What is retrieval augmented generation (RAG)?
In a bid to make AI even more accurate, retrieval augmented generation (RAG) is growing in popularity and purpose to help large language models (LLMs) tap into real-time and up-to-date sources of information
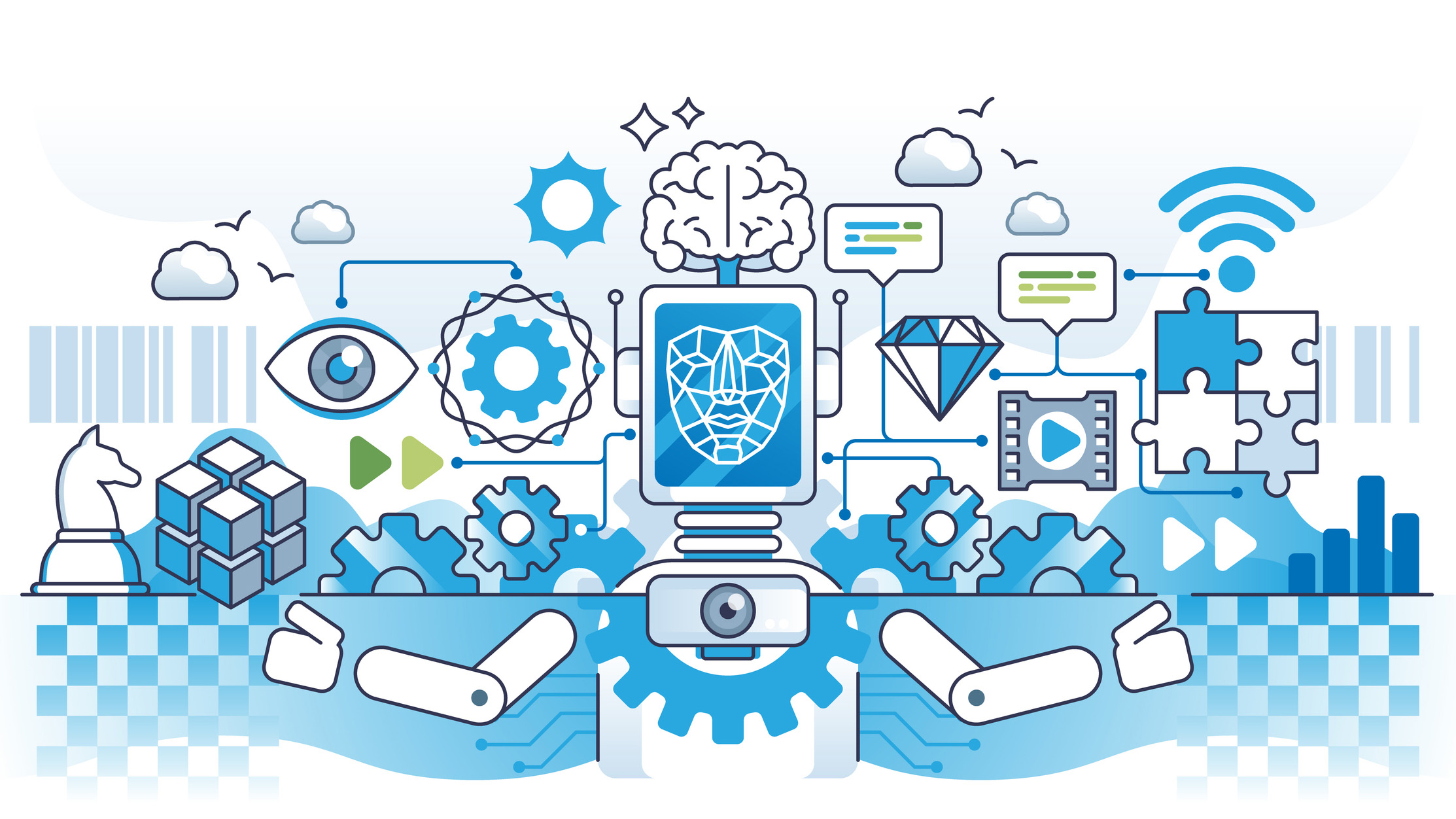
If AI is to become ever more ‘intelligent’ and provide useful answers, it must have greater amounts of contextual information to hand when answering users’ questions.
That’s where new approaches such as retrieval augmented generation (RAG) are now coming to the fore, integrating into large language models (LLMs) – of which OpenAI’s ChatGPT is now one of the most popular and well-known.
Ellen Brandenberger, senior director of product innovation at Stack Overflow, explains: “Generative AI technologies are powerful, but they’re limited by what they ‘know’, or the data they have been trained on.
“RAG is a strategy that pairs information retrieval with a set of carefully designed system prompts that enable LLMs to provide relevant, contextual, and up-to-date information from an external source.”
Examples of RAG being used behind the scenes include people’s interactions with chatbots that know about recent events, are aware of user-specific information, or have a deeper understanding of specific subjects. This is achieved by linking it to sources including internal knowledge bases, science publications from top journals, or other authoritative documents.
Brandenberger adds RAG is particularly adept at improving the accuracy of responses related to domain-specific knowledge, such as industry acronyms.
“LLM users can use RAG to generate content from trusted, proprietary sources, giving them the ability to quickly and repeatedly generate text that is up-to-date and relevant. An example could be prompting your LLM to write good quality C# code by feeding it a specific example from your personal code base.
