Four reasons to be excited about the future of AI – and three reasons to worry
Smaller models and liquid networks are some reasons to be excited about AI, but leaders will have to keep track of AI risks and emissions
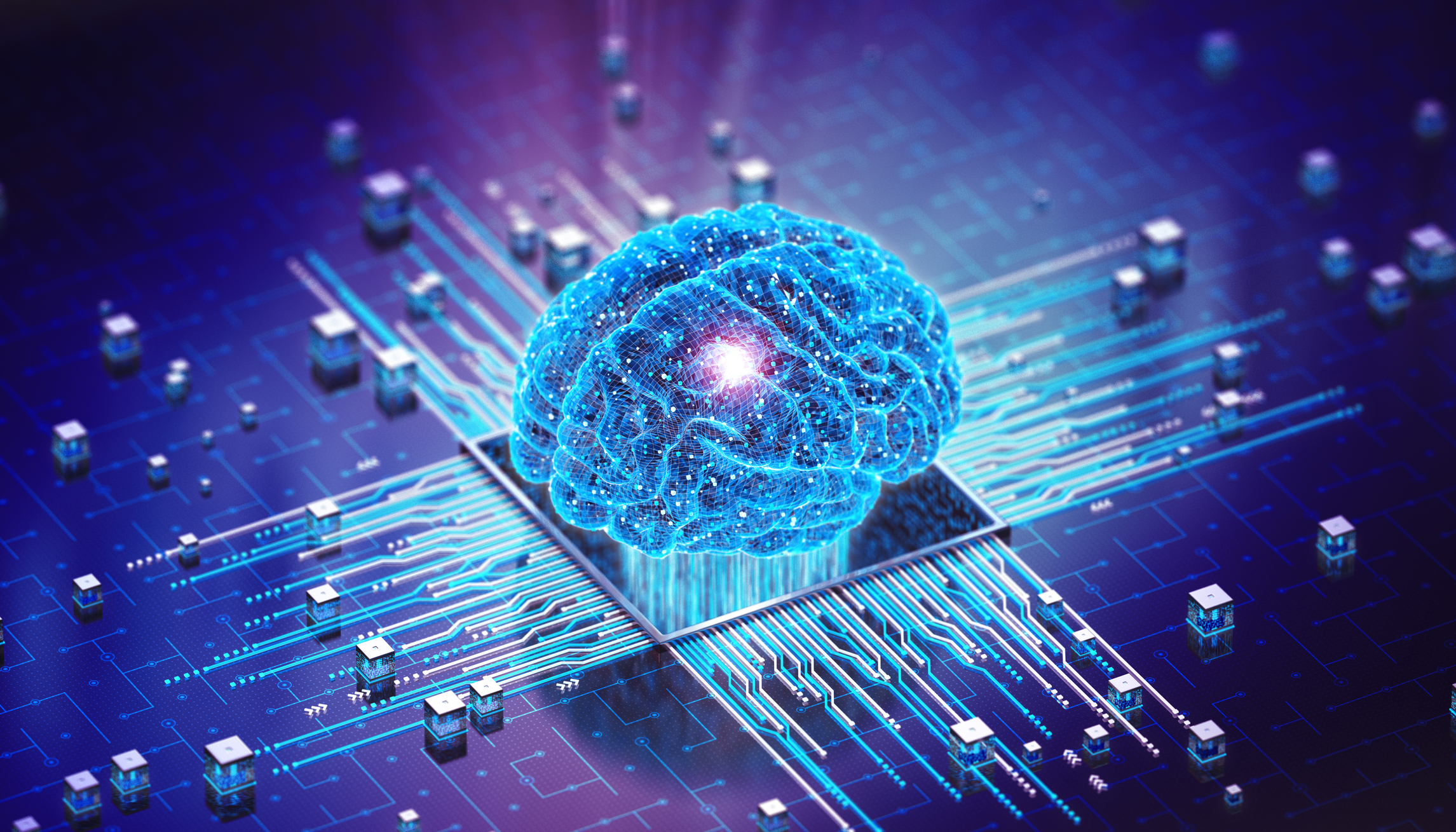
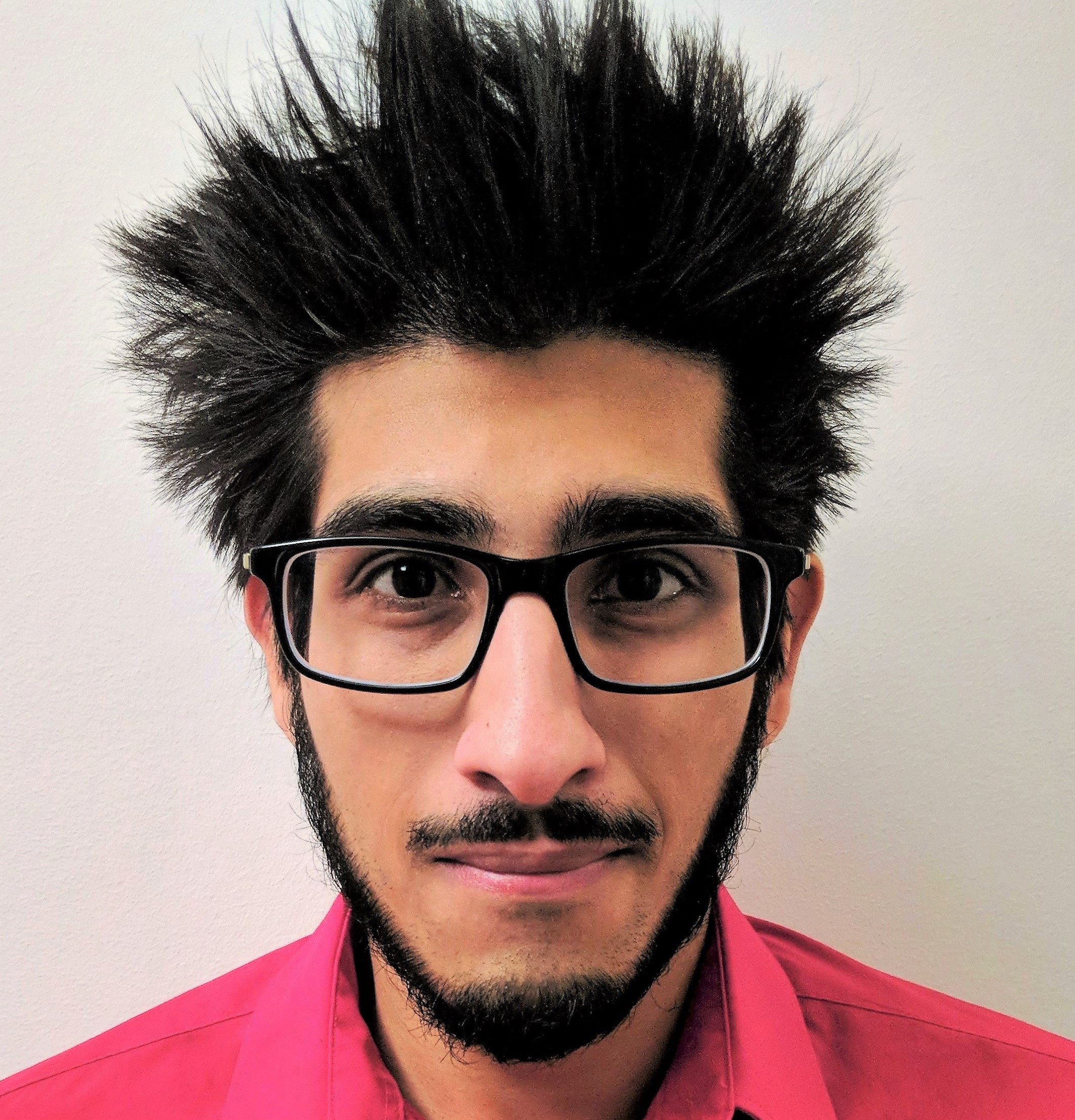
Sandra Vogel
In just the past two years, AI has seen new levels of uptake and generative AI has captured the attention of enterprises all over the world.
