Cloud can help you understand data – but only if you plan first
Moving to the cloud is often seen as a way to get insights from your data, but you need to plan and spend some quality time with it first
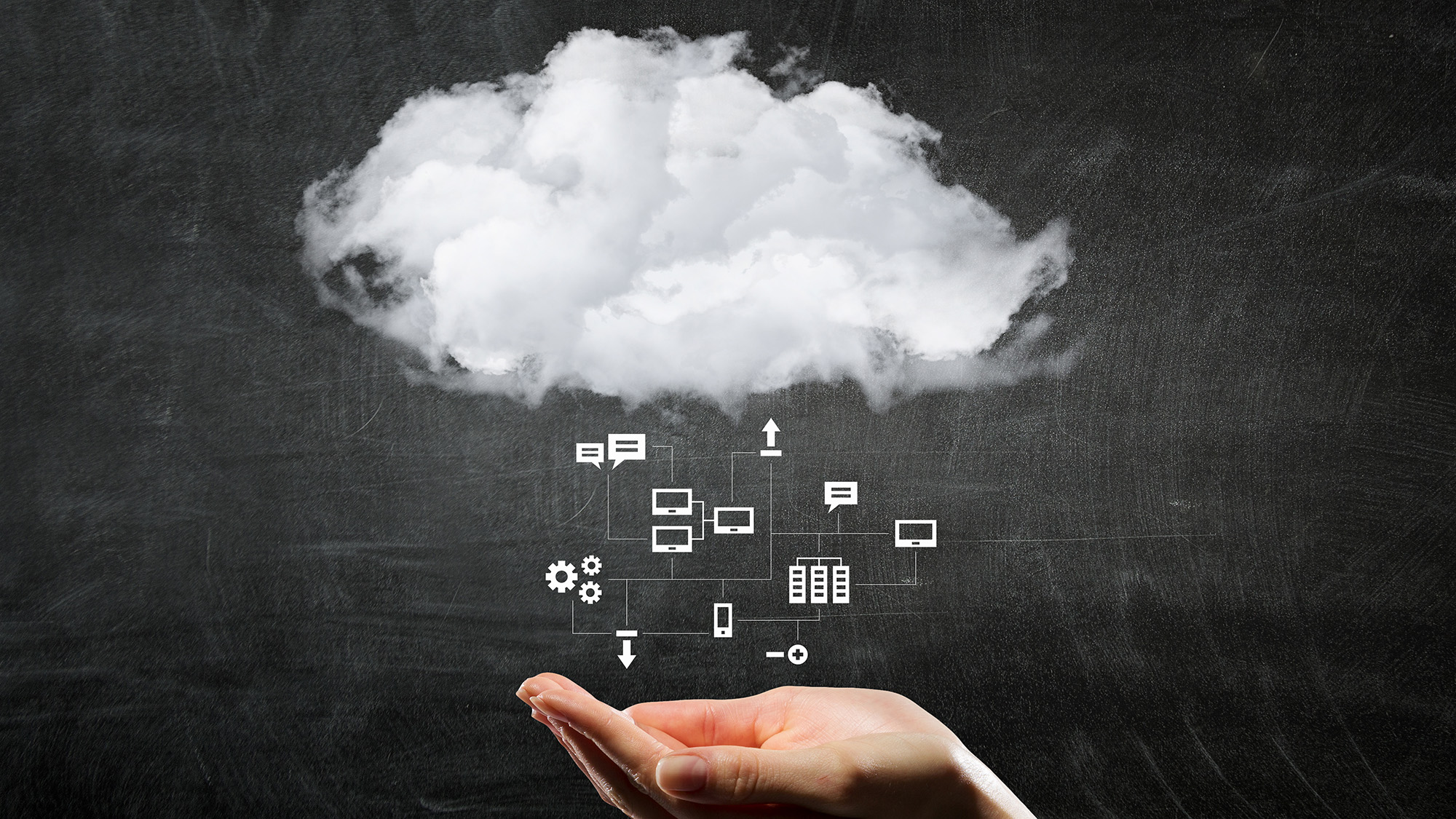
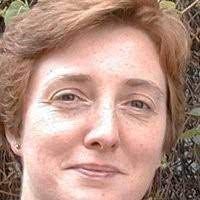
Organisations might be drawn to cloud by the lure of gaining new information and insights from the data they hold. Perhaps they will learn how to retain customers more successfully, or get a better understanding of profit centres – or cost centres. The twin powers of artificial intelligence and machine learning (AI and ML), deployed in the cloud can work wonders. But they need their ‘food’, the raw data they work with, to be in tip top condition, and for many organisations preparing that food requires time, effort, and attention to detail.
Data strategies and clear priorities
There is no doubt that AI and ML can provide information that organisations can’t come by in any other way. Data-crunching can, if done well, help organisations boost productivity and profits. But optimal success doesn’t come overnight. As Yann Lepant, MD for Accenture Technology in the UK tells IT Pro: “It’s easy to get tempted by the extensive set of cloud based new data and AI technologies as it’s an exciting new playground to venture on. However, it’s also a place where it’s easy to consume wastefully and get lost in delivery.”
