Benefits of AI and machine learning for cloud security
AI and machine learning may not be a silver bullet, but they can still play an important part in cloud security strategies
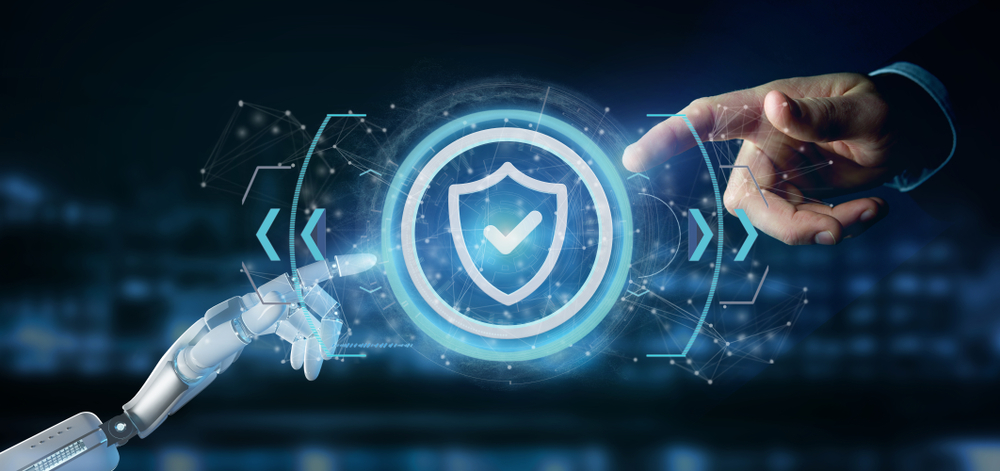
The pace of cloud adoption is only going up, with most organisations now having some kind of cloud-based processes within their operation.
However, given cyber security threats are becoming more sophisticated, it's clear more investment is needed to make cloud-based workloads as secure as they can be.
Much of that investment could be used on artificial intelligence (AI) and machine learning (ML) capabilities, to add a layer of automation to cloud security. While neither can completely reduce the chances of a breach, AI and ML can enhance the overall protection of an IT operation, with real-time data analysis and threat detection.
ML and AI programmes are some of the most sought after security services offered by the likes of Google and Microsoft. Some programmes, such as BoxShield, are even developed to limit the human element of cyber security.
Here are a few examples of how these technologies can benefit cyber security strategies.
Big Data processing
Cyber security systems produce massive amounts of data, more than any human team could ever sift through and analyse. Machine learning technologies use all of this data to detect threat events. The more data is processed, the more patterns it detects and learns, which it then uses to spot changes in the normal pattern flow. These changes could be cyber threats.
For example, machine learning takes note of what's considered normal, such as from when and where employees log into their systems, what they access regularly, and other traffic patterns and user activities. Deviations from these norms, such as logging in during the early hours of the morning, get flagged. This in turn means that potential threats can be highlighted and dealt with in a faster fashion.
Get the ITPro daily newsletter
Sign up today and you will receive a free copy of our Future Focus 2025 report - the leading guidance on AI, cybersecurity and other IT challenges as per 700+ senior executives
Event prediction
By using a more data-driven approach, artificial intelligence can be used to detect and proactively alert on weaknesses and vulnerabilities both that are being exploited right now, or that might be exploited in the future. This works by analysing data coming in and out of protected endpoints, both detecting threats based on known behaviour, and spotting yet known threats based on predictive analytics.
This more predictive approach collects all endpoint activity data rather than just the 'bad' activity, and enriches it from other sources to help address the root causes of a potential attack, rather than just minimising the effects once an attack is detected. It can also help create a shorter cycle between detection and remediation by ensuring a security team has the ability to react faster with better data.
Event detection and blocking
When AI and machine learning technologies process the data generated by the systems and find anomalies, they can either alert a human or respond by shutting a specific user out, among other options.
By taking these steps, events are often detected and blocked within hours, shutting down the flow of potentially dangerous code into the network and preventing a data leak. This process of examining and relating data across geography in real-time enables businesses to potentially get days of warning and time to take action ahead of security events.
Delegating to automated technologies
Alerts about potential threats or anomalies are very common with many security platforms, but there is a lot of potential with automated technologies to eliminate a lot of the noise to be able to focus on the important things. When security teams have AI and machine learning technologies handling routine tasks and first-level security analysis, they are free to focus on more critical or complex threats.
RELATED RESOURCE
This is particularly important given the current skills shortage in cyber security. With 51% of organisations claiming to have a problematic shortage of cyber security skills, companies can relieve some of the pressure by delegating the first level of analysis to bots, allowing security professionals to focus their efforts on combatting more difficult attacks.
This does not mean these technologies can replace human analysts, as cyber attacks often originate from both human and machine efforts and therefore require responses from both humans and machines as well. However, it does allow analysts to prioritise their workload and get their tasks done more efficiently.
-
LaunchDarkly to "double down" on observability with Highlight acquisition
News Highlight's observability tools will be integrated into LaunchDarkly's Guarded Releases software deployment service
By Daniel Todd
-
Samsung Galaxy Tab S10 FE review
Reviews The Tab S10 FE retains the feel and core capabilities of Samsung's high-end S10 tablets, but compromises on the display and the performance
By Stuart Andrews
-
The Total Economic Impact™ of IBM Security MaaS360 with Watson
Whitepaper Cost savings and business benefits enabled by MaaS360
By ITPro
-
Three ways to evolve your security operations
Whitepaper Why current approaches aren’t working
By ITPro
-
A strategic approach to security: Intelligent, collaborative, and efficient
whitepaper How your security fabric can address the challenges of new tech investment
By ITPro
-
Six steps to a stronger security posture through automation
Whitepaper Boost your cyber resilience to enable ongoing technology change with confidence
By ITPro
-
Business value of ServiceNow security operations
Whitepaper Experience transformational gains from automating workflows and data-sharing among IT, security, and risk teams to rapidly remediate threats
By ITPro
-
Thwart cyberthreats fast with security operations + AI Ops
Whitepaper How automated collaboration saves the day
By ITPro
-
Three steps to transforming security operations
Whitepaper How to be more agile, effective, collaborative, and scalable
By ITPro
-
Automation antidotes for the top poisons in cyber security management
Whitepaper How orchestration and collaboration tools can provide a healthy defense against the most serious threats
By ITPro