The human brain is far more complex than AI researchers imagine
Think that the human brain is analogous to a computer? Wake up and read the research
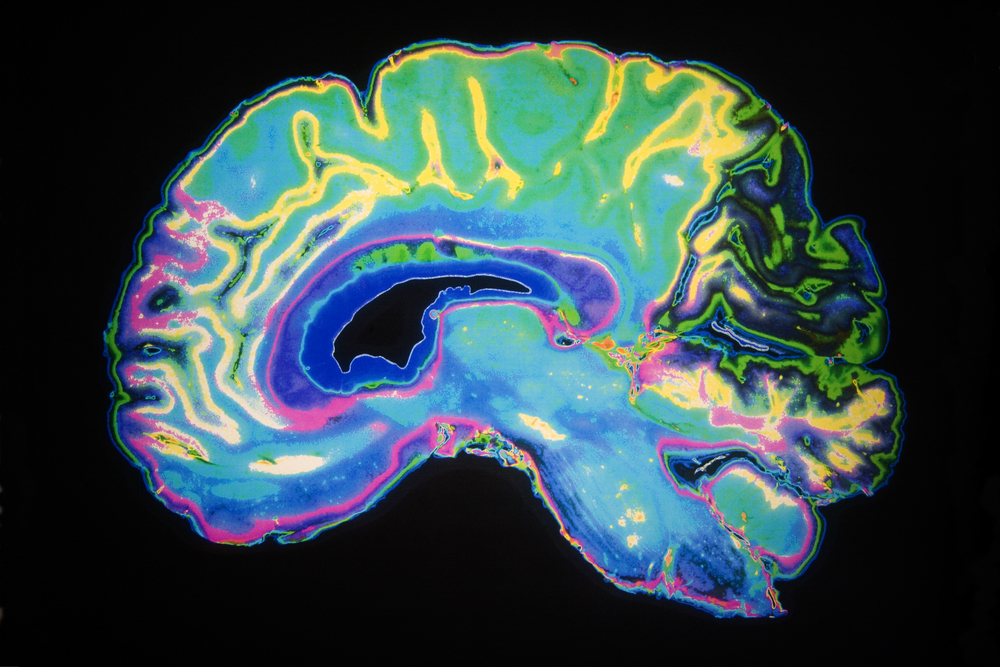
I’ve devoted a lot of space in previous columns to questioning the inflated claims made by the most enthusiastic proponents of artificial intelligence (AI), the latest of which is that we’re on the brink of achieving “artificial general intelligence” on a par with our own organic variety. I’m not against the attempt itself because I’m just as fascinated by technology as any AI researcher. Rather, I’m against a grossly oversimplified view of the complexity of the human brain, and the belief that it’s comparable in any useful sense to a digital computer. There are encouraging signs in recent years that some leading-edge AI people are coming to similar conclusions.
There’s no denying the remarkable results that deep neural networks based on layers of silicon “neurons” are now achieving when trained on vast data sets: the most impressive to me is DeepMind’s cracking of the protein folding problem. However, a group at the Hebrew University of Jerusalem recently performed an experiment in which they trained such a deep net to emulate the activity of a single (simulated) biological neuron, and their astonishing conclusion is that such a single neuron had the same computational complexity as a whole five-to-eight layer network. Forget the idea that neurons are like bits, bytes or words: each one performs the work of a whole network. The complexity of the whole brain suddenly explodes exponentially. To add yet more weight to this argument, another research group has estimated that the information capacity of a single human brain could roughly hold all the data generated in the world over a year.
On the biological front, recent research hints as to how this massive disparity arises. It’s been assumed so far that the structure of the neuron has been largely conserved by evolution for millennia, but this now appears not entirely true. Human neurons are found to have an order of magnitude fewer ion channels (the cell components that permit sodium and potassium ions to trigger nerve action) than other animals, including our closest primate relatives. This, along with extra myelin sheathing, enables our brain architectures to be far more energy efficient, employing longer-range connectivity that both localises and shares processing in ways that avoid excessive global levels of activation.
Another remarkable discovery finds that the ARC gene (which codes for a protein called Activity-Regulated Cytoskeleton-associated protein), known to be present in all nerve synapses, now plays a crucial role in learning and memory formation. So there’s another, previously unsuspected, chemically mediated regulatory and communication network between neurons in addition to the well-known hormonal one. It’s thought that ARC is especially active during infancy when the plastic brain is wiring itself up.
RELATED RESOURCE
The Total Economic Impact™ of IBM Watson Assistant
Cost savings and business benefits enabled by Watson Assistant
In other experiments, scanning rat brains shows that activity occurs throughout most of the brain during the laying down of a single memory, so memory formation isn’t confined to any one area such as the hippocampus. Other work, on learned fear responses, demonstrates that repeated fearful experiences don’t merely lay down bad memories but permanently up-regulate the activity of the whole amygdala to make the creature temperamentally more fearful. In short, imagining the brain (or even the individual neuron) as, say, a business laptop is hopelessly inadequate: rather it’s an internet-of-internets of sensors, signal processors, calculators and motors, capable not only of programming itself, but also of designing and modifying its own architecture on the fly. And, just to rub it in, much of its activity is more like analog than digital computing.
The fatal weakness of digital/silicon deep-learning networks is the gargantuan amount of arithmetic, and hence energy, consumed during training, which, as I’ve mentioned in a recent column, leads some AI chip designers towards hybrid digital/analog architectures. The physical properties of a circuit, like current and resistance, perform the additions and multiplications on data in situ at great speed. However, the real energy hog in deep learning networks is the “back-propagation” algorithm used to teach new examples, which imposes enormous repetitions of the calculatory load.
A more radical line of research is looking outside of electronics altogether, towards other physical media whose properties in effect bypass the need for back propagation. The best known such medium is light: optically encode weights as different frequencies of light and use special crystals to apply these to the video input stream. This could eventually lead to the smarter, faster vision systems required for self-driving cars and robots.
Sign up today and you will receive a free copy of our Future Focus 2025 report - the leading guidance on AI, cybersecurity and other IT challenges as per 700+ senior executives
Another, far more unexpected medium is sound: researchers at Cornell are using vibrating titanium plates that automatically integrate learning examples supplied as sound waves by a process called “equilibrium propagation”. Here, the complex vibration modes of the plate effectively compute the required transforms while avoiding the energy wastage of back propagation.
The ultimate weird analog medium has to be spaghetti, which appeals to the Italian cook in me. This involves sorting the spaghetti (analogous to sequences) by “height”, but see the Wikipedia entry for “Spaghetti sort” for a graphic illustration that will do in ten seconds what it would take me a thousand more words.
-
Helping customers adopt a multi-cloud infrastructure and accelerate their modernization journey
Sponsored Content We outline what shifting to a subscription model means for your business
-
UK firms are 'sleepwalking' into smart building cyber threats
News The convergence of operational technology and IT systems is posing serious risks for property firms.