Google’s new ‘Gemma’ AI models show that bigger isn’t always better
Smaller AI models are clearly the hot new commodity as Google unveils two new lightweight models, Gemma 2B and Gemma 7B
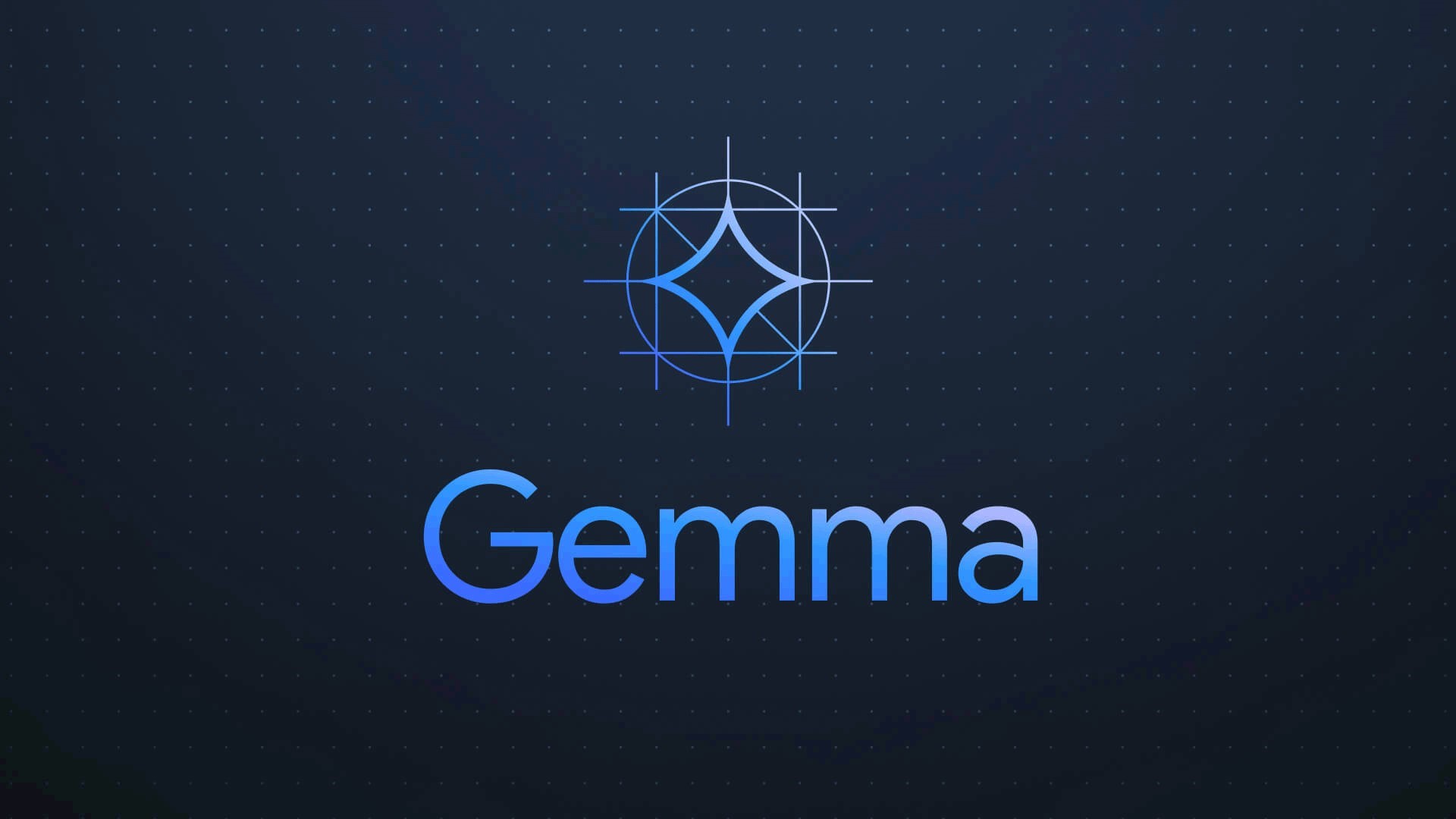
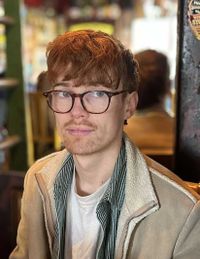
Google is rolling out two new open large language models (LLMs) dubbed Gemma 2B and Gemma 7B, built using the same research and technology that helped build Google’s Gemini.
