Google Cloud targets ‘AI anywhere’ with Vertex AI Agents
AI choice across Google Cloud’s ecosystem has massively expanded, as the firm casts a wide net and emphasizes open models
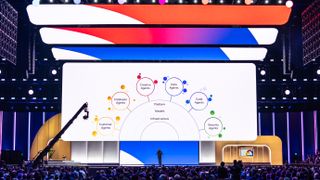
Google Cloud has announced an end-to-end suite of AI updates across its platform, as it leans on its Vertex and Gemini offerings to help customers achieve ‘AI anywhere’.
A core offering touted throughout Google Cloud Next, the firm’s annual conference held this year in Mandalay Bay, Las Vegas, has been AI ‘agents’ accessible through its Vertex AI platform.
Developers can set out custom tasks and capabilities for agents using natural language in Vertex AI Agent Builder, a new no-code solution for deploying generative AI assistants. Rooted in Google’s Gemini family of models, the agents can then complete simple or complex tasks as enterprises see fit.
In conversation with assembled media, Will Grannis, VP & chief technology officer at Google Cloud, described Vertex AI Agents as “at the top” of Google Cloud’s sophisticated AI ecosystem.
“Moving from natural language processing bots sitting on top of a website to intelligent agents that can take context from an organization, its brand guidelines, its knowledge of its own customers and use that to create new experiences, more efficient workflows, changing customer support significantly, agents as a manifestation of all that underlying innovation … it’s a world away from a year ago.”
In the opening keynote of the event, attendees were shown demos in which Vertex AI Agent could identify products within a company’s stock based on a text description of a video, or answer detailed employee questions on their company’s healthcare plan.
Via API connections, the agent could then recommend specific orthodontic clinics near to the user and pull through reviews from Google for each.
Get the ITPro. daily newsletter
Receive our latest news, industry updates, featured resources and more. Sign up today to receive our FREE report on AI cyber crime & security - newly updated for 2024.
Agents are just one avenue for connecting AI with customer data, and Google Cloud has repeatedly stated its goal of providing as many choices for its customers as possible. Another can be found in advancements with Google Distributed Cloud (GDC)
An ‘AI anywhere’ approach via Google Distributed Cloud
Through updates to GDC, Google Cloud has doubled down on its desire for customers to plug AI into their data wherever it lies.
With generative AI search capabilities for GDC, businesses will be able to use natural language prompts to find enterprise data and content. Google Cloud said that as the service is based on Gemma, Google DeepMind’s lightweight, open model, all queries and outputs will remain on-prem to keep data secure.
Businesses will be able to search data in GDC edge and air-gapped environments using AI, in much the same way as Vertex AI Agents operate elsewhere in their stack.
It’s another avenue by which businesses can unlock AI in every corner of the working day, even when that involves using AI to trawl sensitive data that never interacts with the public cloud. The feature is expected to enter preview in Q2 2024.
Through its managed approach, Google Cloud also aims to bring its cloud services to its customers’ data, including at the edge. This means that, should they wish, customers can use GDC’s cross-cloud network functionality to search air-gapped data using natural language prompts as seamlessly as they could trawl data in the public cloud.
This uses Vertex AI models and a pre-configured API for optical character recognition (OCR), speech recognition, and translation.
Two more announcements that center on surfacing data with AI include Gemini in BigQuery and Gemini in Looker, which allow workers to use prompts to discover information for data analysis.
Both run on Gemini 1.5 Pro, the latest version of Google Cloud’s in-house Gemini family of large language models which is now generally available on Vertex. The model will feature a sizeable 128k token prompt window, alongside the capacity for a gigantic one million tokens for specific circumstances.
In the opening keynote, Google Cloud showed a demo in which Gemini in Looker could answer questions on correlation between sales and data and produce graphs based on this data. As Gemini 1.5 Pro is multimodal, the model can also draw information from images.
In the keynote demo, the user could find out product sales and supply chain information by asking for all results that looked similar to a specific product.
Similarly, Gemini in BigQuery can be used for powerful data analysis across an enterprise’s data using natural language inputs. As part of its commitment to achieve ‘AI anywhere’ without the need for businesses to shift or sanitize their datasets, BigQuery and Looker can connect to Vertex AI natively to allow models to connect directly with enterprise data.
An open, mix-and-match approach to models
A key selling point of Google Cloud’s platform throughout Google Cloud Next so far has been its pursuit of the widest possible range of models, architecture choices, and avenues for training. A number of models are newly available on the platform, including all the iterations of Anthropic’s Claude 3, Google’s latest image generation model Imagen 2, and a new lightweight, open code completion model named Code Gemma.
Grannis described Vertex as a platform intended to provide customers with “almost any” model that exists and empower them to train, tune, and ground these on an enterprise’s unique data.
Businesses will be able to use Google Search to ground outputs from models, which Google Cloud maintains will help ensure models align with facts and more reliably provide verifiable information. Customers seeking to use their own data to ground models will be able to plug agents directly into information from platforms such as Salesforce or BigQuery.
“One of the more profound things in that layer is the grounding,” Grannis said.