There are many reasons to be excited about AI, from the creation of models that are easier for businesses to use to multimodal AI that will allow AI tools for business to process data even more intelligently. But life in the AI garden is not all rosy. Like any technology, AI has pros and cons and the past year has seen talk of potential AI threats.
Look, for example at the UK’s AI Safety Summit, which took place in November 2023 at Bletchley Park. A key outcome of this event was the Bletchley Declaration – an agreement between 28 countries including the UK, US, and China, focused on shared efforts to understand the risks AI poses.
Academics also take a broad view, building on a long corpus of research to anticipate AI’s potential as well as its challenges as the field continues to evolve. Where we are right now with AI, there is much to still figure out on the business side, such as monetization strategies, but there are also plenty of ethical and security challenges.
Four reasons to be excited about the future of AI
1. Mass availability of LLMs
The better access firms have to AI models, the more innovation there can be in the space. This theory follows those made when personal computers or the internet first hit the market. There’s certainly no sign, at the time of writing, of AI development slowing down and with a broader choice of models firms will be better able to choose those that best fit their business interests.
Broadening access could also alleviate concerns about inherent biases in large language models (LLM) get set aside. These concerns are real and can mean, for example, that prevailing perspectives are embedded in AI outputs, effectively leading to narrow, potentially exclusive outcomes.
The open source community is playing a key role here, with open source key to the future of generative AI. Matched with an ethical AI approach, open-source development can help businesses unlock the true value of AI without having to worry about vendor lock-in, while also allowing them to meet regulatory requirements over the transparency of their weights and training data.
Get the ITPro daily newsletter
Sign up today and you will receive a free copy of our Future Focus 2025 report - the leading guidance on AI, cybersecurity and other IT challenges as per 700+ senior executives
2. Multimodal AI
The future of AI lies in with multimodal models, meaning those that in addition to text can process images, video, audio, or other forms of input.
RELATED WHITEPAPER
Multimodality is a route to more sophisticated AI models, as it opens the door to models that can assess a problem using multiple kinds of data at once. For example, at Google Cloud Next 2024, Google used its multimodal LLM Gemini 1.5 Pro to provide a summary of the day-two keynote, with the model assessing the video and audio from the event.
Meta has also published information about how multimodal generative AI can work with a brand of smart glasses it is associated with, for example by providing text-based outputs to speakers in the glasses and to an app, with the app also displaying images. This could revolutionize accessibility in tech, or provide those in a manufacturing or healthcare department with an AI copilot for on-the-fly analysis.
3. Liquid neural networks
One of the most exciting innovations to emerge from MIT in recent years has been the idea of liquid networks, and it’s the current focus of Daniela Rus, professor of electronic engineering at MIT and director of its Computer Science and Artificial Intelligence Laboratory (CSAIL).
Described as a new approach to machine learning (ML), liquid networks take continuous approach to deep learning for applications with time-series data.“If you do a very good job of trying to curate your data, you can find these opportunities to process on orders of magnitude less data,” he says.
“While the world is trying to make networks bigger and bigger, I want to make them smaller,” says Daniela Rus, professor of electronic engineering at MIT and director of its Computer Science and Artificial Intelligence Laboratory (CSAIL), speaking at the Databricks Data + AI Summit 2023.
“If you want to get a robot car to stay in lane and steer, it takes about 100,000 different networks to get good behavior and it only takes 19 of our liquid networks.”
Liquid networks aren’t appropriate to every situation that could benefit from AI. But in addition to their applications when it comes to autonomous robots they carry great potential in areas where data is fluid such as climate modelling or stock market analysis.
4. Small language models
Today’s broad approach of scraping as much data as possible to build models is problematic. Aside from ongoing questions of copyright in textual material, scraped data is notoriously “dirty” – error strewn, lacking control, featuring duplication and contradiction.
Garbage in, garbage out may be a very old term in computing, but that doesn’t mean it has no relevance in 2024. Smaller models with highly curated data would be cheaper to build and require fewer resources to compete with larger models.
Smaller AI models are also a good fit with local AI setups which allow applications to run in-house, including on standalone computers or for edge computing. Running AI inference locally has several advantages, including:
- Computational efficiency – lowering AI’s carbon footprint and implementation cost.
