Machine learning vs data science: What’s the difference?
Both intended to put data to good use, choosing machine learning vs data science requires leaders to know the fundamental differences between the two fields
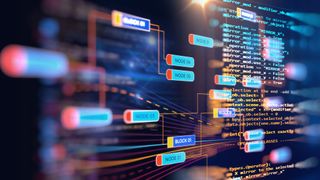
Data scientists and machine learning engineers are in hot demand as more companies look to leverage real-time data and uncover insights and trends that they can then use to gain an advantage in their industry.
The terms ‘data science’ and ‘machine learning’ may often be used interchangeably by those who aren’t experts in either field, but they are two completely separate disciplines.
In short, data science is used to turn large amounts of data into useful insights. Machine learning, on the other hand, falls under the umbrella category of AI and leans on the insights derived through data science to create projections or otherwise improve a system’s performance.
Machine learning vs data science: What are the similarities?
Machine learning and data science have the shared purpose of lifting insight from data. A data scientist will deploy statistical modeling and data vizualisation on this quest, generating structured findings from unstructured data.
Both may use algorithms, but machine learning relies entirely on algorithms to function. Modern data science projects often involve machine learning to speed up manual processes.
However, despite the similarities and overlaps between these two fields, each has distinct characteristics and uses.
Machine learning vs data science: What are the differences?
While data science brings order to disordered data, or pulls order from it, machine learning aims to learn from data to inform future actions or predictions.
Get the ITPro. daily newsletter
Receive our latest news, industry updates, featured resources and more. Sign up today to receive our FREE report on AI cyber crime & security - newly updated for 2024.
A core part of data science is data cleaning, in which erroneous data, anomalies, and non-defined values are corrected or deleted.
Machine learning relies on some level of data science, as its algorithms cannot properly use data to learn and make decisions unless it has been cleaned and proven to be accurate and reliable.
Which skills are required for data science and machine learning?
Data science requires a wide range of skills, including domain knowledge, programming, statistics, and data visualization.
A career in machine learning requires experience in computer science and mathematics. In particular, skills such as linear algebra, calculus, and probability theory are handy to be able to implement the algorithms machines will need to make predictions.
Both disciplines can come in use in a wide range of roles across various industries, including AI engineer, business intelligence analyst, data analyst and research scientist.
How are machine learning and data science used across different industries?
In technology, data science and machine learning are used by engineers to build intelligent systems and improve user experience (UX). For example, Google uses machine learning to improve its search results, and Amazon uses it to personalize product recommendations.
Financial institutions, on the other hand, would use data science and machine learning to detect fraudulent transactions, predict stock prices, and identify potential investment opportunities.
The technologies both have a clear role in healthcare. For example, machine learning algorithms can be used to identify patterns in medical images and make accurate diagnoses, while data science can be used to identify inefficiencies in medical processes and improve the management of a hospital environment.
Similarly, in retail, companies analyze customer data and make personalized product recommendations, while also working to optimize the supply chain and logistics. In transport, meanwhile, machine learning is used to optimize traffic flow, prejudice maintenance, and demand for a specific route.
Finally, data science and machine learning are widely used in research fields such as physics, genomics, biology, and environmental science to analyze and understand complex data sets.
What are some of the most common applications of data science?
There’s an array of use cases for strong data science skills across various organizations. Among these include the following.
1. Predictive maintenance for manufacturers
Data collated from sensors on a factory floor can be used to predict when a piece of machinery might break down and allow engineers to carry out maintenance before a fault occurs. Coupled with automation, this is the basis for smart manufacturing and smart ports.
2. Fraud detection
By analyzing transaction data, data scientists can build models to identify unusual behavior that may indicate fraudulent activity and help with fraud detection and prevention.
3. Market segmentation
Data scientists can segment a market and identify customer groups with similar characteristics based on enterprise data. This allows businesses to target their marketing efforts effectively.
4. Sentiment analysis
Analyzing text data by carrying out text mining can help a data scientist understand customers' perceptions of a particular product, service, and brand and help businesses make decisions accordingly.
What are the most common applications of machine learning?
Machine learning is used across the entire economy, with varying sizes and types of organizations able to leverage the approach to iteratively improve and automate business aims.
1. Forming the basis for more complex AI systems
Machine learning is used as the building blocks for widespread AI approaches such as generative AI, in which it is used to map the contextual web that connects text, images, video, and audio. At a basic level, this forms the basis for large language models (LLM) to accept user input and produce relevant outputs.
2. Image and speech recognition
Machine learning algorithms can be used to analyze images, such as for facial recognition in security systems or for computer vision systems that can help improve accessibility.
Visual data is analyzed based on known visual markers, so that a system may recognize audio patterns such as speech or discern visually between objects and people.
3. Recommendation systems
Machine learning algorithms can be used to analyze user data and make personalized recommendations for products or content.
This has become a core part of the user experience across many online platforms, with most shopping and streaming websites using machine learning to make tailored recommendations based on user data.
4. Handling language
Machine learning models can be used for tasks like natural language processing (NLP), language translation, text summarization, sentiment analysis, and much more.
5. Autonomous vehicles
Machine learning can be used to iteratively train self-navigating vehicles, such as autonomous robots on factory floors or self-driving cars. In practice, machine learning is applied to sensor data, to make low-latency decisions at the edge to ensure vehicles stay within markers and don’t endanger people.