Why is big tech racing to partner with Nvidia for AI?
The firm has cemented a place for itself in the AI economy with a wide range of partner announcements including Adobe and AWS
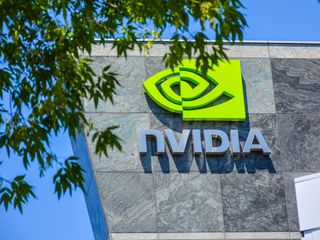
Nvidia has announced a series of big tech partnerships this week, including collaborations with AWS and Adobe to provide cloud infrastructure and hardware for training proprietary AI models.
AWS will work with Nvidia to build scalable infrastructure with the express purpose of training large language models (LLMs) for use in generative AI systems. The cloud giant’s P5 elastic compute instances run on Nvidia hardware and provide support for the largest and most complex LLMs.
Adobe and Nvidia have also announced that they will co-develop a series of generative AI models for eventual integration within Adobe’s Creative Cloud suite.
Adobe Firefly is one such model that will focus on image and text effect generation. Being trained on Adobe's own licensed content, or copyright-free material, it's tipped to be an ideal solution for businesses looking to shield themselves from potential plagiarism claims.
Why is Nvidia big tech's go-to partner on AI?
Core to the growing number of big tech agreements Nvidia has secured is its growing dominance in the AI ecosystem.
This was bolstered with the announcement of the Nvidia DGX cloud platform, an AI supercomputing service that lets enterprise customers rent Nvidia’s supercomputing servers and workstations through a web interface.
Oracle Cloud Infrastructure will be the first to host Nvidia DGX Cloud and Nvidia has already announced that other high-profile cloud services providers such as Microsoft Azure and Google Cloud will follow suit, with the tipped for adoption next quarter.
Cloud Pro Newsletter
Stay up to date with the latest news and analysis from the world of cloud computing with our twice-weekly newsletter
Nvidia’s long-running expertise in designing graphics processing units (GPUs) has also made it particularly sought after for the development of AI models.
The firm has said that GPUs are up to 20 times more energy-efficient than central processing units (CPUs) for AI work, and that its H100 GPU is up to 300 times more efficient for training LLMs than CPUs.
Microsoft’s AI supercomputer, which the firm has revealed it made in time to train both OpenAI’s GPT-3.5 and GPT-4 models, makes heavy use of the H100 for computing power.
It's not clear if this is the same ‘AI supercomputer’ Nvdia and Microsoft announced in November, or whether the firms have partnered again on a longer-term project. In either case, Nvidia has a clear role in Microsoft’s plans for AI going forward.
“Nvidia's status in AI is certainly due in large part to the performance of its GPUs, but this performance is not just a result of hardware,” James Sanders, principal analyst cloud and infrastructure at CCS Insight, told ITPro.
Flexible IT models drive efficiency and innovation
A modern approach to infrastructure management
“Nvidia's CUDA software framework provides a common platform that allows developers and users to run the same software across different models and successive generations of GPUs, making generational upgrades seamless, as well as making original development substantially easier."
Sanders added that while Nvidia does have competition in the GPU market from AMD and Intel, it benefits from its competitors' imperfect software support. On rival cards, adaptations need to be made in order to for them to support critical models such as Stable Diffusion.
At the enterprise level, Nvidia faces competition from purpose-built AI accelerators, which are technically faster than Nvidia's GPUs, but require "significant effort to adapt as existing models", Sanders said.
These accelerators also can't be found in easily accessible hardware like consumer-grade laptops, which means developers have to solely rely on cloud platforms if they want to experiment.
“You hear a lot about LLMs at the moment, but there are also a lot of other AI models such as computer vision and NLPs, and these need to be supported at both the hardware and software layer," said Bola Rotibi, chief of enterprise research at CCS Insight, to ITPro. "Nvidia is providing that, as well as choice over processors as well as AWS’ Graviton 3 processors.”
Does Nvidia face competition in this space?
AMD is a major competitor for Nvidia in the GPU market, occupying a 12% share compared to Nvidia’s 17% in Q4 2022, according to Statista.
But while the firm’s Instinct line of GPUs is specifically targeted for deep learning, it has not announced as expansive a foray into generative AI as its competitor.
“The reason why Nvidia is actively trying to use AI technology even for applications that can be done without using AI is that Nvidia has installed a large-scale inference accelerator in the GPU,” David Wang, SVP engineering for Radeon at AMD, told 4Gamer in an interview that was machine-translated from Japanese.
“In order to make effective use of it, it seems that they are working on a theme that needs to mobilise many inference accelerators. That’s their GPU strategy, which is great, but I don’t think we should have the same strategy,” he added.
Without similar investments in AI infrastructure, it is possible that AMD will miss its chance to establish a similar footing in the market as Nvidia.
In addition to its hardware pedigree, Nvidia’s growing cloud ecosystem has already attracted a number of high-profile media partners including Getty Images, Shutterstock, and financial services firm Morningstar.
With a rapidly-expanding number of partners and customers, it may already be too late for competitors to catch up.
Given AMD’s reputation as a more affordable GPU alternative, the company could become a mainstay for those looking to train open source LLMs.
However, some notable open source AI companies such as Hugging Face have already announced plans to use AWS’ AI ecosystem, which has already put Nvidia ahead in this space.
Nvidia could still find itself facing competition from new challengers in the market. Raja Koduri, formerly Intel’s VP of architecture, graphics, and software (IAGS) division, has announced his resignation in order to start a firm that will challenge Nvidia’s GPU dominance in both gaming and generative AI.
Intel itself has also released a range of hardware that it has designated for AI workloads, such as its high-performance Max Series chip family.
But, Nvidia's focus on diversifying its product offering for enterprise and cloud more broadly will likely see it continue to dominate for some time.
"Immediately, the most visible part of this is its acquisition of Mellanox in 2020 for $7 billion, which provided the company advanced networking capabilities – as AI workloads (like other enterprise workloads) spread across multiple networked servers, high-speed, low-latency networking is essential for high performance,” said Sanders.
Rory Bathgate is Features and Multimedia Editor at ITPro, overseeing all in-depth content and case studies. He can also be found co-hosting the ITPro Podcast with Jane McCallion, swapping a keyboard for a microphone to discuss the latest learnings with thought leaders from across the tech sector.
In his free time, Rory enjoys photography, video editing, and good science fiction. After graduating from the University of Kent with a BA in English and American Literature, Rory undertook an MA in Eighteenth-Century Studies at King’s College London. He joined ITPro in 2022 as a graduate, following four years in student journalism. You can contact Rory at rory.bathgate@futurenet.com or on LinkedIn.