Why cutting-edge innovation is killing the planet
AI and robots will do our work, we’ll get paid in cryptocurrency, and cars will drive themselves – but each of these technologies is a massive energy hog
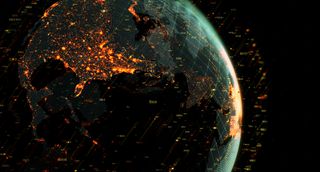
Artificial intelligence (AI), driverless cars, robotics, and other emergent technologies are already having dire climate consequences. Training a single large language model (LLM) creates the same carbon emissions of five cars over their lifetime, according to one academic paper. And it’s not just energy use, but it’s driving demand for rare-earth metals, while financial efficiencies in manufacturing can spark an increase in consumption – bad news for pollution, landfills, and emissions. And this is only the beginning.
At the moment, data centers and data transmission networks are already responsible for 0.6% of global greenhouse gas emissions, according to the International Energy Agency, though some pin the number closer to 2% – similar to global air travel emissions. The rule of thumb that a Google Search was on par with boiling a kettle has been rightly debunked, though beefing up its search engine with AI will unquestionably boost its carbon footprint – we just don’t know by how much, until that data is made available. And right now, it isn’t.
The impact of AI on climate change
Making machine learning more accurate usually means creating bigger models with more parameters churning through ever bigger data sets for training. “Since AI models are, on average, getting bigger, their energy costs are definitely rising as well,” says Sasha Luccioni, climate lead at AI developer Hugging Face. “So far, all of the numbers and research are mostly about training AI models, but we actually have very limited information about the energy costs of their deployment. Since we are increasingly putting AI models in many products and services – think navigation, smartphones, voice assistants – the energy costs of that are consequential too.”
Research suggests training large machine learning models only makes up a tenth of energy use, with running the model making up the rest. That’s true whether it’s to analyze medical data sets or generate a goofy image for a meme. And that’s merely the energy cost of compute: Luccioni’s own research reveals that considering other carbon costs, such as manufacturing components, doubles the footprint.
But, she notes, it’s worth knowing not all AI is necessarily gobbling up energy: it depends on the type and the use case. “A very small number of very large models – [such as] GPT-type models and other generative language models – are dominating the discourse when it comes to AI but, in reality, there’s a plethora of much smaller and more efficient models that are being used across the community, including for climate-positive applications like tracking deforestation or predicting the climate,” Luccioni says. “So it’s easy to think that all AI models are incredibly energy-intensive, but truly it’s only a small portion (that take up all the space right now).” In short, just because it’s AI, doesn’t mean it’s an energy hog.
Using AI and machine learning to kickstart climate change fightback
Of course, tech companies are keen to slash their energy use and, because of that, they’ve put significant effort into building dedicated hardware to boost performance and efficiency. In other words, these are already the optimized versions of such systems. What else could be done? Researchers have called for those building large models to publish energy usage, in the hopes of spurring an arms race not just in terms of capability but efficiency.
The key to slashing the energy demands, and in turn the carbon cost, of AI and related technologies? “Transparency is crucial,” says Luccioni. “Organisations should report both the training costs: compute time, hardware used, energy consumption, greenhouse gas emissions, etc, as well as inference efficiency (quantity of energy consumed per 100 queries, for instance). Otherwise, users are left in the dark regarding all of these factors and can’t use them to make informed decisions.”
Get the ITPro. daily newsletter
Receive our latest news, industry updates, featured resources and more. Sign up today to receive our FREE report on AI cyber crime & security - newly updated for 2024.
The impact of driverless cars on climate change
Waymo’s automated cars are already ferrying passengers around the San Francisco Bay Area, as are the Alphabet-owned company’s main rival, GM’s Cruise. Both use similar technologies to let their vehicles see the world around them. LIDAR scanners paint a 3D picture by pinging light pulses off everything, and measuring how long it takes to return. Cameras ring the vehicles – for Waymo, there are 19 cameras on its smaller Chrysler Pacifica cars and 29 on the larger Jaguar I-PACE – as well as radar to help improve calculations of an object’s distance and speed despite weather such as rain, fog, or snow.
All of that real-time data crunching chews through energy – though it’s difficult to know exactly how much, as that information isn’t made public and it will differ by car and by system. In 2018, researchers estimated that cars at the lower end of autonomy generate as much as 3Gb/sec of data, while cars at higher levels will have sensors spitting out as much as 40Gbit/sec of data. That figure will only have increased in the space of five years.
One challenge is understanding the growth in data processing and improvements in computing efficiency that are likely to happen before these cars become widely available, if they ever do. Academics at MIT modeled the future impact on emissions of driverless cars, finding one billion driverless cars on roads globally would lead to the same amount of emissions as all of the world’s IT data centers currently do. That’s based on the assumption that cars are used for just an hour a day, driven by a computer using 840W.
