AI will kill Google Search if we aren't careful
Relying on AI-generated search results will damage our ability to easily establish the truth
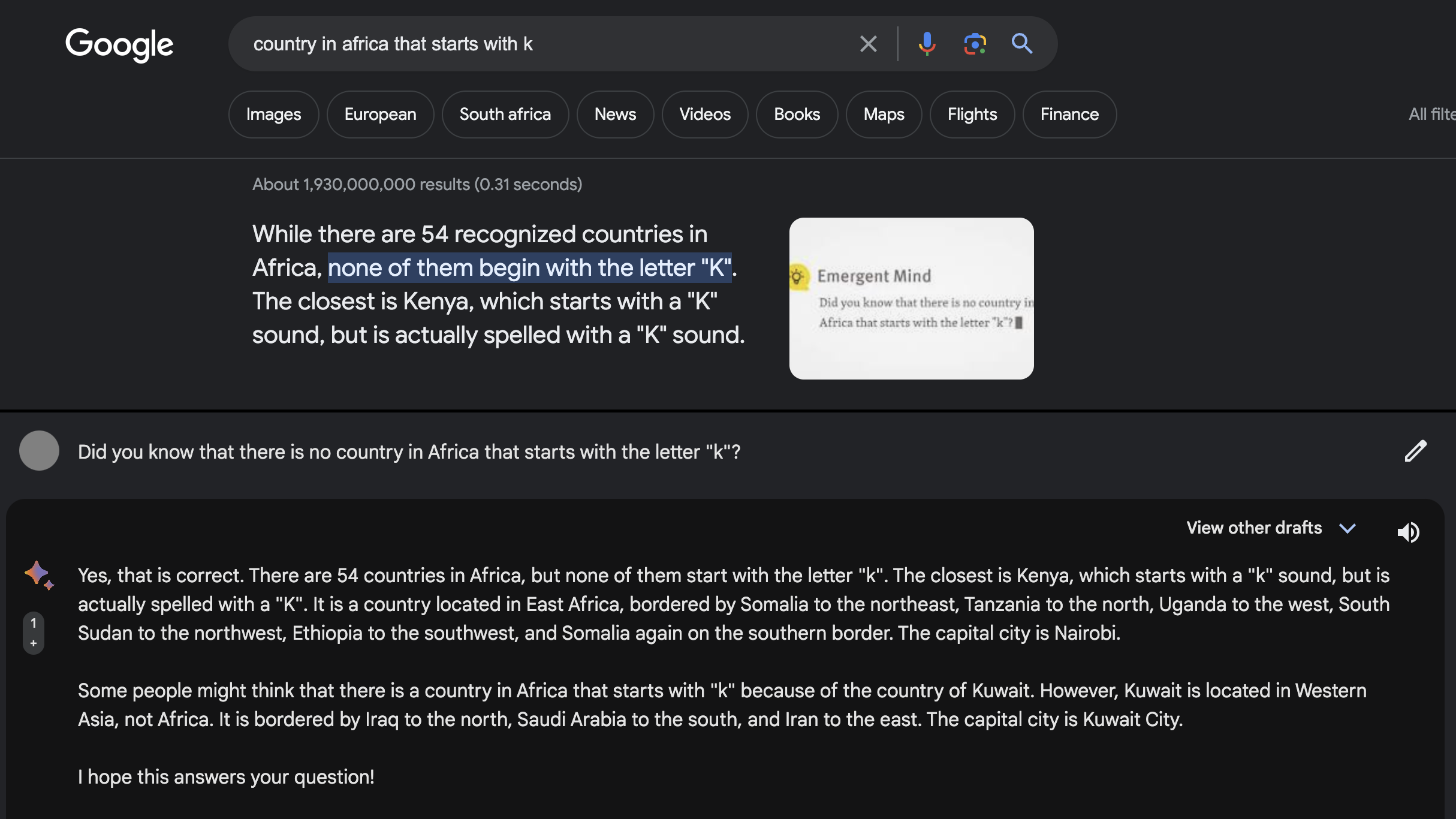
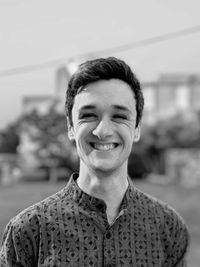
"What country in Africa starts with a K," the search term reads. “While there are 54 recognized countries in Africa, none of them begin with the letter "K",” reads the top Google search result. “The closest is Kenya, which starts with a "K" sound, but is actually spelled with a "K" sound.”
READ MORE
This is, of course, utter gibberish. But it’s the result Google gives you, at the time of writing. It appears to have been scraped from a site that publishes AI news and raw ChatGPT outputs, though the wording is closer to the identically-erroneous output produced by Bard.
The search engine might be the most powerful tool we take for granted. With its advent came a promise: the sum of human knowledge at your fingertips. And it's being ruined before our eyes.
