The costs of building generative AI platforms are racking up
Businesses must be alert to how much it actually costs to develop and train, or even just use, AI systems
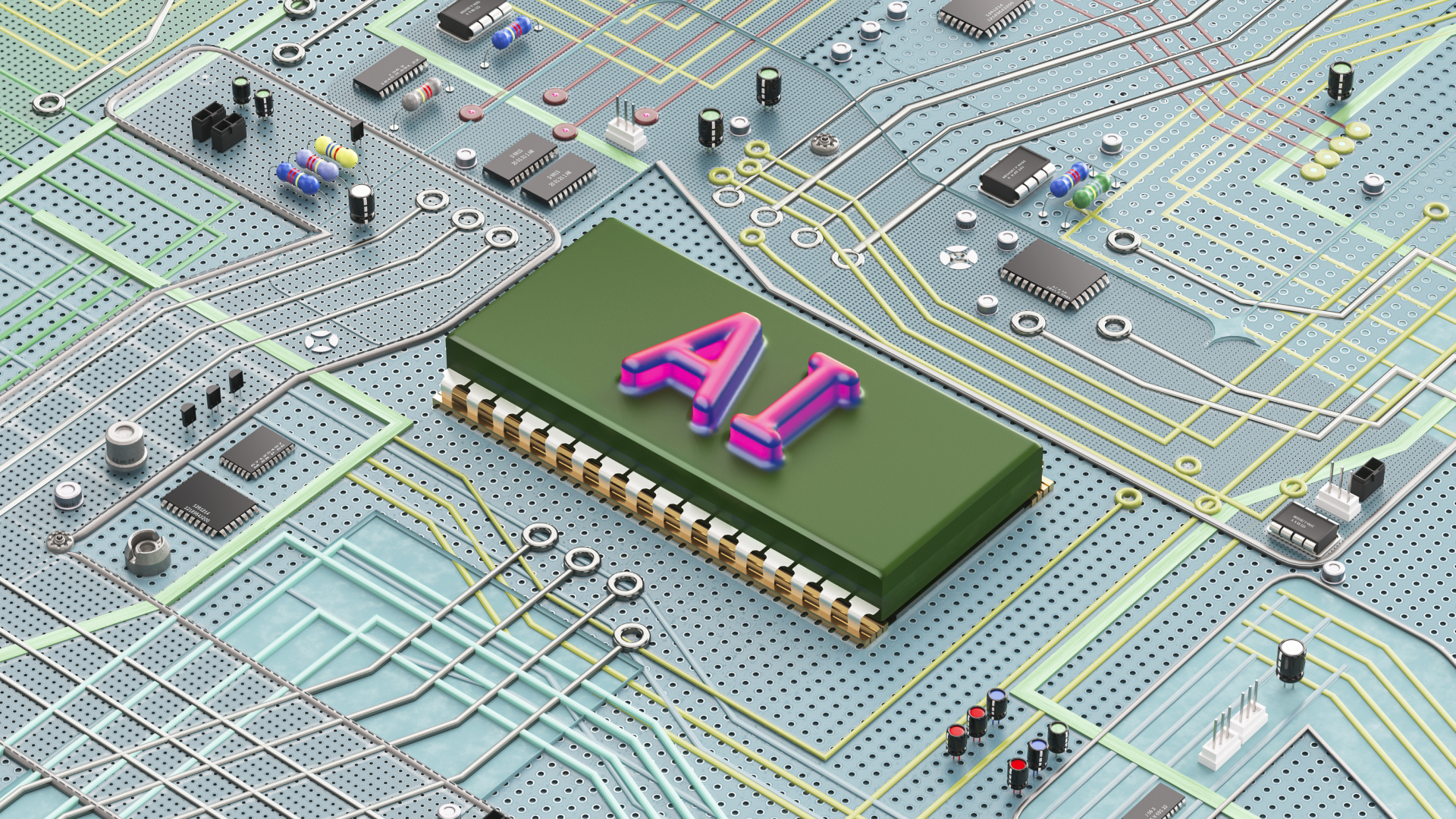
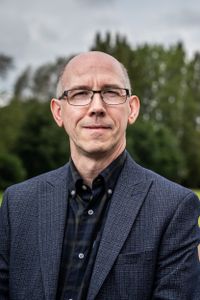
Artificial intelligence (AI) is becoming a critical part of strategic planning as businesses seek to pursue digital transformation projects in the wake of COVID-19. Advancements in generative AI have more than just turned heads, with businesses throwing serious money behind the endeavor.
