DeepMind's AI can lip read better than humans
DeepMind AI beats a human expert in lip reading competition
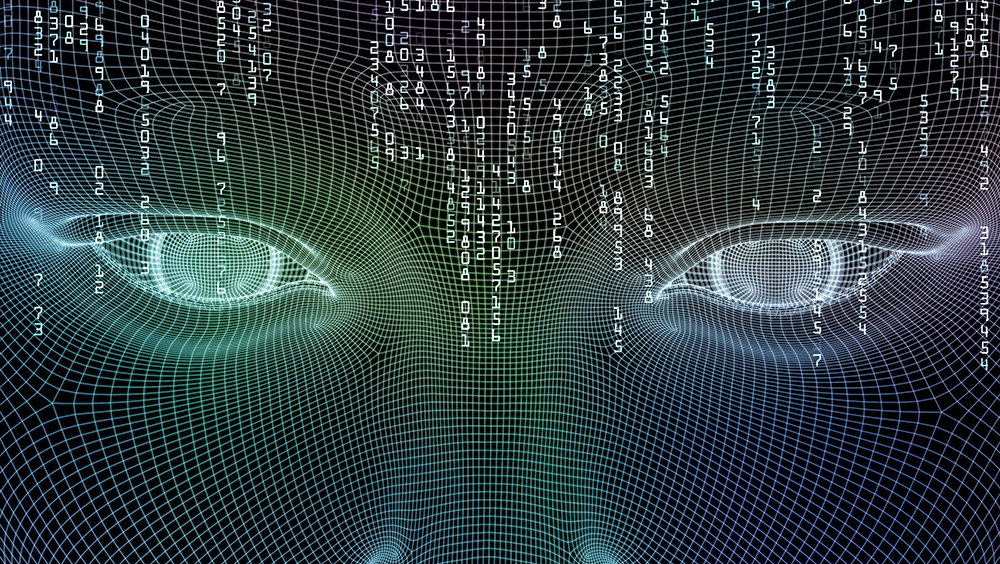
Google's DeepMind has partnered with Oxford University researchers to create a new AI that can read lips, calling it Watch, Listen and Spell (WLAS).
The researchers released a scientific paper suggesting the newly developed AI could correctly interpret more words that a trained professional in lip reading.
When tested on the same randomly selected 200 clips, a human professional lip reader was able to guess words correctly 12.4% of the time, while WLAS had an accuracy rate of 46.8%.
The paper reads: "The WLAS model trained on the LRS dataset surpasses the performance of all previous work on standard lip reading benchmark datasets, often by a significant margin. This lip reading performance beats a professional lip reader on videos from BBC television, and we also demonstrate that visual information helps to improve speech recognition performance even when the audio is available."
The system was trained on a dataset of 118,000 different sentences (17,500 words) using 5,000 hours of video footage from the BBC.
The BBC videos were prepared using machine learning algorithms, and the AI was also taught to realign video and audio when it was out of sync.
Earlier this month, the University of Oxford published a similar research paper, testing a lip reading program called LipNet. LipNet had a 93.4% level of lip reading accuracy, compared to 52.3% scored by a human expert on the same material presented.
Get the ITPro daily newsletter
Sign up today and you will receive a free copy of our Future Focus 2025 report - the leading guidance on AI, cybersecurity and other IT challenges as per 700+ senior executives
However, LipNet was tested on videos with volunteers saying formulaic sentences, with a dataset of only 51 words, whereas WLAS was tested on a much larger range of data, analysing actual conversations from BBC shows.
There are various possible applications of this lip reading technology. An AI tool such as WLAS could be of great help to improve the quality of live subtitles and better support individuals whose hearing is impaired.
It could also be a useful additional integration for virtual assistants such as Siri, as they could use the phone camera to lip read, improving their understanding of users' words even in crowded or noisy environments.
Such a tool could also be implemented for surveillance purposes, although reading lips from a grainy CCTV video could prove more challenging.
-
RSAC Conference 2025: The front line of cyber innovation
ITPro Podcast Ransomware, quantum computing, and an unsurprising focus on AI were highlights of this year's event
-
Anthropic CEO Dario Amodei thinks we're burying our heads in the sand on AI job losses
News With AI set to hit entry-level jobs especially, some industry execs say clear warning signs are being ignored
-
Future focus 2025: Technologies, trends, and transformation
Whitepaper Actionable insight for IT decision-makers to drive business success today and tomorrow
-
B2B Tech Future Focus - 2024
Whitepaper An annual report bringing to light what matters to IT decision-makers around the world and the future trends likely to dominate 2024
-
Six steps to success with generative AI
Whitepaper A practical guide for organizations to make their artificial intelligence vision a reality
-
The power of AI & automation: Productivity and agility
whitepaper To perform at its peak, automation requires incessant data from across the organization and partner ecosystem
-
Operational efficiency and customer experience: Insights and intelligence for your IT strategy
Whitepaper Insights from IT leaders on processes and technology, with a focus on customer experience, operational efficiency, and digital transformation
-
Sustainability at scale, accelerated by data
Whitepaper A methodical approach to ESG data management and reporting helps GPT blaze a trail in sustainability
-
What businesses with AI in production can teach those lagging behind
Whitepaper The more sophisticated the AI Model, the more potential it has for the business
-
Four steps to better business decisions
Whitepaper Determining where data can help your business