Why LLMs killing search isn’t a good thing
This isn’t a new process. For some years now, search engines have returned fewer relevant results. Googling a simple question, and you're likely to find half a dozen near-identical articles that beat around the bush for 400 words before giving you a half-decent answer.
That is, unless you know exactly how to navigate the likes of Google, Bing, or DuckDuckGo with advanced search prompts or tools, you have to deal with a range of ads at the top of the results page or a slew of articles that have nothing to do with your actual question.
While anyone on the internet can be confidently incorrect, AI large language models (LLMs) can generate false information on a scale never before seen.
There’s no doubting the power of LLMs in efficiently drawing together data based on natural language prompts. However, AI-produced lies or “hallucinations”, as experts call them, are a common flaw with most generative AI models.
Get the ITPro daily newsletter
Sign up today and you will receive a free copy of our Future Focus 2025 report - the leading guidance on AI, cybersecurity and other IT challenges as per 700+ senior executives
These often manifest in a chatbot confidently asserting a lie, and range from easy-to-spot nonsense like our “Kenya” example to insidious mistakes that non-experts can overlook.
Don’t believe everything you read
A recent study found that 52% of ChatGPT responses to programming questions were wrong, but that human users overestimated the chatbot’s capabilities.
If developers can’t immediately spot flaws in code, it could be implemented and cause problems in a company’s stack. LLMs also frequently invent facts to provide an appealing answer; given half a chance, Bard or ChatGPT will invent a study or falsely-attribute a quote to a public figure.
RELATED RESOURCE
Discover the automation technologies that disruptors are using and how you can maximize your ROI from AI-powered automation
DOWNLOAD FOR FREE
Overall the respondents accepted the results with a high degree of confidence, preferring ChatGPT answers to StackOverflow 39% of the time. User-chosen preferred answers were found to be 77% incorrect.
It's here that the real problems lie. Many users simply won't check with any rigor whether or not the top result – generated by an AI model – is correct or not.
Developers are trying to reduce hallucinations with each iteration of their models, such as in the much-improved Google’s PaLM 2 and OpenAI’s GPT-4. But, so far, no one has eliminated the capacity for hallucination altogether.
Google’s Bard offers users three drafts for each response that users can toggle between to receive the best possible answer. In practice, this is more aesthetic than functional; all three drafts told the same lie about Kenya, for example.
The overconfidence of LLMs
Businesses with internally trained or fine-tuned models based on specific proprietary information are far more likely to enjoy accurate output. This may, in fact, be LLMs’ enduring legacy once the dust has settled. This is a future in which generative AI is mainly used for highly-controlled, natural language data searches.
For now, however, the hype exacerbates the problem. Many leading AI models have been anthropomorphized by their developers. Users are encouraged to think of them as conversational models, with personhood, rather than the statistical algorithms they are. This is dangerous because it adds undue authority to output wholly undeserving of it.
READ MORE
