MIT researchers hail "liquid" algorithm breakthrough
A set of differential equations at the base of an algorithm could lead to a more adaptable type of machine learning
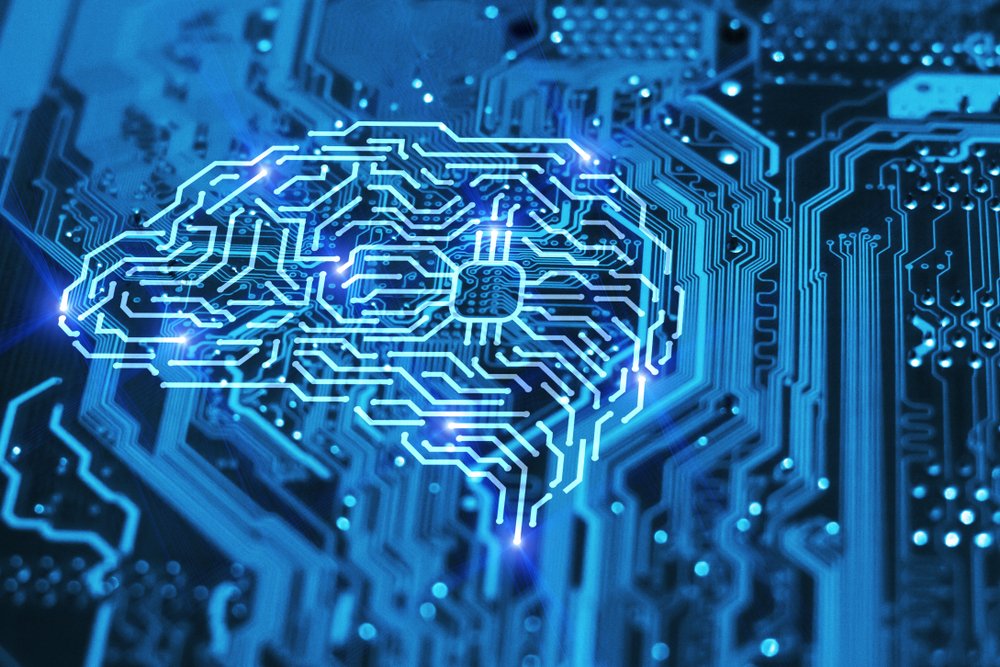
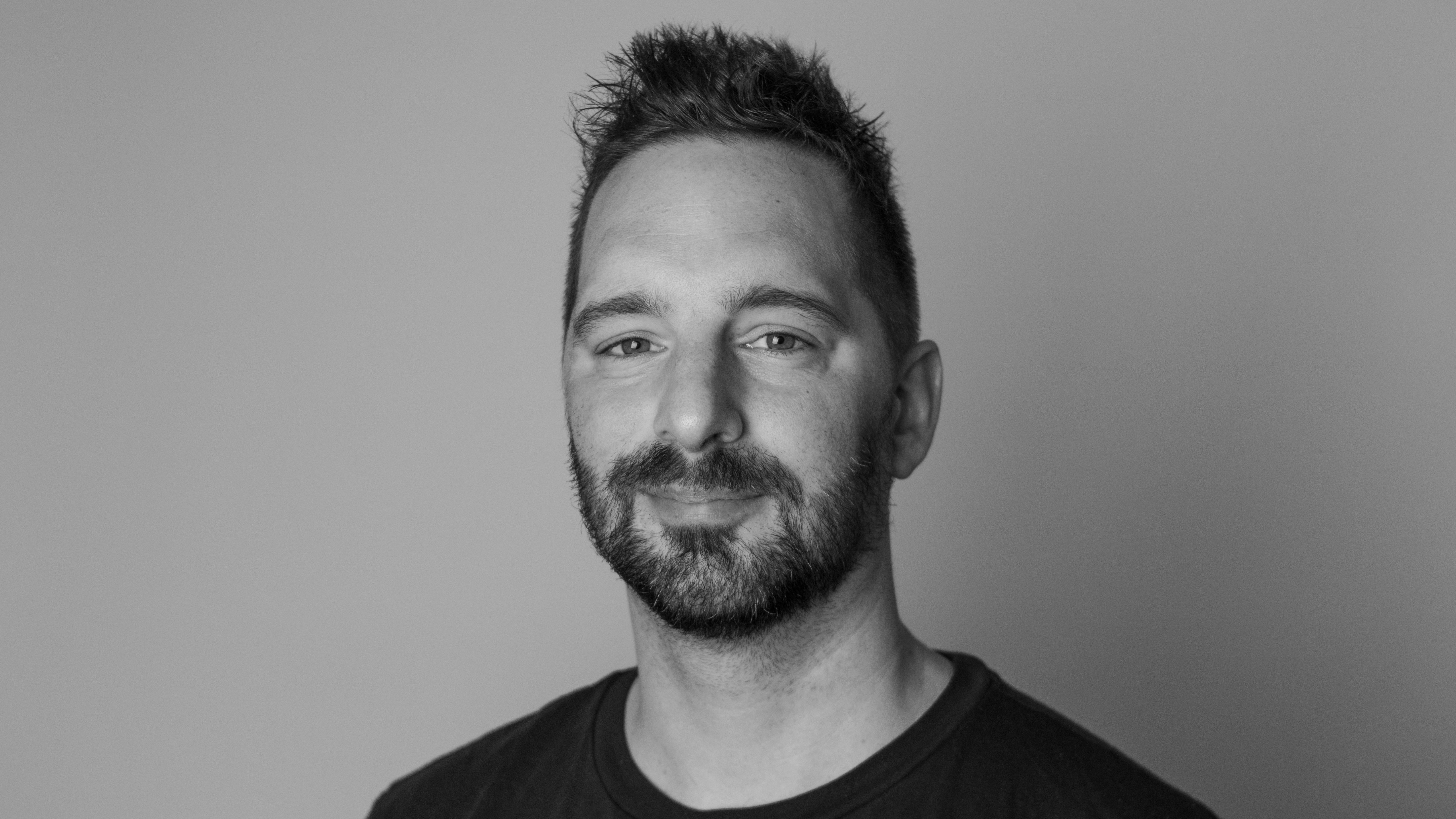
Researchers at MIT say they have developed a flexible algorithm that can change its underlying equations to continuously adapt to new inputs of data.