Unlike Gemini, however, they are defined decoder-only models which are “lightweight” and focused only on text-to-text generation. Both Gemma 2B and Gemma 7B are designed with open weights, pre-trained variants, and instruction-tuned variants.
As with other language models, Google said its new offerings are well-suited to text-generation tasks like answering questions and summarizing information, though they offer these capabilities in a much smaller, easier-to-deploy package.
As Gemma is built on a smaller model size, users can utilize them with ease in environments with limited resources. These environments could include laptops, desktops, or individual cloud infrastructures.
“Google's announcement of Gemma 2B and 7B is a sign of the fast-growing capabilities of smaller language models,” Victor Botev, CTO of Iris.ai, told ITPro.
“A model being able to run directly on a laptop, with equal capabilities to Llama 2, is an impressive feat and removes a huge adoption barrier for AI that many organizations possess,” he added.
Botev here echoed the sentiments of Google, which said Gemma will help level the playing field of artificial intelligence (AI) by “democratizing access” to AI models.
Get the ITPro daily newsletter
Sign up today and you will receive a free copy of our Future Focus 2025 report - the leading guidance on AI, cybersecurity and other IT challenges as per 700+ senior executives
The attraction of smaller language models doesn’t just lie in their ease of deployment, either. For many use cases, smaller parameter counts are also more effective practically speaking, as they can be tailored to specific tasks.
Rather than using larger models and expecting them to excel at many different tasks, smaller models perform more reliably when undertaking focused tasks.
“Bigger isn’t always better,” Botev said. “Practical application is more important than massive parameter counts, especially when considering the huge costs involved with many large language models.”
“Purpose-built interfaces and workflows allow for more successful use rather than expecting a monolithic model to excel at all tasks,” he added.
The open aspect of the model is appealing as well, as Chirag Dekate, VP analyst at Gartner, told ITPro.
“Because these models are open, you can actually bring them into your enterprise data context and separate it from the internet and create a really cogent customization of game changing AI,” Dekate said.
Dekate added that open models allow a level of access to model innovation that would otherwise be proprietary and expensive.
Google is entering a “crowded marketplace” with the Gemma models
Google’s new models are far from the only smaller, lightweight models gaining traction in the AI conversation. Mistral, for example, offers a model with Mistral 7B.
Mistral 7B outperforms Meta’s Llama 2 13B on all benchmarks. The model is already gaining a significant level of popularity among developers who use it to fine-tune their own applications, according to Harmonic Security CTO Bryan Woolgar-O’Neil.
“Gemma 7B is entering a crowded marketplace of similarly-sized models,” he said.
“Google's announcement is interesting but only compares itself to Llama 2, which hasn't been state of the art for a while,” he added.
Microsoft has also been vocal about the value it sees in smaller, more bespoke models in recent months. The tech giant’s Phi-2 model, announced in December 2023, operates on a total of 2.7 million parameters.
According to Microsoft, Phi-2 matches or outperforms models up to 25x larger.
“As with all of these models, the proof will be in the pudding,” O’Neil said. “Expect to see plenty of comparisons between Gemma 7B and Mistral 7B, as well as Gemma 2B and Phi-2,” he added.
Market changes are pushing companies towards smaller model sizes
Elaborating further, Dekate said the rapid pace of innovation in the generative AI space over the last year has brought businesses to a point where they are more carefully considering things like model training and model size.
RELATED WHITEPAPER
“What we have now discovered is [that] customizing these models means we cannot just take large models and just train them on engagement,” Dekate said.
“[We now need to] think in terms of cost, accuracy, and scalability of these models,” he added.
Increasingly, businesses will likely look to smaller models like Google’s Gemma to boost the efficiency and productivity of AI rollouts,” Dekate believes.
“Last year was all about LLMs. In 2024, we will see the market evolve, if you will, [sic] to expand into SLMs, small language models, domain specific models,” he said.
