IT Pro Panel: Does AI have a place in security?
Can machine learning really help keep your organisation safe from cyber criminals?
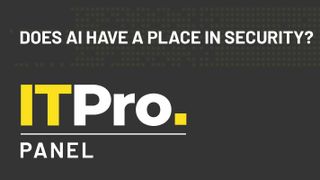
“Machine intelligence is the last invention that humanity will ever need to make.” That’s according to philosopher Nick Bostrom, and it’s arguably true; AI has huge potential to enable and accelerate the efforts of human beings across a wide range of disciplines. It has been predicted that AI may one day diagnose our illnesses, drive our cars, and even prepare our meals.
While that future may still be some years off, AI has found a place in business here and now. Organisations across the globe are deploying specialised AI to handle everyday tasks from data analysis to answering phones, and the capabilities of the technology are being expanded every day.
The IT security space is one industry that has expressed the most interest in advancing this AI. Indeed, the near-constant shortage of talent and resources is often seen as fertile ground for the deployment of AI systems to help plug the gaps in companies’ defences. But does the reality of security-conscious AI live up to the hype? Is it mature enough to deploy within a security organisation? Or is it all smoke and mirrors? Finally, how much work does it take to set up?
FUD fight
The first problem one runs into when discussing this technology from a commercial perspective is that there’s no set definition for what does or does not constitute AI. Although the phrase conjures up images of omniscient, self-aware programs sifting through vast reams of data at lightning speeds, the reality is frequently far less grandiose.
The question of where to draw the line between a system that merely automates certain processes based on a pre-defined set of rules and one which displays genuine learning and intelligence is the subject of much debate. Software vendors have been known to use somewhat liberal definitions of AI, only to be met with scepticism from the security community.
Many security professionals will argue that companies attempt to baffle customers with fancy-sounding jargon which disguises the comparative simplicity of their products’ functionalities, while the firms in question contend that their solutions use machine learning to deliver transformational benefits.
“What is 'genuine AI' anyway?” asks Kantar CISO Paul Watts. “I think that's where the vendors like to play: in the 'grey space' between the terms. I think a few vendors might be passing off rules-based decisioning as AI.”
Get the ITPro. daily newsletter
Receive our latest news, industry updates, featured resources and more. Sign up today to receive our FREE report on AI cyber crime & security - newly updated for 2024.
The most common area in which AI and machine learning systems have been deployed so far is in data analysis, and for Moonpig’s head of cyber security, Tash Norris, this question all comes down to the insights that a system can deliver.
“One of the definitive factors for me is the ability to give insights on 'new' information or behaviour and to be able to perform even with incomplete data sets. There are too many vendors with just a large set of if-else statements talking about having AI!”
“As Tash pointed out,” says William Hill group CISO Killian Faughnan, “I'd challenge whether something is AI if it can't deal with gaps in the data it is receiving, and I suspect that where that happens, we'd find ourselves with a system hammering us with false positives that burn time tracking down.”
Man vs machine
The situation Faughnan describes is the last thing any CISO is looking for when they deploy AI - not least because removing time-consuming manual workloads from security professionals are among the most popular benefits of the technology. Much of a security analyst’s job is spent wading through logs and monitoring dashboards, trying to identify potential vulnerabilities or attack patterns.
This is often a long and tedious task, and Watts notes that one of the reasons it’s frequently given over to AI tools is that “humans find it as dull as hell to do themselves”. Indeed, Norris suggests the use of AI for these tasks may actually boost employee retention rates within organisations’ security operations centre (SOC) by removing one of the least-favoured jobs.
A more technical reason for their deployment to these roles is that current-generation AI is well-suited to pattern analysis, and works best when it’s fed large volumes of data - whereas human operatives are the exact opposite.
“Analysts will naturally look for correlations they've seen before, or that they expect to see,” Norris explains. “A true implementation of AI should be able to draw 'unbiased' correlations, bring more value from the datasets you have.”
“What Tash said,” adds Watts. “Humans looking at the same data over and over again will miss the obvious or will approach analysis in a singular methodical way meaning intricate patterns can be missed. True AI should have no unconscious bias.”
In general, our panellists agree that the most sensible place to deploy AI and machine learning systems is in the broad category of detection and response functions, including tasks like SIEM, SOAR, and EDR. By automating these more manual processes, staff can be freed up to work on more dangerous threats, using AI as a force multiplier to extend the capabilities of a security team.
“Attackers are using machine learning and other AI approaches to improve their attacks,” says LafargeHolcim EMEA CISO José María Labernia, “driven by commoditisation of machine learning software and the massive availability of data.”
“The way to tackle it, to me, is by using AI to improve security defensive tools, investing in machine learning that will increase the power that security analysts have in their day to day activities. Security analysts need to focus on the sophisticated attacks and machine learning can help to keep the focus right.”
Bad bots
Labernia’s point is one that is rapidly gaining traction within the industry; businesses aren’t the only ones who see the benefits of AI, and cyber criminals are starting to leverage it, too. Watts and Norris have both seen machine learning tools used for nefarious purposes, such as using natural language processing to customise the text of phishing emails, or adding wait conditions to malware before it’s executed.
“I worry about ransomware actors starting to leverage AI,” Watts says, “we would be so screwed!”
The threat of AI-enabled hackers is one that the entire security industry is in the process of grappling with, but Norris worries that the issue may push widespread deployment of autonomous AI before it’s ready.
“There is a risk that the ever-growing threats from external attackers will drive complete AI adoption (with no oversight in resulting actions taken by the model) in some areas before enough trust is fully established,” she notes.