While the opportunity is ripe, so to is the cost of building and maintaining these tools for an exponentially growing userbase. The cost of GPUs, alone, is huge – with Nvidia A100 chips, at $10,000 apiece, powering many of the biggest AI systems like ChatGPT. With thousands of A100s needed to train some models, the costs can be eye-watering. That’s not to mention the broader compute architecture and the cost of training models in such a way they return sensible results to each query.
Read more
GPUs are just one part of the equation, with systems like Microsoft's Bing chatbot reportedly needing $4 billion in overall infrastructure costs to meet the needs of daily users. Without doubt, enterprises seeking to build their own in-house models aren’t likely to spend anywhere near as much. Even then, it might be cheaper to simply lease a specialized third-party tool as they emerge, but costs can still begin to add up here; it costs as much as $0.15 per query to run ChatGPT, for example, says managing member at Visionary Future, David Shrier.
Continued debate about the risks of unregulated AI expansion notwithstanding, do enterprises understand the cost of using generative AI and how these could impact their bottom line and environmental credentials?
Paying the price of AI intelligence
AI systems undoubtedly offer new opportunities. Some $15 trillion could be added to the global economy by 2030, largely due to much stronger productivity, according to PwC, with IDC predicting global spending on AI will move beyond $300 billion by 2026. Compute architecture providers, too, such as AWS, Azure, Oracle, and Google are more than ready to deliver AI services, too. Azure, for example, hosts ChatGPT and continuously adds capacityto meet surging demand. It’s unclear, however, whether the costs involved can continue to be met.
RELATED RESOURCE
Gain Observability in Cloud-Native Applications explores the significance of observability in the context of cloud-native environments.
DOWNLOAD FOR FREE
"These models tend to scale up; scaling up means to "grow" in dataset size, as well as the increase the compute power that’s needed to train the models,” explains Jan Van Hoecke, head of data science at iManage. “On top of that, the scaling is exponential – every generation of the model becomes larger by ‘x’ factor. This means if you need one compute node for GPT-1, you will need ten compute nodes for GPT-2 and 100 nodes for GPT-3. Similarly, the prediction compute cost goes up too, as this is relative to the model's size.”
OpenAI’s GPT-3 cost $3 million to develop and train, according to an IBM report, and DeepMind’s AlphgaGo cost $35 million just to train. The report added: “With costs this significant (and rising quickly), the conundrum grows – how to balance the need for bigger models, more data and training, as well as more compute power, with the inherent business realities of budgets and efficiency. Researchers will have to address this conundrum, or progress may languish.”
Get the ITPro daily newsletter
Sign up today and you will receive a free copy of our Future Focus 2025 report - the leading guidance on AI, cybersecurity and other IT challenges as per 700+ senior executives
With generative AI in its infancy, how does the initial investment compare against long-term maintenance, training, and infrastructure costs? Stephen J Andriole, Thomas G Labrecque professor of business technology at the Villanova University School of Business, thinks the early costs are more than warranted.
Read more
“As always, there's a bump in development, delivery, and support costs for all new technologies – but longer-term costs decline,” says Adriole. “Remember that investments in AI go back decades to DARPA and lots of other government agencies and universities around the world. Investments are cumulative. Training? It largely disappears as new architectures enable real-time training of even new tasks – all discovered by large language models.”
“Infrastructure? Cloud costs will also decrease over time,” Labrecque continues. “If the argument is the deployment of LLMs will collapse under its own financial weight, then we're all missing the enormous profit providers want to capture.
