Machine learning vs statistics: What’s the difference?
Both machine learning and statistics involve collecting datasets, building models and making predictions, but they differ in approach
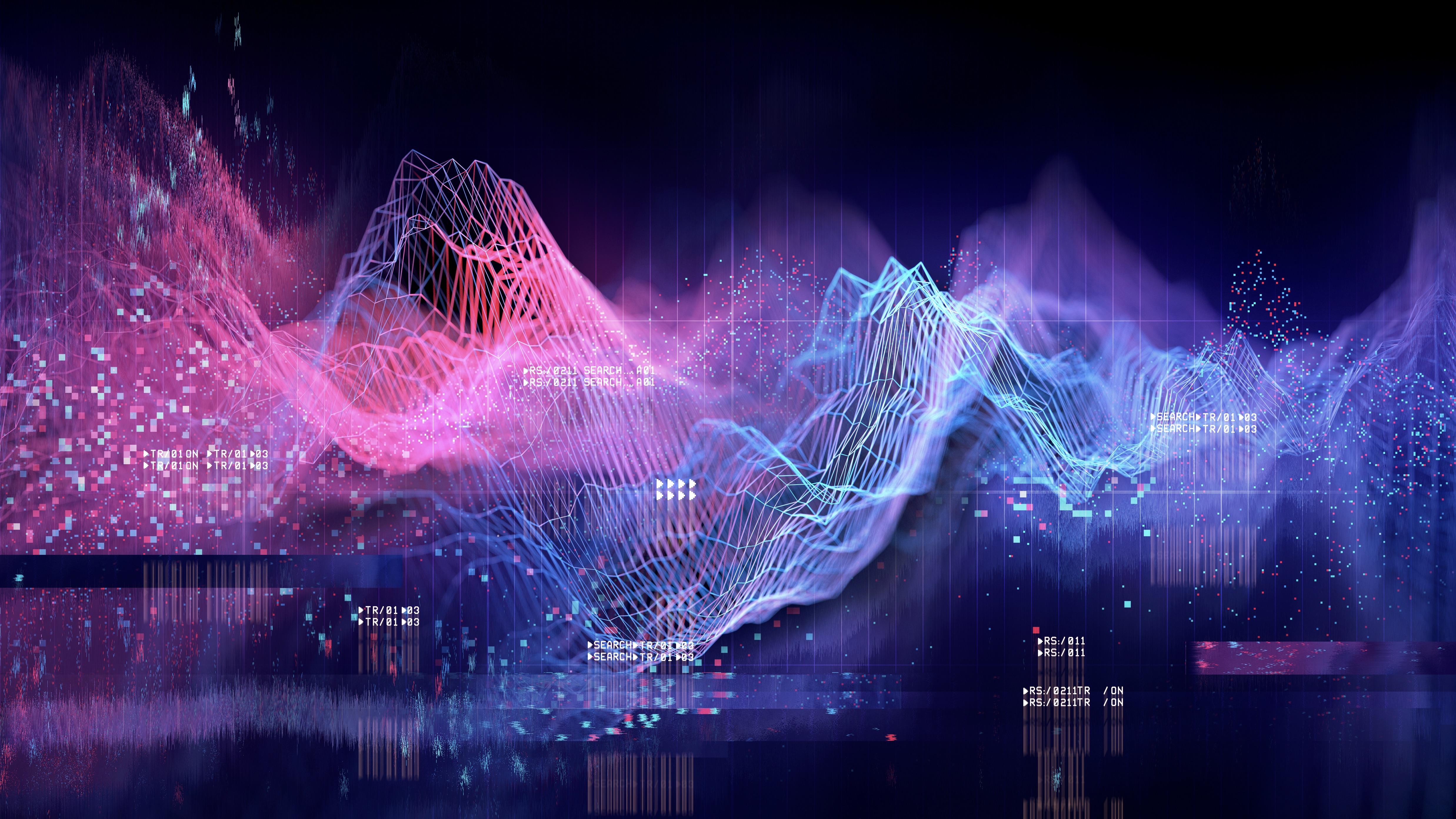
Explaining or predicting future trends and outcomes is not a straightforward task. Regardless of quality data science or determination, it's rare for predictions to be 100% accurate. In the modern world, this is where two practices come into play - namely, machine learning and statistics.
Historically, humans have been a necessary part of the statistical process as it was we who were ultimately capable of examining and interpreting data. Through machine learning, however, computers can now answer questions and make predictions in the same way.
Yet despite the fact AI and analysis models are fast becoming ubiquitous, there are still common misconceptions about the benefits of using a machine learning approach as opposed to a statistical approach. Educational direction and career opportunities are just some of the differences here, with key differentiating factors between courses in machine learning and courses in statistics.
Machine learning vs statistics: What is machine learning?
Machine learning uses computers to identify patterns in data, without the computers needing to follow explicit instructions. Instead, they are programmed with initial algorithms and models, and they learn to adapt these based on the data inputted to offer answers. However, this also means there’s less human control over the results.
Dr Michail Basios, co-founder of TurinTech, says: “Machine learning models are built for providing accurate predictions without explicit programming. Machine learning models can provide better predictions, but it’s more difficult to understand and explain them.”
Machine learning vs statistics: What are statistics?
Statistical models make their predictions from numerical data. This is often gained from questioning people and asking them to make a choice; for example, it’s how political and election polling works, or consumer shopping surveys. Human involvement is usually more involved with the interpretation of this data. “Although some statistical models can make predictions,” Basios explains, “the accuracy of these models is usually not the best as they cannot capture complex relationships between data.”
Machine learning vs statistics: What are the major differences?
