What is small data and why is it important?
Amid a deepening ocean of corporate information and business intelligence, it’s important to keep things manageable with small data
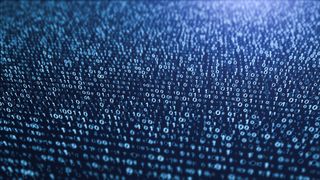
The volume of data in circulation is growing on an exponential basis, with much of this data being fed into systems to help businesses gain insights they can act on. Humans still need to be able to read and process data, though, in their own right, which is where small data comes into play.
One might think of small data as the definition of data as we once knew it – data on a scale that’s easy for most people to comprehend, digest and act on. With the rise of big data and big data analytics, though, has come the tendency to forget about the importance of small data in a modern business context.
As trendy (and useful) as big data might be, only small data can actually allow us to make sense of why these trends machines help us see are happening. As such, while big data technologies can find patterns and correlations, small data helps us understand the context.
What is small data?
What makes small data ‘small’ isn’t the data itself, but the scope of the query. You can think about small data as corresponding to an infographic. This type of visualisation is designed to tell a strictly specific story with an absolute minimum of necessary context (or none).
You’re not expected to dive into the data or pivot it into different dimensions: nobody has so far come up with a standard for live, tappable infographics, and that’s probably a good thing. That’s not to say that such a presentation wouldn’t have its uses. It could be valuable to the mobile gaming development community, or the old-school telecoms businesses, who are having all sorts of fun with their proprietary phone-call-hacking API services. But once you start delving into the numbers, you’re leaving the realm of small data.
Meanwhile, you shouldn’t think of small data as merely a selective distillation of big data. Focusing on individual data points can – with a little human intuition and understanding – yield valuable information that’s overlooked by traditional big-data analysis.
That’s the thesis of Martin Lindstrom’s book Small Data: Tiny Clues that Uncover Huge Trends. Based on extensive consumer analysis, Lindstrom advises businesses not to focus on figures and trends, but to examine the behaviour of individual customers to understand why they act in the way they do.
Get the ITPro. daily newsletter
Receive our latest news, industry updates, featured resources and more. Sign up today to receive our FREE report on AI cyber crime & security - newly updated for 2024.
In particular, it’s suggested that a customer’s decision-making process can be affected by intangible factors such as the climate, the governmental and religious context or cultural tradition. Ultimately, the goal is not only to predict what customers will do, but to identify the underlying drivers that motivate them. This is the sort of analysis that big data alone could never produce: it’s fair to say that few customer relationship management (CRM) systems are set up to record and track this type of data, and interpreting it would be problematic for even the most advanced AI.
Why are businesses turning to small data?
Engaging with big data is a lot more complicated than just pulling up an Excel spreadsheet. A query is likely to involve multiple systems and data sources, and often some sort of fuzzy artificial intelligence (AI) interpretation. It’s too big and woolly for most people’s daily tasks.
Even formulating the right questions for a big data system is a challenge. “How do our last five years of sales figures compare to those of our top three competitors?” is a human-grade question: you’re probably better off spending your time putting the graph together yourself and drawing your own conclusions, rather than trying to figure out how to get a meaningful answer out of the AI.
Although we humans can only process limited amounts of data, we have a natural talent for identifying which items are important, and making actionable decisions on that basis. We can also draw on our wider contextual understanding to interpret information: every big data event starts out as small data, so it certainly doesn’t hurt to have eyes on that end of things.
Understanding the economics of in-cloud data protection
Data protection solutions designed with cost optimisation in mind
There isn’t so much of a backlash against big data, as there is a newfound recognition of its limitations. The point of the “big data” approach is to not get bogged down in details. For example, you don’t need to know exactly which numbers are feeding into the output, or how many petabytes they take up – it’s just big data. That’s great as far as it goes, but you can’t run a business entirely on not knowing things.
Big data is, indeed, as powerful as it ever was. The problem, however, is that anything that qualifies for the term is, by definition, too large and complex for a human to comprehend. It’s great to have machines poring over a huge aggregated mass of data to extrapolate emerging global trends, but staff need to focus on much smaller sets of facts and figures. These might be customer profiles, daily sales totals or server statistics – things we can understand and process for ourselves.
What are the limitations of small data?
There are reasons to be wary of embracing small data too wholeheartedly. When a vital part of your process is actively excluding data, it’s easy to be misled.
There are also risks associated with keeping human-readable records and spreadsheets that aren’t connected to the original data source – it’s all too easy for things to get accidentally
changed in ways that could lead to disconnected and misconceived decisions. Ideally, small data should be an application of your big data assets, and treated with at least as much caution and seriousness as the main repository.