“So Google Search native with the Gemini API as well as authoritative search for your own documents, your unstructured data, structured data, all part of Vertex AI. So taking a model and actually making it useful in the context of an industry or use case.”
“The real outcomes don’t come from the first shot,” said Grannis. “The real outcomes come from iterative development and exploring what these models can do, what they don’t do, what data you have and don’t have, and being able to combine that insight into your development workflow.”
“Think of Vertex as an opinionated MLOps platform that democratizes experimentation and generative AI,” added Grannis.
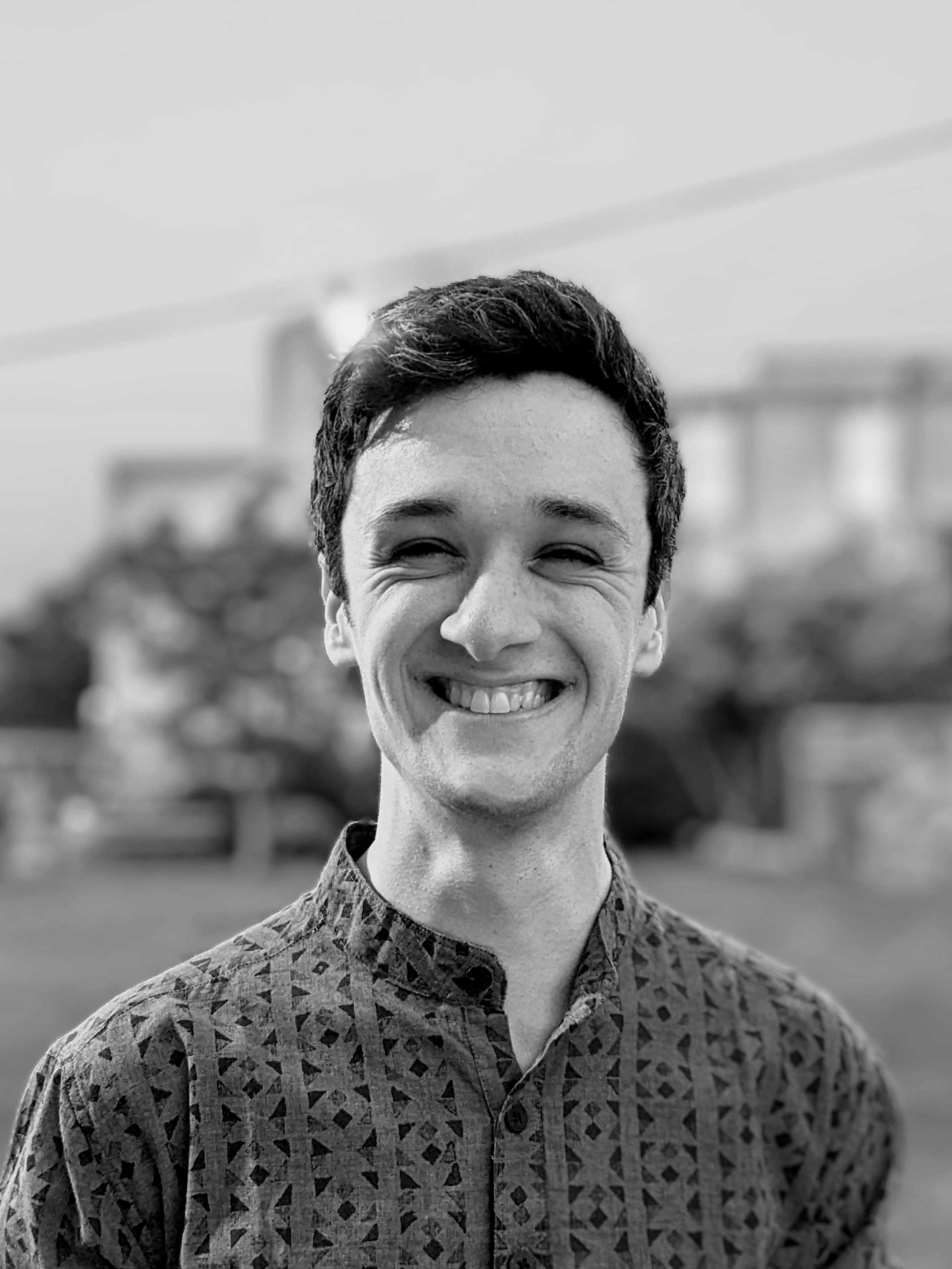
Rory Bathgate is Features and Multimedia Editor at ITPro, overseeing all in-depth content and case studies. He can also be found co-hosting the ITPro Podcast with Jane McCallion, swapping a keyboard for a microphone to discuss the latest learnings with thought leaders from across the tech sector.
In his free time, Rory enjoys photography, video editing, and good science fiction. After graduating from the University of Kent with a BA in English and American Literature, Rory undertook an MA in Eighteenth-Century Studies at King’s College London. He joined ITPro in 2022 as a graduate, following four years in student journalism. You can contact Rory at rory.bathgate@futurenet.com or on LinkedIn.