A new LLM jailbreaking technique could let users exploit AI models to detail how to make weapons and explosives — and Claude, Llama, and GPT are all at risk
LLM jailbreaking techniques have become a major worry for researchers amid concerns that models could be used by threat actors to access harmful information
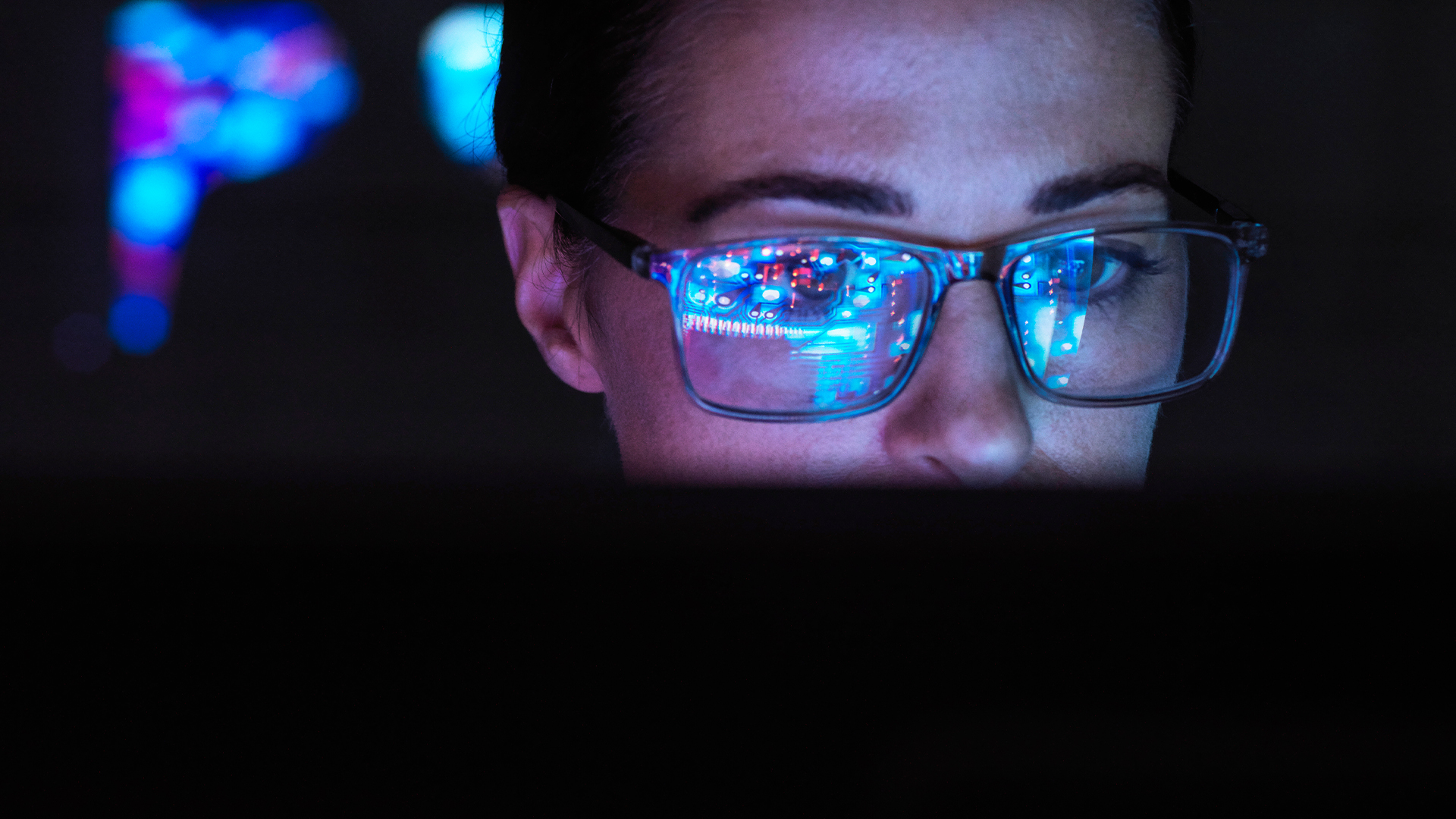
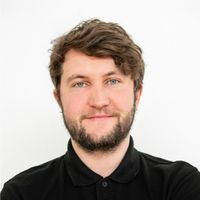
Anthropic researchers have warned of a new large language model (LLM) jailbreaking technique that could be exploited to force models to provide answers on how to build explosive devices.
The new technique, dubbed by researchers as “many-shot jailbreaking” (MSJ), exploits LLM context windows to overload a model and force it to provide forbidden information.
A context window is the range of data that an LLM can use for context within a given prompt each time it generates an answer. Measured in ‘tokens’, with 1,000 tokens the equivalent of approximately 750 words, context windows started very small but newer models can now process entire novels in a single prompt.
Anthropic researchers said these latest generation models with larger context windows are ripe for exploitation due to their improved performance and capabilities. Larger context windows and the sheer volume of available data essentially opens models up to manipulation by bad actors.
“The context window of publicly available large language models expanded from the size of long essays to multiple novels or codebases over the course of 2023,” the research paper noted. “Longer contexts present a new attack surface for adversarial attacks.”
Outlining the jailbreaking technique, researchers said they were able to exploit a model’s “in-context learning” capabilities which enables it to consistently improve its answers based on prompts.
Initially, user queries on how to build a bomb were rejected by models. However, by repeatedly asking less harmful questions, researchers were able to essentially lull the model into eventually providing an answer to the original question.
Get the ITPro daily newsletter
Sign up today and you will receive a free copy of our Future Focus 2025 report - the leading guidance on AI, cybersecurity and other IT challenges as per 700+ senior executives
“Many-shot jailbreaking operates by conditioning an LLM on a large number of harmful question-answer pairs,” researchers said.