The "liquid" algorithm is said to be a new type of neural network that learns during tasks rather than just in its initial training phase.
It's hoped this new approach could revolutionise technology that relies on decision-making protocols where the data changes over time, or in unpredictable environments, such as medical diagnosis or autonomous driving.
The research will be presented at the AAAI Conference, an artificial intelligence event taking place in Vancouver, Canada, in February.
"This is a way forward for the future of robot control, natural language processing, video processing - any form of time series data processing," says Ramin Hasani, the study's lead author. "The potential is really significant."
Most neural networks have fixed behaviour and they typically don't adjust all that well to changes in incoming data streams. For example, the crash of an Uber autonomous vehicle in 2018 that resulted in the death of Elaine Herzberg, considered the first fatality involving the technology, was said to have been caused by the system being unable to identify the shape of a pedestrian when they were walking alongside a bicycle.
RELATED RESOURCE
Unleashing the power of AI initiatives with the right infrastructure
What key infrastructure requirements are needed to implement AI effectively?
The neural network designed by Hasani has the potential to avoid these issues by using a set of differential equations as the base of its algorithm, potentially creating a more fluid type of machine learning. The idea is inspired by the microscopic nematode, Caenorhabditis (C) elegans, which has only 302 neurons in its nervous system. Hasani said they can still "generate unexpectedly complex dynamics".
Get the ITPro daily newsletter
Sign up today and you will receive a free copy of our Future Focus 2025 report - the leading guidance on AI, cybersecurity and other IT challenges as per 700+ senior executives
Similarly, Hasani and his team used equations that allowed the parameters of his neural network to change over time. These are essentially a nested set of differential equations that change the representation of the neuron, creating a small number of highly "expressive" ones, according to Hasani.
"We have a provably more expressive neural network that is inspired by nature," Hasani said. "But this is just the beginning of the process. The obvious question is how do you extend this? We think this kind of network could be a key element of future intelligence systems."
Bobby Hellard is ITPro's Reviews Editor and has worked on CloudPro and ChannelPro since 2018. In his time at ITPro, Bobby has covered stories for all the major technology companies, such as Apple, Microsoft, Amazon and Facebook, and regularly attends industry-leading events such as AWS Re:Invent and Google Cloud Next.
Bobby mainly covers hardware reviews, but you will also recognize him as the face of many of our video reviews of laptops and smartphones.
-
M&S suspends online sales as 'cyber incident' continues
News Marks & Spencer (M&S) has informed customers that all online and app sales have been suspended as the high street retailer battles a ‘cyber incident’.
By Ross Kelly
-
Manners cost nothing, unless you’re using ChatGPT
Opinion Polite users are costing OpenAI millions of dollars each year – but Ps and Qs are a small dent in what ChatGPT could cost the planet
By Ross Kelly
-
Intel targets AI hardware dominance by 2025
News The chip giant's diverse range of CPUs, GPUs, and AI accelerators complement its commitment to an open AI ecosystem
By Rory Bathgate
-
Calls for AI models to be stored on Bitcoin gain traction
News AI model leakers are making moves to keep Meta's powerful large language model free, forever
By Rory Bathgate
-
Why is big tech racing to partner with Nvidia for AI?
Analysis The firm has cemented a place for itself in the AI economy with a wide range of partner announcements including Adobe and AWS
By Rory Bathgate
-
Baidu unveils 'Ernie' AI, but can it compete with Western AI rivals?
News Technical shortcomings failed to persuade investors, but the company's local dominance could carry it through the AI race
By Rory Bathgate
-
OpenAI announces multimodal GPT-4 promising “human-level performance”
News GPT-4 can process 24 languages better than competing LLMs can English, including GPT-3.5
By Rory Bathgate
-
ChatGPT vs chatbots: What’s the difference?
In-depth With ChatGPT making waves, businesses might question whether the technology is more sophisticated than existing chatbots and what difference it'll make to customer experience
By John Loeppky
-
Bing exceeds 100m daily users in AI-driven surge
News A third of daily users are new to the past month, with Bing Chat interactions driving large chunks of traffic for Microsoft's long-overlooked search engine
By Rory Bathgate
-
OpenAI launches ChatGPT API for businesses at competitive price
News Developers can now implement the popular AI model within their apps using a few lines of code
By Rory Bathgate