- Faster computation as even the small latency involved in cloud-based systems is reduced, aiding real-time or extremely high traffic needs.
- Greater privacy and security as no potentially vulnerable external servers are in use.
Examples of small language models already in use include Google’s Gemma family of models, which come in 2-billion 2B or 7B parameter size options, Llama 3’s 8B parameter model, or Apple’s 1B OpenELM family.
Three reasons to worry about the future of AI
1. Continuing concerns over AI risks
These models have plenty of security and privacy challenges. For example, as AI services will be “exposed” to the general user, there are concerns around responsible usage, explains Dawn Song, professor of computer science at UC Berkeley. Recent abuses of AI models include a new LLM jailbreaking technique which could let hackers hijack the models to output instructions on bomb-making or other normally restricted responses.
At present, concerns that generative AI could present a major risk through direct attacks that would see LLMs used to generate malware may have been overstated. Researchers recently found that very few AI models could be used by hackers to prey on zero-day exploits, with only OpenAI’s GPT-4 found capable of reliably posing a threat.
2. AI’s massive carbon footprint
There are three categories of AI right now, according to Rus; solutions around pattern recognition, systems primarily designed to come to a decision, which is where reinforcement learning comes in, and finally there’s generative AI.
“In each of these three categories we have issues; we have issues around data, because they all require a lot of data. And that means the computation is huge,” says Rus. “That also means there’s a large environmental footprint.”
An MIT study published in December 2023 calculates carbon emissions from AI. One example cited is that “Generating 1,000 images with a powerful AI model, such as Stable Diffusion XL, is responsible for roughly as much carbon dioxide as driving the equivalent of 4.1 miles in an average gasoline-powered car.”
Meta releases detailed information about the direct emissions from training its models. Its latest, most powerful model Llama 3 took 7.7 million GPU hours of training, emitting 2290 tons CO2 equivalent (tCO2eq), which the firm says it offset through its sustainability program. This is an illustration of the scale of emissions linked to AI, with 2290 tCO2eq roughly 572 times the tCO2eq per person, worldwide.
3. Big tech monopolizing AI
Because AI is such a powerful tool, with so many different applications, a significant danger as the fledgling industry evolves is that world-leading tech companies including the likes of Microsoft and Google dominate the field and shape development.
The alternative to this vision relies on an expansion in, for example, open source development, as well as in-house development of generative AI systems in which individual enterprises own the IP to the models they use. Strides in this direction are being made, but
For example, experts have questioned how open the leading open source AI models really are, with the likes of Meta and Databricks receiving praise for their approach but still falling short of the technical definition of ‘open source’.
Meta’s flagship model Llama 3 has certain restrictions, such as one which prohibits companies with more than 700 million monthly active users from using the model unless they receive express permission from Meta. DBRX, Databricks’ leading model, has a similar clause.
