The pros and cons of chatbots for customer service
As IT leaders evaluate the risks and rewards of chatbots in customer service, user experience will have to be balanced against potential productivity gains
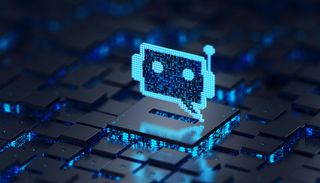
As generative AI has enabled more detailed, context-driven conversations in natural language, firms quickly put the technology to use as the backbone for next-generation chatbots for customer service.
It’s clear there’s much to gain here, with chatbots increasingly able to cut through the noise of repeat customer questions and refer more complex queries to human operators at call centers, who can diagnose the problem in more detail.
While the upside of chatbots can produce savings in cost and boost team efficiency, the downsides may have negative implications for brands that would take equal amounts of funds and team efforts to reverse.
Rogue chatbots could be damaging for a company’s image, or even produce malicious outputs such as malware. There is also the issue of data sanitization for chatbot inputs, Chatbots remain a popular tool for customer service and are only becoming more embedded in the apps and services of businesses as time goes on.
Using chatbots for productivity
Chatbots driven by large language model (LLM can be very effective if they draw from small, specialized data sets. If grounded in the right enterprise data, chatbots can help businesses improve their productivity.
“We started using [chatbots in customer service] in May 2023 and our average customer satisfaction score (CSAT) went from about a 91% average to a 95% average,” Christian Sokolowski, VP of Customer Support at Rebuy tells ITPro.
“Productivity gains for my team include minimizing repetitive inquiries initially,” he continues,. “… eliminating repetitive questions enables them to allocate more time to focus on technical tasks.”
Get the ITPro. daily newsletter
Receive our latest news, industry updates, featured resources and more. Sign up today to receive our FREE report on AI cyber crime & security - newly updated for 2024.
Sokolowski’s team efficiency has risen from 52% to 62% on average. The freeing of his team from repetitive tasks comes as a relief since they have high technical skills and are better focused on critical product solutions.
For many customer service teams, repetitive tasks emerge from returns and cancellations primarily, according to Gartner’s latest research on customer service chatbots.
In Gartner’s figures, complexity was found to greatly affect chatbot resolution rates. For example, the chatbot resolution rate for billing disputes was just 17% whereas routine, highly predictable interactions involving returns or purchase queries questions enjoyed rates of 58 and 52% respectively.
Given the sheer volume of support requests, even an incremental reduction in per-transaction costs can make a difference to a firm’s bottom line.
Cost-cutting measures of chatbots
While analyst predictions vary, it’s clear that chatbots can have a profound impact on the outgoings at firms that implement them. As well as cutting salary cost for workers such as those in call centers, chatbots can improve the efficiency of a company’s sales workflows or improve the user experience to lock in more loyal customers.
Sokolowski reveals how these ideas translate into real-world results. He implemented Rebuy’s chatbot strategy in May 2023, which has since driven cost reductions in the form of repetitive action automation.
“With the additional time and resources freed up, we have been able to expand our scope of responsibilities to include troubleshooting bugs and resolving issues at a product level, collaborating more closely with our development team,” shared Sokolowski.
He also refutes fears over AI threatening the jobs of some workers:
“Consequently, while cost savings have been achieved, job losses have not been the outcome; rather, we have focused on cultivating new, more substantial roles that offer greater meaning and opportunity for professional growth.”
Drawbacks of chatbots for customer service
Before getting stellar results, IT leaders have to carefully prepare their AI threat strategy. Enterprises fall into two categories with chatbots: ones that feed chatbots with broad data and use the most effective proprietary generative AI platforms as backing – usually via application programming interface (API) – and those who upload their knowledge base and leverage open source AI to do the rest.
The results of each will depend on the quality of the data used to train the model, as well as the private data the firm looking to implement the chatbot is able to leverage for its fine tuning.
A major risk of connecting generative AI chatbots directly to customers is exposure to hallucinations, the term used for confidently incorrect claims made by generative AI models. This could give customers false information about a product and hallucinations can also be invoked by users through prompt engineering for malicious uses.