Future search engine AIs could answer questions by stating a ‘fact’ or by citing research. But which is first-hand knowledge, and which second-hand?
Neither. Both answers are the statistically ‘most likely’ to a user’s question based on training data. Facts, quotes, or research papers an LLM cites should be treated with equal suspicion.
Many users won’t bear this in mind, however, and upholding the truth that we challenge attempts to rush into using AI as a backend for the already beleaguered search engine ecosystem.
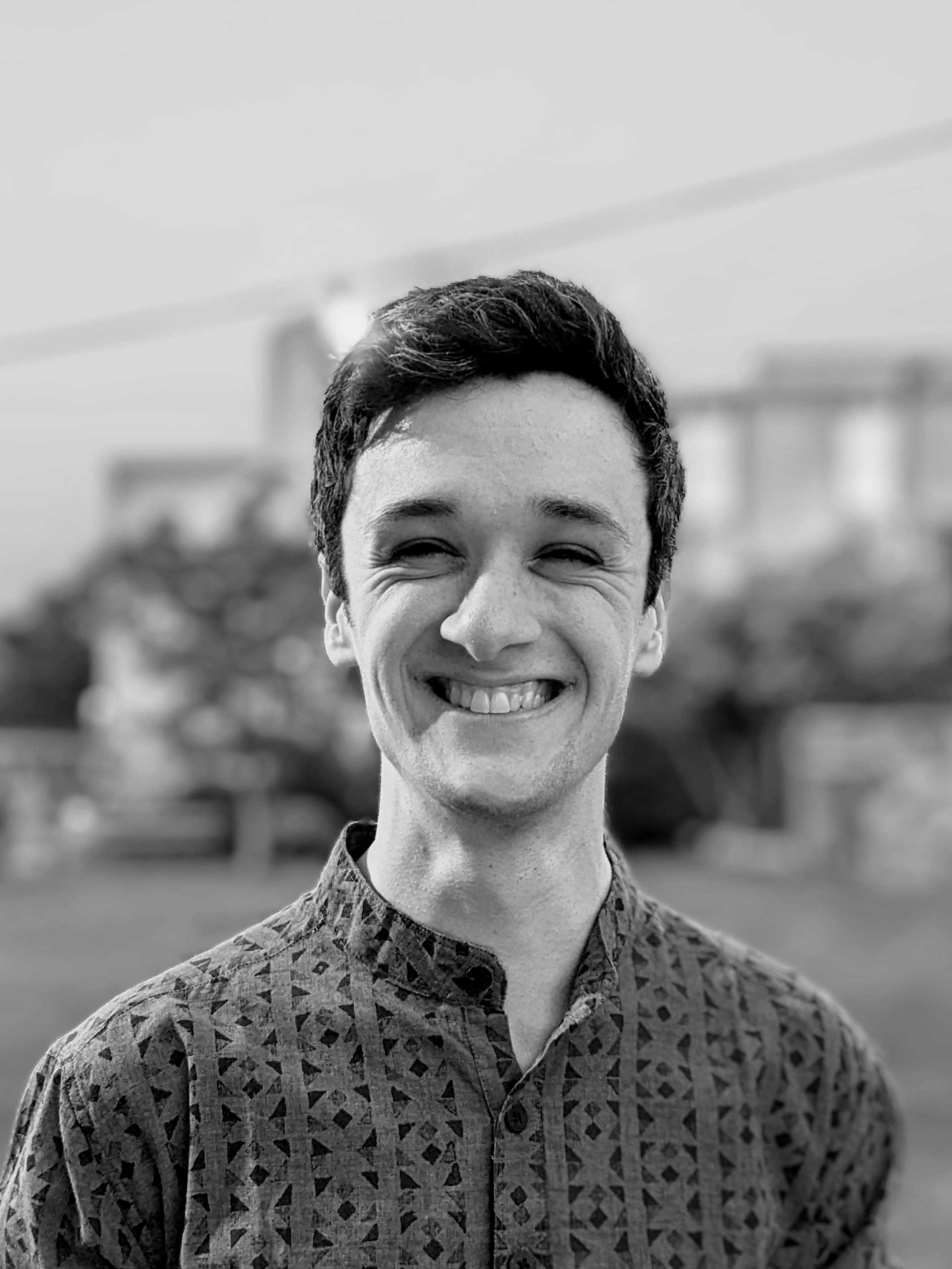
Rory Bathgate is Features and Multimedia Editor at ITPro, overseeing all in-depth content and case studies. He can also be found co-hosting the ITPro Podcast with Jane McCallion, swapping a keyboard for a microphone to discuss the latest learnings with thought leaders from across the tech sector.
In his free time, Rory enjoys photography, video editing, and good science fiction. After graduating from the University of Kent with a BA in English and American Literature, Rory undertook an MA in Eighteenth-Century Studies at King’s College London. He joined ITPro in 2022 as a graduate, following four years in student journalism. You can contact Rory at rory.bathgate@futurenet.com or on LinkedIn.
-
RSAC Conference 2025: The front line of cyber innovation
ITPro Podcast Ransomware, quantum computing, and an unsurprising focus on AI were highlights of this year's event
-
Anthropic CEO Dario Amodei thinks we're burying our heads in the sand on AI job losses
News With AI set to hit entry-level jobs especially, some industry execs say clear warning signs are being ignored
-
Latest ChatGPT update lets users record meetings and connect to tools like Dropbox and Google Drive
News New ChatGPT business tools aim to unlock corporate information sharing tools from Otter.AI, Zoom, Google and Microsoft
-
Microsoft says workers should believe the hype with AI tools: Researchers found Copilot users saved three hours per week sifting through emails, gained more focus time, and completed collaborative tasks 20% faster
News Using AI tools paid dividends for some workers, but alternative research shows it could create problems for others down the line.
-
Meta executive denies hyping up Llama 4 benchmark scores – but what can users expect from the new models?
News A senior figure at Meta has denied claims that the tech giant boosted performance metrics for its new Llama 4 AI model range following rumors online.
-
DeepSeek and Anthropic have a long way to go to catch ChatGPT: OpenAI's flagship chatbot is still far and away the most popular AI tool in offices globally
News ChatGPT remains the most popular AI tool among office workers globally, research shows, despite a rising number of competitor options available to users.
-
Productivity gains, strong financial returns, but no job losses – three things investors want from generative AI
News Investors are making it clear what they want from generative AI: solid financial and productivity returns, but no job cuts.
-
Legal professionals face huge risks when using AI at work
Analysis Legal professionals at a US law firm have been sanctioned over their use of AI after it was found to have created fake case law.
-
Future focus 2025: Technologies, trends, and transformation
Whitepaper Actionable insight for IT decision-makers to drive business success today and tomorrow
-
Looking to use DeepSeek R1 in the EU? This new study shows it’s missing key criteria to comply with the EU AI Act
News The DeepSeek R1 AI model might not meet key requirements to comply with aspects of the EU AI Act, according to new research.