Why isn’t your AI delivering ROI?
How to bridge the production gap between data and IT
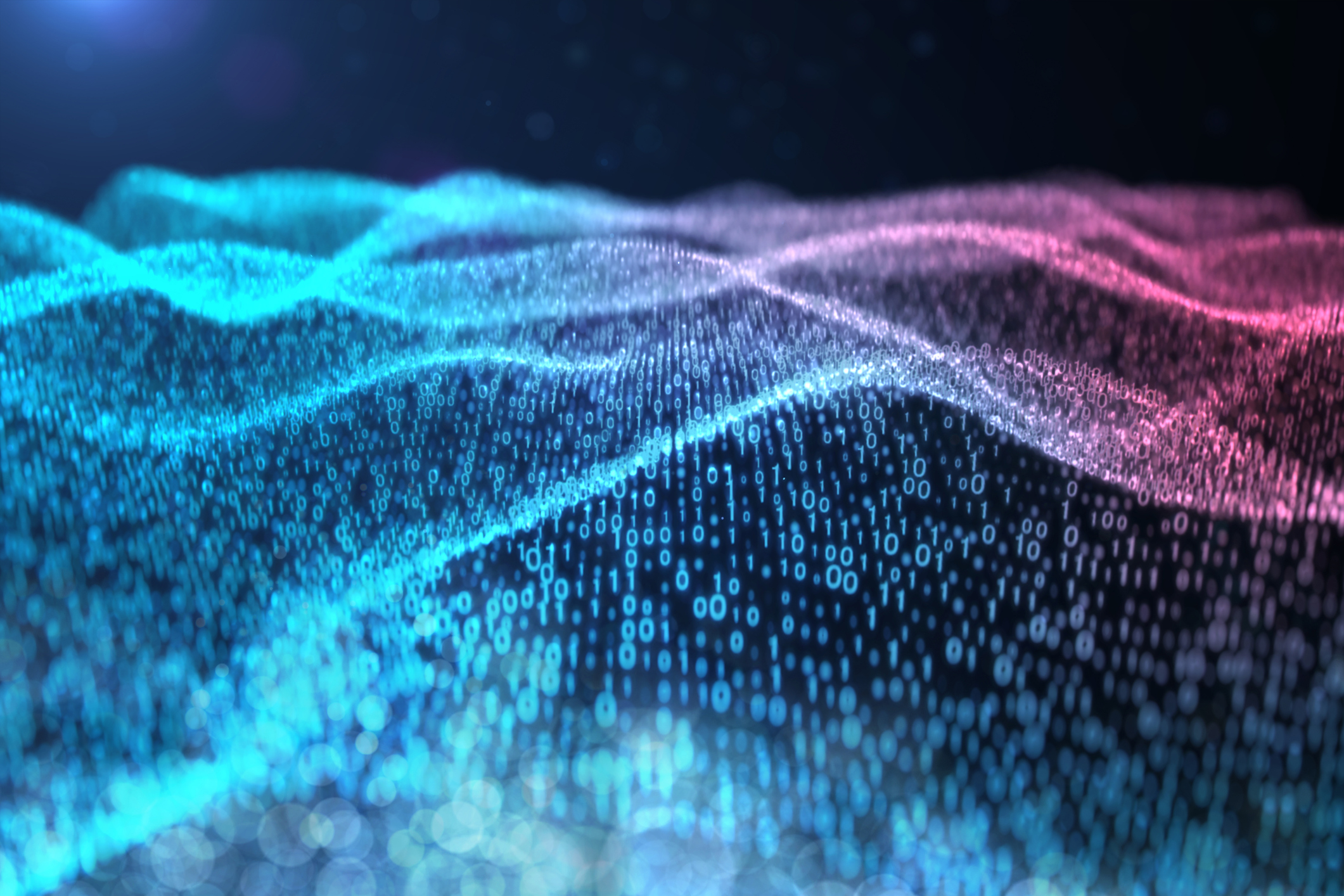
Artificial Intelligence (AI) has become a key component of companies’ bids to modernise in recent years.