Despite the fact statistics are entered into computers as part of the machine learning process, there is one overarching variation between both methods. This difference lies in the objective or desired outcome rather than in how the data is analyzed, according to Paul Clough, professor at the University of Sheffield Clough and head of AI and data science at business intelligence and data science firm Peak Indicators.
Get the ITPro daily newsletter
Sign up today and you will receive a free copy of our Future Focus 2025 report - the leading guidance on AI, cybersecurity and other IT challenges as per 700+ senior executives
Providing an example, Clough describes a comparison between the amount of chicken feed consumed by a hen and the number of eggs that chicken produces. Where a statistical analysis would look to depict the correlation between the amount of feed and the number of eggs, Clough says, a machine learning analysis would act differently. The data would instead be used to predict what number of eggs will be produced in the future.
Machine learning vs statistics: When would you use machine learning over statistics?
Clough’s egg analogy is useful in exploring this further. “The machine learning model doesn’t tell you anything about running a more efficient farm,” he suggests, “whereas the statistical model would be unwieldy if you owned tens of thousands of chickens.”
When looking at huge data sets, machine learning can be more optimal. Many concede statistics are unable to offer a deeper analysis on the relationships and correlations between the data when the levels of data are high.
They also cannot be relied on for causation, probability, and certainty because the danger is they might be misinterpreted or intentionally misused to back up a particular argument. As the famous saying goes: “There are lies, damn lies, and there are statistics.” Human involvement presents a weakness in statistics.
Basios, however, explains you can apply statistical modelling when you understand “specific interaction effects between variables” and “have prior knowledge about their relationships”. Machine learning, meanwhile, can be used when aiming for “high predictive accuracy”.
It tends to be ‘deployed’ more at the source of the data; as this is gathered and grows, algorithms automatically begin to provide intelligence. In manufacturing, for instance, it can predict when machines will need maintenance, reducing disruption. It can also analyse one option when compared to another – predicting which outcome would be better.
Machine learning vs statistics: When would you choose statistics over machine learning?
RELATED WHITEPAPER
Conceptualizing problems is a good use case for statistics, especially problems that demand close consideration. According to Graham Upton, chief architect of intelligent industry at Capgemini, statistics are also great for the creation of metrics, tables, and key performance indicators (KPIs). Statistics is focused on humans and easy to explain, AI writer and researcher Eleanor Watson adds, as opposed to machine learning.
Machine learning has, in theory, greater power in more complex scenarios, Watson goes on, such as those that involve more variables than a human would be able to deal with. The most powerful tool is not always required, though. Watson adds that many organizations tend towards the overpowered “jackhammer” of AI for surgical problems in which traditional data science would be more cost-effective and more efficient. Data science has become an “unsung hero,” Watson says, as in reality it props up the world’s current economy to a much great degree than machine learning.
Machine learning vs statistics: Is there a role for both machine learning and statistics?
Governments, businesses, and organisations are increasingly becoming reliant on machine learning as well as traditional statistics to help them make better and faster decisions. Each method, though, has its own individual drawbacks to consider.
“Statistical models have limited predictive accuracy since, sometimes, the underlying assumptions of the model are far too strict to represent reality,” Basios says. “Today’s businesses are adopting hybrid methods combining characteristics of statistical modelling and machine learning to understand in-depth how the underlying models work as well as to generate accurate predictions.”
Machine learning vs statistics: What does the future hold?
Decision intelligence (DI) is the space to understand in the future, highlights Dr Lorien Pratt, chief scientist at Quantellia. She explains it bridges both technology and the everyday decisions we all make hundreds or thousands of times to construct our world.
“There is a certain class of problem – ranging anywhere from day-to-day business strategy to the realities of climate change – that involves intangibles, complex systems dynamics, and human-machine interaction,” she says. “Machine learning, as a ‘supercharged’ form of AI, can cut through the noise and make a real difference.”
Pratt continues: “Rather than starting by thinking about the data we have, the key is to begin by asking what outcomes we want and what actions can be taken to achieve those outcomes, and then to fit the data into this action-to-outcome pathway.”
Jonathan Weinberg is a freelance journalist and writer who specialises in technology and business, with a particular interest in the social and economic impact on the future of work and wider society. His passion is for telling stories that show how technology and digital improves our lives for the better, while keeping one eye on the emerging security and privacy dangers. A former national newspaper technology, gadgets and gaming editor for a decade, Jonathan has been bylined in national, consumer and trade publications across print and online, in the UK and the US.
-
European financial firms are battling a huge rise in third-party breaches
News Growing vendor dependency has contributed to a marked rise in third-party breaches
-
‘We’ve got some fabulous conditions’: Salesforce UK chief exec Zahra Bahrololoumi touts the country's tech industry potential
News The UK remains a “priority market” for Salesforce, according to its regional CEO
-
Learning and operating Presto
whitepaper Meet your team’s warehouse and lakehouse infrastructure needs
-
Four ways AI is helping knowledge workers excel
Case Study From medical diagnostics to mining and exploration, many industries are using AI to make their workers more effective
-
How to help IT manage itself with autonomous operations
Whitepaper Using AI and automation to proactively adapt to business disruptions
-
Green Quadrant: Enterprise carbon management software 2022
Whitepaper Detailing the 15 most prominent carbon management software vendors to see if they fit your requirements
-
insideBIGData: Guide to energy
Whitepaper How big data can help energy companies manage intense disruption
-
Magic quadrant for master data management solutions
Whitepaper Informing your MDM solution choices
-
Kyndryl partners with Teradata to boost AI and data modernisation
News The new service combines Kyndryl’s data and AI expertise with Teradata’s cloud analytics platform to help customers migrate data to the cloud
-
Majority of CIOs concerned that cloud complexity exceeds human ability
News Greater observability and rollout of automated systems are also needed to reduce IT team strain and burnout