For example, a Chevrolet dealership in the US that had implemented a chatbot based linked to ChatGPT discovered that customers had manipulated the chatbot into agreeing to sell a car for just one dollar. The chatbot even ended the exchange with “and that’s a legally binding offer - no takesies backsies”, as prompted by the user.
Aside from misinformation, this can also lead to reputational damage. As AI developers adopt new training methods to reduce hallucinations and firms such as Google Cloud offer AI models that are ‘grounded’ in enterprise data to offer more relevant and accurate outputs, the risk of poor quality exchanges could go down. At the very least, those looking to adopt generative AI chatbots for customer service need to be aware that there is a possibility of interactions going wrong.
Data leaks
It’s now easier than ever to create a custom chatbot. With this ease comes an expansion of an enterprise’s attack surface. As AI gains access to more data throughout a firm, leaders will need to revisit their data protection policies and procedures to ensure they apply to how they use AI.
There is the potential for customers or employees to upload sensitive information such as salaries, job descriptions, and other sensitive data alongside information that is relevant to customers. These concerns intersect with the issue of shadow AI, in which leaders lose track of AI usage and the flow of data into LLMs within their business.
Researchers at Northwestern University pinpointed the specific risks associated with custom GPTs, OpenAI’s solution for custom-built chatbots. The researchers identified a three-step process bad actors engage to pull files from custom GPTs:
- Scan the Custom GPT store.
- Inject specific prompts.
- Extract sensitive data.
Success rates in extracting sensitive data from 216 custom GPTs ranged between 97 and 100%, following three attempts to breach each GPT.
Using chatbots for malware
In some cases, hackers could use chatbots to deliver malware packages. The theoretical exploit relies on firms using chatbots for internal AI code assistance and would see attackers put malware online under commonly hallucinated package names, in a hands-free attack method that would see victims passively download infected packages over time.
However, at present there is no evidence that chatbots pose this direct risk and claims that AI could be used to assist hackers have been overstated.
Novel attack methods that make use of prompt engineering are also being caught by schemes such as Microsoft’s Bing AI bug bounty program, in which white hat hackers are offers between $2,000 to $15,000 in return for proof of flaws in the search engine’s AI chatbot.
Value of the human touch in chatbot customer service
The unpredictability of human behavior and needs call for human intervention in chatbot customer service. For this reason, successful use of chatbots for customer service often takes the form of a triage system, in which requests below a baseline difficulty are resolved by chatbots and those that the chatbots cannot diagnose or remedy are passed onto human operators.
Humans have agency, to improvise and to expand the list of options available to them or simply expand the boundaries of pre-existing options. Chatbots, no matter the size of the data sets in their language models, hit interaction limits that they cannot surpass due to the limit of their available data or the complexity of user requests.
Put another way: “[T]he difference between a mechanical act and an authentically human one is that the latter terminates at a node whose decisive parameter is not “Because you told me to,” but “Because I chose to,” wrote Joseph Weizenbaum, former MIT professor and the inventor of ELIZA which is often considered the first chatbot, in Chapter 10 of Computer Power and Human Reason, published in 1976.
This is something leaders will have to bear in mind as they push chatbots in front of customers. To maintain their reputations and ensure apparent productivity gains do not come at the expense of digital experience, chatbots will need to be deployed strategically.
“[O]rganizations can address [lack of empathy in chatbots] by restricting the use of chatbots to specific workflows where sensitive situations are less likely to occur,” Sokolowski told us.
“For example, avoiding chatbot deployment in monetary transactions ensures that delicate situations requiring emotional intelligence and empathy are handled by human representatives, thus preserving the quality of customer interactions.”
Lisa D Sparks is an experienced editor and marketing professional with a background in journalism, content marketing, strategic development, project management, and process automation. She writes about semiconductors, data centers, and digital infrastructure for tech publications and is also the founder and editor of Digital Infrastructure News and Trends (DINT) a weekday newsletter at the intersection of tech, race, and gender.