“If we just keep the business-as-usual trends in decarbonization and the current rate of hardware efficiency improvements, it doesn’t seem like it is going to be enough to constrain the emissions from computing onboard autonomous vehicles,” said study author Soumya Sudhakar, a graduate student in aeronautics and astronautics, in a statement. “This has the potential to become an enormous problem. But if we get ahead of it, we could design more efficient autonomous vehicles that have a smaller carbon footprint from the start.”
Learn about the key roadblocks to digital transformation and find out how you can accelerate it
DOWNLOAD NOW
We should have plenty of time: the study looked at all of this happening by 2050. However, to avoid driverless cars contributing to climate change, computing hardware must double in efficiency every 1.1 years. Another option, the researchers note, is to improve the efficiency of the algorithms themselves, so they require less computing power in the first place, though the difficulty is managing this without impacting accuracy.
The model doesn’t take into account autonomous cars being used differently than how we drive now, however. One idea often mooted is shifting car ownership to a shared, subscription model – essentially handing the keys to Alphabet et al and accessing a car via an app – which could potentially reduce the number of cars on the road in the first place, Still, if we’re all traveling via Waymo taxis, we’re going to need an awful lot of them.
The impact of robotics on climate change
There’s more to the environmental impact of future technologies than energy use and subsequent emissions, however. For example, the rush to embed chips in everything means more mining of key materials, such as rare earth minerals. Plus, how we use such innovations matter: giving up on public transport for corporate-owned, driverless (or even flying) taxis could exacerbate urban traffic.
Laurie Wright, a professor at Solent University, Southampton, points to the “rebound effect”: an economics observation that as we become more efficient at producing something, the price falls so we use it more. “You can play this out with AI: if we produce more stuff, more cheaply, more efficiently, that’s great – but if there’s more stuff to buy at a cheaper price, that can potentially drive up emissions,” says Wright.
Consider robots. They can take on dirty, dangerous jobs from humans, which is good news for the people who used to suffer those roles. But as robotics become more advanced, they can go further than people ever could, opening up areas of our world – and beyond – that previously were safe from environmental degradation simply by being too hard to reach. For example, robotics could allow mining in ever more remote and dangerous regions, such as deep seas – and even in space. “Is that actually a better thing to do?” asked Wright. “It’s always a complex balancing act.”
How can the industry innovate sustainably?
Should we stop using ChatGPT write silly sonnets, grammar check our WhatsApp messages, and other low-value queries? Loïc Lannelongue, a researcher at the University of Cambridge’s department of public health who runs the Green Algorithms carbon calculator, doesn’t think so – most of what we do for fun has a carbon impact. The onus shouldn’t be on those at home playing around and exploring technology to limit the carbon impact; instead, it should lie in the hands of the people building such systems. “They’re paying the energy bills, so they know what the carbon footprint is,” said Lannelongue.
He agrees with the argument that instead of an arms race for larger models, AI developers should publish the carbon footprint of training and using their models. We could then choose not the biggest, but the most efficient. “It’s just a cost-benefit analysis that we need to do beforehand,” says Lannelongue. “And the only way is to know the cost.”
Without counting the cost, there’s no reason to reduce computing use. The classic example, Lannelongue says, is tuning the hyperparameters of a machine learning model; it’s impossible to know when you’ve hit the best combination of parameters, so researchers run many different combinations to find performance improvements. “Imagine it’s 5pm, you’ve been running tests all day, and you think you’ve hit the plateau [in performance],” he says. “As financial costs are negligible, it’s tempting to just let it run overnight and do as many tests as it can, because I’m sleeping and it doesn’t matter… but the extra half percent you might get could get you published at NeurIPS.”
That’s the sort of behavior Lannelongue wants researchers developing AI to think twice about. “The whole point of the project is not to say we shouldn’t do computing anymore,” he says. “We just need to acknowledge that there is a carbon cost.” And hopefully planting that seed will remind people to only compute where necessary, to not waste energy, and to double-check their work before running complex calculations: “It’s happened to everyone that you run a massive thing and only at the end realize you forgot to write the outputs… and have nothing to show for it.”
Tracking the impact, helped by projects such as Hugging Face and Green Algorithms, can help us understand the environmental costs of future technologies, and hopefully encourage developers to build in mitigations such as energy efficiency. But in the meantime, adding generative AI to search, driverless automation to cars, and using robots for everything else might be convenient and intriguing for us, but less so for the planet. When we talk about human-centric, ethical AI, we should probably consider clean air and tolerable temperatures as key components of safe technology.