A key factor in avoiding the pitfalls is to have clear priorities and a solid data strategy. Lepant tells IT Pro that having these will mean “each initiative is done with purpose and outcome, contributing to a journey of maturation through the cloud”.
Ingrid Verschuren, head of data strategy at Dow Jones, whose global news database Factiva grows by a million articles every day, from approximately 33,000 sources, tells IT Pro: “It doesn’t matter how good the technology is if the data feeding it is poor quality. The first and most important step to get the most out of your data is to ensure you are using the right data – and that it is structured in a way that will answer the questions you want to ask.”
So what do clear priorities look like? Paul Clough, professor of search and analytics at the University of Sheffield tells IT Pro: “Identifying the use cases is critical and needs to be based on issues and problems identified in the business by business stakeholders, rather than by data scientists or the IT department.” This approach keeps the priorities very practically focused, he says.
Cleaning and refining
Another pair of related tasks that may need to be undertaken before AI and ML can work their magic are cleaning up old data and refining what new data is collected.
Part of the cleaning task will involve bringing data out of silos. This can be tricky, but worth the effort and organisations undertaking the task can take some comfort from the fact that they’re not alone. Often, data silos are a result of historical practice and how things have grown organically over time, and it takes a concerted effort to undo in a relatively short time what’s built up over a much longer period. But as Lepant points out, there are really no short-cuts. “From getting a 360 degree view of the customer to end-to-end supply chain management, via fraud prevention and intelligent forecasting, the list of business outcomes enabled by the removal of data silos is endless,” he says.
Sign up today and you will receive a free copy of our Future Focus 2025 report - the leading guidance on AI, cybersecurity and other IT challenges as per 700+ senior executives
RELATED RESOURCE
Cleaning data is another task that can take time, but the effort is definitely worth it in the longer term. Verschuren provides some useful advice to help keep the cleaning task focussed, telling IT Pro: “Before you start cleaning your data, you need to determine how that data will be used and what insights you need to generate. Ask yourself, what does perfect look like? From there, you need to define the data fields that will be part of your data set, and the input for each of those fields.” Effectively, work backwards from the outcomes you want to get, and determine what data you need to get them.
And finally, along come analytics and insights
With clean data, a clear data strategy in place, and perhaps some new data collection streams in place too, an organisation is finally in a position to start using AI and ML to gain those valuable insights it craves.
But that’s not quite the end of the story. To continue to gain insights over time, data should take its place, unsiloed, front and centre. Or, as Lepant tells IT Pro when sharing key advice, organisations should “implement a programme to update the ways of working, culture and data literacy to help the business become more data driven and self-served”.
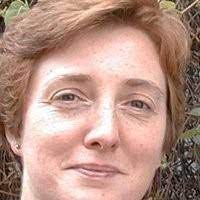
Sandra Vogel is a freelance journalist with decades of experience in long-form and explainer content, research papers, case studies, white papers, blogs, books, and hardware reviews. She has contributed to ZDNet, national newspapers and many of the best known technology web sites.
At ITPro, Sandra has contributed articles on artificial intelligence (AI), measures that can be taken to cope with inflation, the telecoms industry, risk management, and C-suite strategies. In the past, Sandra also contributed handset reviews for ITPro and has written for the brand for more than 13 years in total.
-
Data center water consumption is out of control, but cloud providers want EU lawmakers to go easy on them
News The European Commission says water shortages are being exacerbated by leaks and pollution, but also points to high usage from data center operators.
-
British IT worker jailed for revenge attack on employer that caused a “ripple effect of disruption” for colleagues and customers
News West Yorkshire man Mohammed Umar Taj was suspended from his job in Huddersfield in July 2022, and began taking revenge within hours.
-
Learning and operating Presto
whitepaper Meet your team’s warehouse and lakehouse infrastructure needs
-
Four ways AI is helping knowledge workers excel
Case Study From medical diagnostics to mining and exploration, many industries are using AI to make their workers more effective
-
How to help IT manage itself with autonomous operations
Whitepaper Using AI and automation to proactively adapt to business disruptions
-
Green Quadrant: Enterprise carbon management software 2022
Whitepaper Detailing the 15 most prominent carbon management software vendors to see if they fit your requirements
-
insideBIGData: Guide to energy
Whitepaper How big data can help energy companies manage intense disruption
-
Machine learning vs statistics: What’s the difference?
In-depth Both machine learning and statistics involve collecting datasets, building models and making predictions, but they differ in approach
-
Magic quadrant for master data management solutions
Whitepaper Informing your MDM solution choices
-
Kyndryl partners with Teradata to boost AI and data modernisation
News The new service combines Kyndryl’s data and AI expertise with Teradata’s cloud analytics platform to help customers migrate data to the cloud