Get the ITPro daily newsletter
Sign up today and you will receive a free copy of our Future Focus 2025 report - the leading guidance on AI, cybersecurity and other IT challenges as per 700+ senior executives
“RAG also reduces risk by grounding an LLM’s response in trusted facts that the user specifically identifies,” Brandenberger says.
However, she warns developers should always test results from their RAG systems against sample queries and then assess these with human reviews and other forms of LLM evaluation. Taking this approach produces a more accurate and consistent LLM model, she believes.
RAG: Greater return on investment
With so many different AI tools currently on the market, Ryan Carr, chief technology officer (CTO) and VP of engineering at Enveil, believes RAG stands out because of its ability to deliver “real, business-enabling value”.
He explains answers from LLMs alone aren’t always trustworthy, producing so-called “hallucinations”; what he describes as “confident but incorrect responses”. This means it becomes “risky” for businesses to base critical decisions entirely on AI-driven outcomes.
“RAG solves this problem by taking the user’s question or prompt, performing a semantic search for related ground-truth documents, and then feeding these documents to the LLM along with the user’s prompt,” Carr says.
“This allows the LLM to ‘cite its sources’ in the response, allowing users to verify and validate the LLM’s answer.”
But while RAG is still very much in its R&D phase for many, experts see huge promise ahead. It is reported how the term was coined by researcher Patrick Lewis and his team, although he is said to have admitted the acronym wasn’t as nice sounding as they’d hoped for. Another researcher, Douwe Keila, also authored a well-received early paper about RAG.
Veera Siivonen, CCO and co-founder at AI governance company Saidot, is another who sees RAG’s potential while warning “it doesn’t guarantee total immunity from hallucinations”.
However, Siivonen suggests RAG is a good mechanism through which companies can reduce the likelihood of hallucinations and make results easier to fact-check.
But she advises: “A RAG solution should not be implemented in areas where mistakes would be critical unless there is human oversight to filter out bad responses. In addition, RAG should not be trusted as the only risk mitigation strategy, especially if we are discussing a highly delicate customer interface.
“Best practices might include filtering the questions that can be asked, providing transparency on what the bot is designed to answer, and filtering outputs for safety. Finally, it’s always advisable to use generative AI internally before deploying it in customer interfaces.
“This means you can learn its handicaps quickly. Therefore, before releasing the product to customers, I would first provide the tool to help customer agents, and then get their feedback to understand shortcomings and carry out improvements if needed.”
RAG: A bridge for knowledge
RAG’s ability to bridge generative capabilities and access to external knowledge is a major step forward. It has been likened to taking an exam with the materials you need to answer questions alongside you in the exam room to consult – rather than having to memorize the knowledge in advance.
This is because it can quickly interpret breaking news, read through the latest policy documents, or note changes in large amounts of complex information.
RAG offers many potential use cases, especially within regulated industries such as finance (verifying data and offering investment insights) and healthcare, where it can be used to analyze complex medical information and help doctors and patients make or take decisions based on accurate evidence.
Rohan Whitehead, data training specialist at a not-for-profit body the Institute of Analytics, explains how combining the “strengths of retrieval-based and generative models” through RAG ensures outputs are grounded in factual data. This, he says, is delivered thanks to the access the AI has to vast amounts of data in real-time.
“This approach mitigates the risk of generating incorrect or fabricated information, as the generative model is anchored in the retrieved data, which acts as a factual reference point,” he tells ITPro.
“Initially, AI models relied heavily on either retrieval-based methods, which fetched existing information, or generative methods, which created new content based on training data.
“RAG emerged by combining these approaches, leveraging the precision of retrieval systems with the creativity of generative models. Companies like OpenAI and Google have been instrumental in developing and refining RAG techniques to enhance the accuracy and reliability of AI systems.”
With LLM as the front-end backed by RAG, there is also far less time needed for the AI to be trained. Peter van der Putten, director of AI Lab at Pegasystems and assistant professor of AI at Leiden University, explains: “RAG can prove to be a powerful technique that ensures content is always up-to-date.
“Users no longer need to retrain or fine-tune a model every time there’s new content, which would be very costly. Or more importantly, there is a simple solution if some information is no longer valid: just remove the document from the corpus. In contrast, there is no such thing as ‘untraining’ a model.”
Looking ahead, he is hopeful for the technology’s future, suggesting enterprises should now be asking themselves an important question if they are to leverage its full capabilities: “How can new RAGs easily be built and maintained, without requiring data science, AI or engineering support?”
He also cites security as an important factor for companies to consider, for example, who has access to a specific RAG and ensuring RAG’s answers are only based on the specific documents to which this person is allowed to have access. Additionally, there is a critical need to safely log all requests for audit, reporting, and content improvement purposes while filtering sensitive data from a question.
RELATED WHITEPAPER
While the “core idea of RAG is simple” according to van der Putten, it offers generative AI “a better direction and sharper purpose”.
He explains how research areas such as natural language processing (NLP), information retrieval, and semantic search have been studied since the early days of AI but with RAG now propelling the technology forward, van der Putten adds: “What drives adoption for enterprises is not the core smart idea, but the embedding into their architecture and organization”.
Jonathan Weinberg is a freelance journalist and writer who specialises in technology and business, with a particular interest in the social and economic impact on the future of work and wider society. His passion is for telling stories that show how technology and digital improves our lives for the better, while keeping one eye on the emerging security and privacy dangers. A former national newspaper technology, gadgets and gaming editor for a decade, Jonathan has been bylined in national, consumer and trade publications across print and online, in the UK and the US.