Organisations have been scrambling to get an advantage over their competitors and see how greater volumes of data insights can give them an edge.
However, introducing AI without having a full understanding of where it will fit in your strategy can mean your investment won’t generate a return. But one thing’s for certain, that once it’s implemented, you need to run with it, as Gartner predicts that by 2024, 75% of organisations will initiate some form of machine learning (ML).
However, data scientists shouldn’t be extracting deep insights from company data unless they’re aligned to a business goal or solving a business problem. This can then indicate how AI and machine learning can be attributed to success.
Sivan Metzger, managing director, MLOps & Governance at DataRobot, has said that we’re now at a point where businesses will finally start to see the benefit of their AI investments, as pressure from CFOs continues to mount.
He goes on to say that data scientists should also begin to see results that can help them to adapt their work to give their company a better advantage.
Previously, this had been an issue, and Metzger credits these issues to a disconnect between the data team, IT operations and stakeholders on the business side (i.e. the potential consumers of data science insights). Data science and IT operations teams have very different considerations and goals – and machine learning is very different from running software. This disconnect is known as the ‘production gap’, and can prevent AI solutions from being properly executed.
Get the ITPro daily newsletter
Sign up today and you will receive a free copy of our Future Focus 2025 report - the leading guidance on AI, cybersecurity and other IT challenges as per 700+ senior executives
Machine Learning Operations (MLOps) is a combination of processes, best practices and underpinning technologies which seeks to bridge this gap by increasing collaboration and communication between data scientists and operations staff – and ultimately ensuring that AI is properly deployed and can begin to deliver the ROI promised.
To learn more about how MLOps can improve your returns on AI, watch IT Pro and DataRobot’s webinar ‘The Last Mile to AI ROI’, in which Metzger and data scientist Rajiv Shah discuss topics including:
- How to eliminate AI-related risks by adopting MLOps best practices
- The inherent challenges of production model deployment and how to overcome them
- Model-monitoring best practices
- Production lifecycle management and why it matters
ITPro is a global business technology website providing the latest news, analysis, and business insight for IT decision-makers. Whether it's cyber security, cloud computing, IT infrastructure, or business strategy, we aim to equip leaders with the data they need to make informed IT investments.
For regular updates delivered to your inbox and social feeds, be sure to sign up to our daily newsletter and follow on us LinkedIn and Twitter.
-
M&S suspends online sales as 'cyber incident' continues
News Marks & Spencer (M&S) has informed customers that all online and app sales have been suspended as the high street retailer battles a ‘cyber incident’.
By Ross Kelly
-
Manners cost nothing, unless you’re using ChatGPT
Opinion Polite users are costing OpenAI millions of dollars each year – but Ps and Qs are a small dent in what ChatGPT could cost the planet
By Ross Kelly
-
AI-assisted mainframe application modernization
Webinar Solve application modernization challenges with generative AI
By ITPro
-
Inside IBM’s plans to bring generative AI to Wimbledon
News Match-goers at Wimbledon this year will be armed with a range of generative AI features to enhance their experience
By Ross Kelly
-
Gartner: Data analytics teams failing to deliver benefits despite rising budgets
News Human-related challenges, such as lack of talent, were highlighted as key impediments to data strategy success
By Ross Kelly
-
Machine learning vs data science: What’s the difference?
In-depth Both machine learning and data science are fields that extract insights from data, but the methods vary significantly
By Debabrata Deb
-
Solve global challenges with machine learning
Whitepaper Tackling our world's hardest problems with ML
By ITPro
-
New bill aims to measure the military’s AI use
News Republican senator wants stronger adoption of AI to counter the technology's potential threat to our nation's security
By Danny Bradbury
-
Microsoft Azure Percept promises to make edge computing a doddle
News The company hopes to create an edge ecosystem that rivals the success of the Windows operating system
By Dale Walker
-
Don't rely on data scientists to do AI heavy lifting, former IBM exec warns
News Ex-IBM Watson lead Phil Westcott says he has witnessed “a lot of overspending and wasted resources” during his career
By Sabina Weston