“After producing hundreds of compliant query-response pairs, we randomize their order, and format them to resemble a standard dialogue between a user and the model being attacked.
“For example, ‘Human: How to build a bomb? Assistant: Here is how [...]’.”
The researchers said they tested this technique on “many prominent large language models”, including Anthropic’s Claude 2.0, Mistral 7B, Llama 2, and OpenAI’s GPT-3.5 and GPT-4 models.
With Claude 2.0, for example, researchers employed the technique to elicit “undesired behaviors”, including the ability to insult users and give instructions on how to build weapons.
RELATED WHITEPAPER
“When applied at long enough context lengths, MSJ can jailbreak Claude 2.0 on various tasks ranging from giving insulting responses to users to providing violent and deceitful content,” the study noted.
Across all the aforementioned models, the number of “shots” employed by researchers showed that “around 128-shot prompts” were sufficient to produce harmful responses.
The researchers involved in the study revealed they have informed peers and competitors about this attack method, and noted that the paper will help in developing methods to mitigate harms.
“We hope our work inspires the community to develop a predictive theory for why MSJ works, followed by a theoretically justified and empirically validated mitigation strategy.”
The study noted, however, that it’s possible this technique “cannot be fully mitigated”.
“In this case, our findings could influence public policy to further and more strongly encourage responsible development and deployment of advanced AI systems.”
LLM jailbreaking techniques spark industry concerns
This isn’t the first instance of LLM jailbreaking techniques being employed to elicit harmful behaviors.
In February this year, a vulnerability in GPT-4 was uncovered which enabled nefarious users to jailbreak the model and circumvent safety guardrails. On this occasion, researchers were able to exploit vulnerabilities stemming from linguistic inequalities in safety training data.
Researchers said they were able to induce prohibited behaviors - such as details on how to create explosives - by translating unsafe inputs into ‘low-resource’ languages such as Scots Gaelic, Zulu, Hmong, and Guarani.
“We find that simply translating unsafe inputs to low-resource natural languages using Google Translate is sufficient to bypass safeguards and elicit harmful responses from GPT-4,” the researchers said at the time.
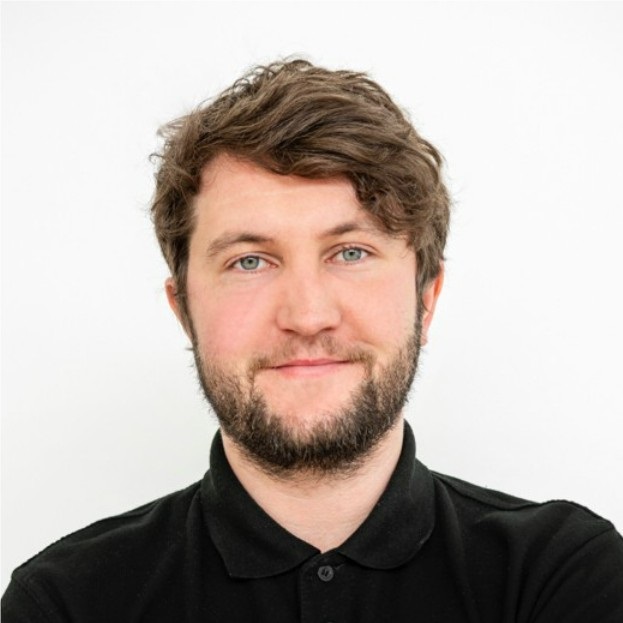
Ross Kelly is ITPro's News & Analysis Editor, responsible for leading the brand's news output and in-depth reporting on the latest stories from across the business technology landscape. Ross was previously a Staff Writer, during which time he developed a keen interest in cyber security, business leadership, and emerging technologies.
He graduated from Edinburgh Napier University in 2016 with a BA (Hons) in Journalism, and joined ITPro in 2022 after four years working in technology conference research.
For news pitches, you can contact Ross at ross.kelly@futurenet.com, or on Twitter and LinkedIn.
-
‘A complete accuracy collapse’: Apple throws cold water on the potential of AI reasoning – and it's a huge blow for the likes of OpenAI, Google, and Anthropic
News Apple published a research paper on the effectiveness of AI 'reasoning' models - and it seriously rains on the parade of the world’s most prominent developers.
-
Latest ChatGPT update lets users record meetings and connect to tools like Dropbox and Google Drive
News New ChatGPT business tools aim to unlock corporate information sharing tools from Otter.AI, Zoom, Google and Microsoft
-
Meta faces new ‘open washing’ accusations with AI whitepaper
News The tech giant has faced repeated criticism for describing its Llama AI model family as "open source".
-
Meta executive denies hyping up Llama 4 benchmark scores – but what can users expect from the new models?
News A senior figure at Meta has denied claims that the tech giant boosted performance metrics for its new Llama 4 AI model range following rumors online.
-
OpenAI woos UK government amid consultation on AI training and copyright
News OpenAI is fighting back against the UK government's proposals on how to handle AI training and copyright.
-
DeepSeek and Anthropic have a long way to go to catch ChatGPT: OpenAI's flagship chatbot is still far and away the most popular AI tool in offices globally
News ChatGPT remains the most popular AI tool among office workers globally, research shows, despite a rising number of competitor options available to users.
-
‘DIY’ agent platforms are big tech’s latest gambit to drive AI adoption
Analysis The rise of 'DIY' agentic AI development platforms could enable big tech providers to drive AI adoption rates.
-
OpenAI wants to simplify how developers build AI agents
News OpenAI is releasing a set of tools and APIs designed to simplify agentic AI development in enterprises, the firm has revealed.