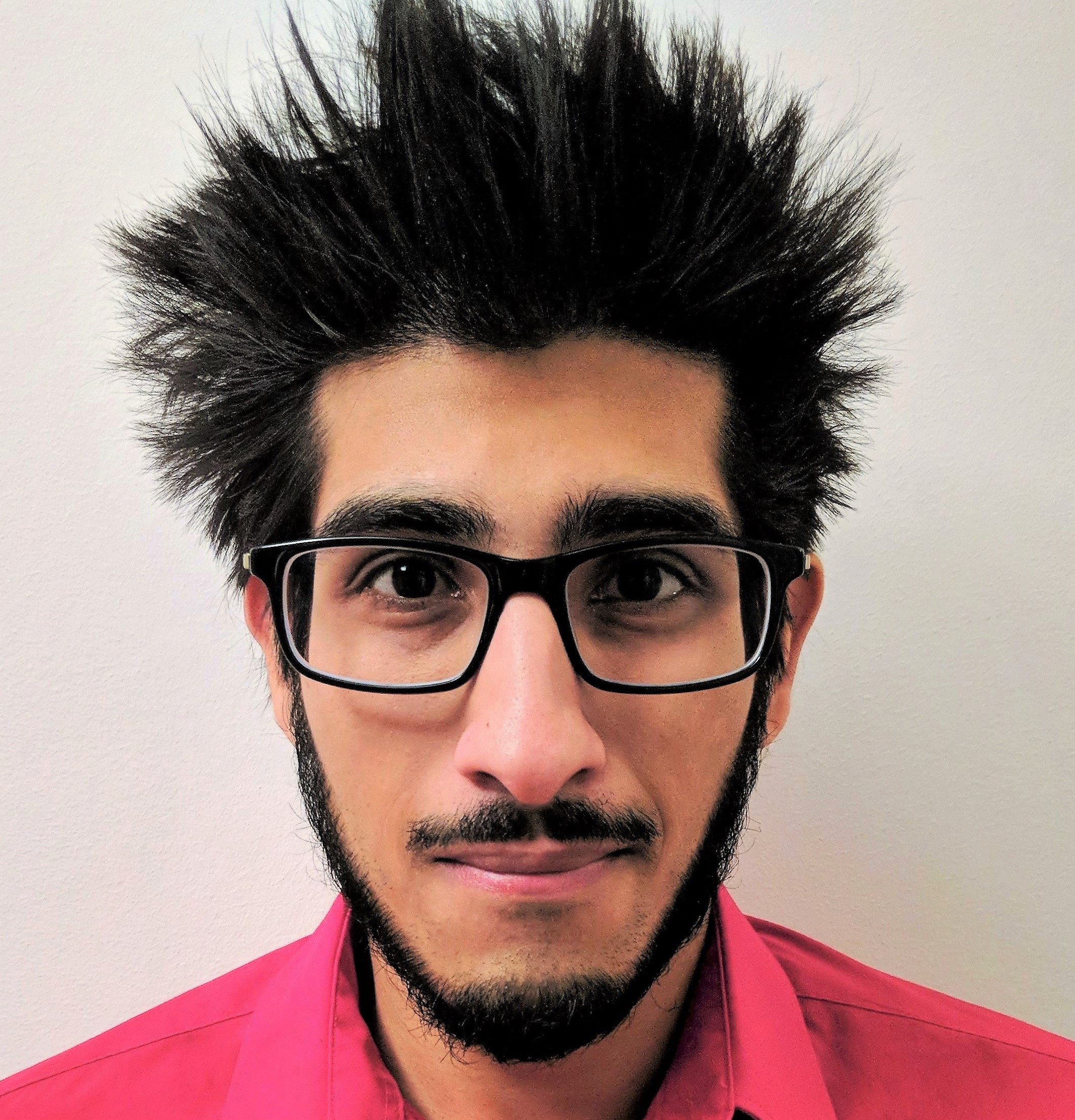
Keumars Afifi-Sabet is a writer and editor that specialises in public sector, cyber security, and cloud computing. He first joined ITPro as a staff writer in April 2018 and eventually became its Features Editor. Although a regular contributor to other tech sites in the past, these days you will find Keumars on LiveScience, where he runs its Technology section.
- Sandra VogelFreelance journalist
-
M&S suspends online sales as 'cyber incident' continues
News Marks & Spencer (M&S) has informed customers that all online and app sales have been suspended as the high street retailer battles a ‘cyber incident’.
By Ross Kelly
-
Manners cost nothing, unless you’re using ChatGPT
Opinion Polite users are costing OpenAI millions of dollars each year – but Ps and Qs are a small dent in what ChatGPT could cost the planet
By Ross Kelly
-
Databricks expands AWS partnership to drive generative AI capabilities
News The new agreement promises “unmatched scale and price performance” to help customers take genAI applications to market faster
By Daniel Todd
-
OpenAI, others pushing false narratives about LLMs, says Databricks CTO
News Tech giants are widely overstating AI dangers, as well as the costs of building generative AI platforms, to maintain a stranglehold on the emerging field
By Keumars Afifi-Sabet
-
JPMorgan Chase vows to avoid rolling out generative AI until problems are resolved
News Tech chief Larry Feinsmith cites hallucinations and the potential for misuse as his biggest concerns
By Keumars Afifi-Sabet