“They’ll make this profitable for them and productive for their clients, as it's always been, and will always be. How fast did Pfizer and Moderna develop COVID-19 vaccines? Look at the profit they generated. The incentives to build game-changing technology are astronomical. They won't be dismayed by the initial costs.”
The price of scaling up AI
AI represents a global transformation opportunity that we haven’t seen in 200 years, and the pace at which it’s happening is much, much faster than the industrial revolution
David Shrier, managing member at Visiona
Read more
The cost of building AI must be qualified, and final sums can have many components. Businesses hoping to tap into AI are also anxious – justifiably – about fees, as they can quickly spiral. AI systems, after all, aren’t fixed assets but evolving entities with ever-expanding capabilities.
Shrier, however, can see a bright future for the organizations that move quickly to capitalize. “We’ve reached a tipping point where companies simply can no longer afford to ‘wait and see’,” he says, “Given there’s an imperative to do something, the question then becomes, what is the most prudent course to capitalize on growth and manage the downside? AI represents a global transformation opportunity that we haven’t seen in 200 years, and the pace at which it’s happening is much, much faster than the industrial revolution. Smart leaders are aggressively building capacity and preparing for the storm."
The business case for using generative AI across an organization are stil in the process of being defined. Currently, systems like ChatGPT offer an almost consumer-like approach to accessing these services. Developers may become the victims of their own success if the popularity of these services continue to grow exponentially; only businesses with bottomless pockets will manage to keep their AI systems alive and evolving.
The massive amount of computing power and data storage needed may restrict how generative AI develops over the next few years. Potential hidden costs of massive data center expansion to meet these demands, and the impact this could have on enterprises’ environmental credentials can't be ignored either. But there’s a risk, in the midst of the AI gold rush, these concerns will fall by the wayside until it’s impossible not to reckon with them.
David Howell is a freelance writer, journalist, broadcaster and content creator helping enterprises communicate.
Focussing on business and technology, he has a particular interest in how enterprises are using technology to connect with their customers using AI, VR and mobile innovation.
His work over the past 30 years has appeared in the national press and a diverse range of business and technology publications. You can follow David on LinkedIn.
-
Marc Benioff’s agentic AI gambit is paying dividends
Analysis Agentforce is dominating the agenda at Salesforce – and it appears to be working
-
Enterprises are worried about agentic AI security risks – Gartner says the answer is just adding more AI agents
News Not content with deploying agents for frontline operations, some enterprises might double down with ‘guardian agents’ to monitor their bot-based workforces
-
Latest ChatGPT update lets users record meetings and connect to tools like Dropbox and Google Drive
News New ChatGPT business tools aim to unlock corporate information sharing tools from Otter.AI, Zoom, Google and Microsoft
-
DeepSeek and Anthropic have a long way to go to catch ChatGPT: OpenAI's flagship chatbot is still far and away the most popular AI tool in offices globally
News ChatGPT remains the most popular AI tool among office workers globally, research shows, despite a rising number of competitor options available to users.
-
'People use it much more than we expected': Sam Altman says OpenAI is 'losing money' despite launching $200 ChatGPT Pro subscription
News OpenAI CEO Sam Altman admitted the company is still "losing money" despite launching a $200 per month Pro subscription tier for ChatGPT
-
Everything you need to know about ChatGPT Pro, including pricing, features, and available models
News OpenAI has launched a new subscription tier for ChatGPT at a cost of $200 per month.
-
ChatGPT could be facing some serious competition: Amazon is reportedly working on a new AI tool, ‘Metis’, to challenge the chatbot’s dominance
News Amazon could be preparing to mount a serious challenge on ChatGPT’s dominance with the launch of a new chatbot service
-
Big tech’s sleeping giant has finally entered the generative AI arena
Analysis Apple unveiled a raft of new AI-powered tools and features at its WWDC conference this week
-
ChatGPT answers still can’t be trusted as EU regulators cite GDPR non-compliance
News ChatGPT is still inaccurate when it comes to returning responses to users, EU lawmakers have warned
-
Generative AI is here: Are you ready?
whitepaper Best practices for bringing AI to organizational data