For CISOs like Watts, AI still has a long way to go before it can be left to its own devices, and although he sees the benefits for things such as inference and analysis, he says he “wouldn't be comfortable in letting it drive end-to-end”.
“I do think there's a core question of trust when it comes to AI,” Norris says. “Generally, I think governance, regulation and ethics around AI has quite some way to come to help build meaningful trust (especially with vendors) that will allow users to permit little to no oversight.”
Transform and roll-out
While conversations around AI have become widespread within the security community, the actual deployment of the technology is still somewhat varied. Labernia, for example, reports that while LafargeHolcim wants to roll it out, “we simply don't have the time and manpower to invest in it yet.”
Kantar, meanwhile, has jumped in with both feet. Watts notes that the organisation is “very invested” in the use of AI across a number of its own products and services, and says that he wants to use that expertise to build more AI capabilities into his defensive stance.
“From a security perspective, it’s working its way into our detect and respond capabilities and I can see it branching across to other tenets of the cyber security framework very soon. Strategically, I hope we will be able to build bespoke AI capability into our security posture, building on the broader AI experience and knowledge from within our organisation.”
William Hill is also putting AI to work in its threat detection apparatus, and Faughnan reports that he has found success deploying it in areas where there’s a steady stream of data that can be fed to machine learning systems in order to improve their effectiveness.
“The AI opportunities we've found useful thus far are much more in the user behavioural space with regard to internal threats, or other areas where we can ingest enough structured data that it becomes reasonable to expect accurate assessments of risk.”
Data on the activity and status of the organisations’ employees and end-users within the business has been most useful for this purpose, he says, although he adds that the last year has forced him to adapt somewhat.
“The shift away from office-based work meant we lost a lot of the value on some of our more traditional solutions, but thankfully were already delving into approaches to deal with more distributed working patterns.”
“Web application firewalls are also a really great place to capture large data sets that could be used for deep learning,” Norris adds, “especially vendors with huge customer bases. This type of data could be really powerful in terms of being able to better (and more quickly) quantify bad versus good bot traffic.”
“My feeling is that over time we'll find smaller and smaller pieces of functionality that fit together to replace what a WAF should do today,” says Faughnan. “Arguably at that point, you're getting into territory where AI would be valuable; in that it can focus on increasingly smaller pieces of the puzzle and automate a response to a very specific threat.
“I think Tash is right. As we start to leverage the ability to run specific WAF functions where we need them, choosing the pieces we need in a modular fashion, vendors will need to follow the same path. At that point then it becomes sensible that we have AI per function, dealing with the data relevant to it to make decisions.”
Build versus buy
One of the eternal questions in IT is whether to purchase an off-the-shelf solution or invest in building your own custom tool, but when it comes to AI, our panellists are somewhat divided.
“The answer is always ‘buy’ from my end,” says Labernia. “When you buy, others have taken the risk, have invested, have explored and iterated - and you can just benefit by paying. It’s very clean if what you need is available in the market already.”
Similarly, Faughnan generally comes down on the side of purchasing off the peg, on the basis that there’s little value in trying to reinvent the wheel when somebody else has already done most of the legwork for you.
“I buy into it when it comes in a box with a badge on it (theoretically speaking) and requires minimal investment in terms of my teams time,” he says. “I've got some really talented engineers, but building custom AI isn't what they're there for. Integrating someone else's ‘AI’ is a different story though.”
On the other hand, building your own AI tools is easier to justify if your engineering organisation is already working on similar projects - as is the case for both Norris and Watts. Moonpig in particular has invested heavily in AI capabilities to power its recommendations engine, and Norris says that while she’s “almost always going to be ‘team buy’”, organisations shouldn’t discount the possibility of doing it themselves.
“Buy is often a smart choice,” she says, “but I think it's wise not to miss the opportunity build can give to engineers! It's an exciting challenge, and a good skillset to encourage. We have some exceptionally talented engineers in our tech team here at Moonpig and they've been driving the work on AI. I don't know if they'd call themselves AI specialists but I think they're quite super!”
Regardless of whether or not organisations are building their own tools, however, Watts cautions that organisations may have to budget more time than they think when deploying AI systems.
“We’ve seen immediate efficiency benefits but also have seen workloads increase in the short term, as the AIs and humans have learned how to work together,” he says.
There were some initial challenges to overcome, such as managing additional knowledge capital and sorting false inferences from interesting results, but he notes that these issues - as well as the temporary workload increases - have now been smoothed out.
“You just need to recognise that both the AI product and the consumer need to learn and adapt over time to get the usage right; there can be a lot of noise upfront and you need to be able to tune that out. We now know to expect early-life overheads in future implementations of AI and machine learning products.”
To apply to join the IT Pro Panel, please click here to enter your details. Please note that we are not accepting applications from technology vendors at this time.
Adam Shepherd has been a technology journalist since 2015, covering everything from cloud storage and security, to smartphones and servers. Over the course of his career, he’s seen the spread of 5G, the growing ubiquity of wireless devices, and the start of the connected revolution. He’s also been to more trade shows and technology conferences than he cares to count.
Adam is an avid follower of the latest hardware innovations, and he is never happier than when tinkering with complex network configurations, or exploring a new Linux distro. He was also previously a co-host on the ITPro Podcast, where he was often found ranting about his love of strange gadgets, his disdain for Windows Mobile, and everything in between.
You can find Adam tweeting about enterprise technology (or more often bad jokes) @AdamShepherUK.