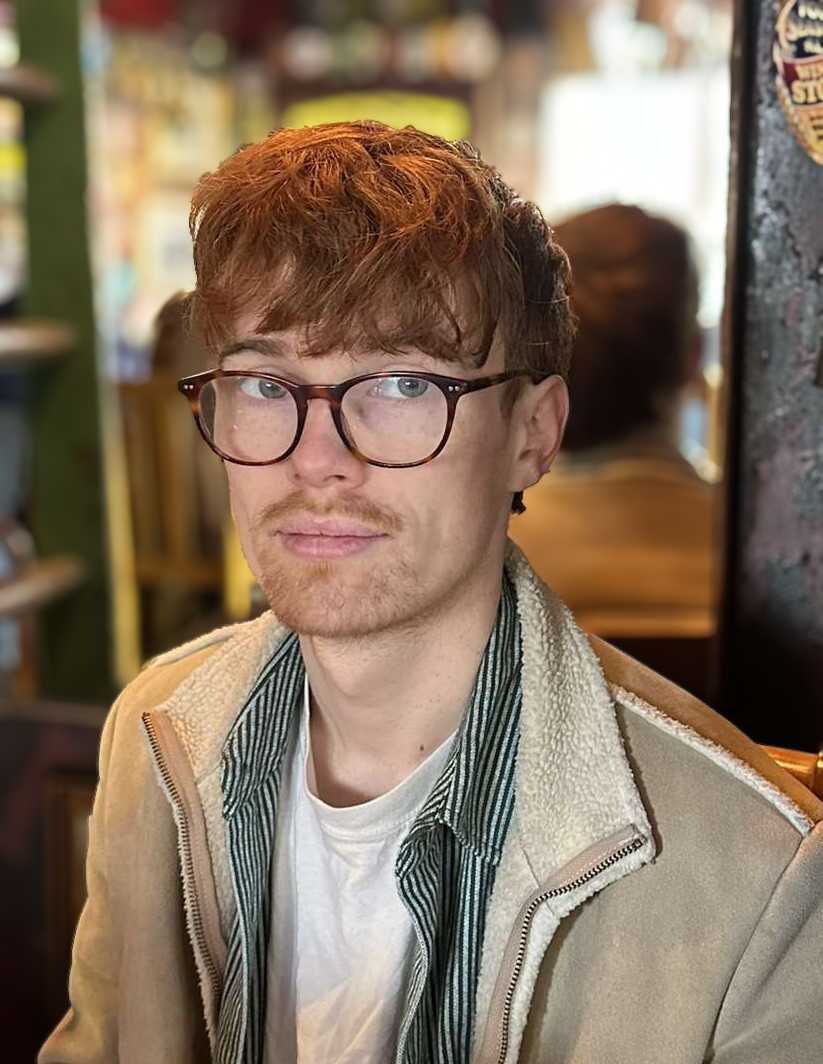
George Fitzmaurice is a former Staff Writer at ITPro and ChannelPro, with a particular interest in AI regulation, data legislation, and market development. After graduating from the University of Oxford with a degree in English Language and Literature, he undertook an internship at the New Statesman before starting at ITPro. Outside of the office, George is both an aspiring musician and an avid reader.
-
‘A complete accuracy collapse’: Apple throws cold water on the potential of AI reasoning – and it's a huge blow for the likes of OpenAI, Google, and Anthropic
News Apple published a research paper on the effectiveness of AI 'reasoning' models - and it seriously rains on the parade of the world’s most prominent developers.
-
Meta faces new ‘open washing’ accusations with AI whitepaper
News The tech giant has faced repeated criticism for describing its Llama AI model family as "open source".
-
Google Cloud is leaning on all its strengths to support enterprise AI
Analysis Google Cloud made a big statement at its annual conference last week, staking its claim as the go-to provider for enterprise AI adoption.
-
Meta executive denies hyping up Llama 4 benchmark scores – but what can users expect from the new models?
News A senior figure at Meta has denied claims that the tech giant boosted performance metrics for its new Llama 4 AI model range following rumors online.
-
Google DeepMind’s Demis Hassabis says AI isn’t a ‘silver bullet’ – but within five to ten years its benefits will be undeniable
News Demis Hassabis, CEO at Google DeepMind and one of the UK’s most prominent voices on AI, says AI will bring exciting developments in the coming year.
-
Google CEO Sundar Pichai says DeepSeek has done ‘good work’ showcasing AI model efficiency — and Gemini is going the same way too
News Google CEO Sundar Pichai hailed the DeepSeek model release as a step in the right direction for AI efficiency and accessibility.
-
The DeepSeek bombshell has been a wakeup call for US tech giants
Opinion Ross Kelly argues that the recent DeepSeek AI model launches will prompt a rethink on AI development among US tech giants.
-
Google will invest a further $1 billion in AI startup Anthropic
News This is the latest in a flurry of big tech investments for the AI startup