurate: it may not always correctly predict the impact of patches, which can lead to false positives or negatives.”
There is a balance to be had. Anyone considering the use of AI in patch management should consider their IT infrastructure’s complexity, AI training requirements, and the balance between automation and human oversight, says Smale. “Effective patch management isn’t easy – and organizations need to find a balance between trying to keep up and applying AI too quickly and getting it wrong.”
Kate O'Flaherty is a freelance journalist with well over a decade's experience covering cyber security and privacy for publications including Wired, Forbes, the Guardian, the Observer, Infosecurity Magazine and the Times. Within cyber security and privacy, her specialist areas include critical national infrastructure security, cyber warfare, application security and regulation in the UK and the US amid increasing data collection by big tech firms such as Facebook and Google. You can follow Kate on Twitter.