IT Pro Panel: Building a data-first culture
How do you make data-driven decision-making part of an organisation’s DNA?
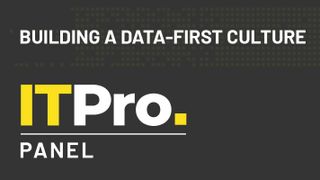
Oil, gold, water, air – whatever you equate data to, there are few organisations that won’t acknowledge data’s importance to business operations. IT leaders have been making efforts for years to place it at the heart of their organisations’ decision-making processes, and these initiatives are now coming to fruition. Most companies now have some form of data analysis capability in place and are on their way to transforming their data into actionable insights.
Now that the groundwork has been laid, however, the next task facing technology and data teams is to embed these strategies and attitudes into the DNA of their organisations. In order to truly derive value from data, it must be embraced throughout it, rather than being the domain of a siloed team producing on-demand reports.
This is a challenge that many members of the IT Pro Panel are grappling with on a daily basis, and in this month’s IT Pro Panel discussion, we’re taking a look at how to instil a truly data-first culture within your organisation.
Share the love
The wider business community has been gradually opening up to the possibilities offered by data, and convincing senior leadership to invest in data operations isn’t the uphill struggle it once was. After a four and a half year stint at Addison Lee, Graeme McDermott became TempCover’s first chief data officer in September 2020 and says that the company’s decision to recruit for the position indicates the growing role that data is playing within the organisation’s strategy.
“Previously, ‘data’ was done in IT and finance. In reality, they were not experts by their own admission,” he says, “so they happily gave me the keys to the data cupboard when I arrived. Before I got there, I think the company was very numerical but not very data-driven. They'd make a decision on a set of numbers, but they’d be from three hours of trading and therefore not statistically significant.”
As an accountancy practice, data is at the core of what Kreston Reeves does, explains IT and operations manager Chris Madden. However, he also notes that there is still work to be done on integrating siloed data sets to provide a more holistic overview of the business.
“We can see the potential to aggregate the data we hold to provide greater insight into our clients’ businesses and the potential for new services that data depth would enable,” he says. “Our data is a mix of standing data (i.e. names and addresses) but also financial information such as accounts, tax, and personal wealth. Given those data sets, we have a lot of data on a client or business – however, it’s how to aggregate and analyse it that’s our challenge.”
Get the ITPro. daily newsletter
Receive our latest news, industry updates, featured resources and more. Sign up today to receive our FREE report on AI cyber crime & security - newly updated for 2024.
“As an example, if we have data from hundreds of similar businesses, we could look at overall trends in profitability to then provide advice ... but we need to know the benchmarks first. And it’s getting all that separate software to integrate and data to be accessible that’s the challenge.”
RoosterMoney also operates in the financial sector, and CTO Jon Smart says data is a similarly key part of its strategy. As a younger startup, data has been built into the company’s infrastructure in a more centralised manner and is used for tasks including trend analysis and marketing.
“We use as much data as we can to understand the reach of product ideas,” says Smart.
Data can be useful outside of a purely commercial context as well, as demonstrated by Guide Dogs for the Blind CIO Gerard McGovern. The charity uses data extensively in its canine breeding programme, including a system which McGovern refers to as ‘dog relationship management’, as well as using it to fuel outreach programmes and donor marketing.
“Without doubt, we could not function without data,” McGovern says, “but we still have a way to go before everyone understands just how important data is to our success. Implicitly, people know and use data, but being explicit about it is the next stage in any data strategy. We’re like teenagers about to head off to university: We’ve got very good building blocks in place, but as a whole, we need to learn (and experience) a lot more.”
“I love that comment Gerard,” adds McDermott; “it’s all about maturing over time at a pace that suits your organisation.”
McGovern notes that while there are certain employees with strong data skills, relying on these individuals to drive an organisation’s data strategy is insufficient, adding that “not enough” departments within the charity are comfortable with interpreting data.
“All departments have people who are data literate, and many of those actively use and manipulate data as a fundamental part of their role, but it needs to be embedded deeper. Those people can’t be the ones people always go to; the knowledge needs to spread and grow.”
This view is echoed by Madden, who argues that Kreston Reeves needs more employees who have data skills first and accountancy skills second, “rather than great accountants who are less in tune with tech”.
“Our industry is ripe for technology and AI to disrupt it, and we need to be ahead of that curve,” he says. “I agree with Gerard in that there are a small number of people who are very good at data manipulation. However, they then become the 'go to' people and knowledge does not propagate further.”
This problem is liable to become more acute the larger a business is, but for those with smaller headcounts, it’s easier to manage the distribution of knowledge. RoosterMoney, for example, has been able to spread data skills throughout almost half its employees.
“We have a core data team with three employees, in addition to a number of developers who are very data-literate,” Smart says. “However, we have been on a drive to extend literacy within the company to allow many non-tech roles to self-service their data. I would say therefore we have around 40% of our overall team that are capable of querying our data for the insights they require. We would love for that number to be at least double that.”
TempCover is also on its way to a 40% rate of data literacy, according to McDermott, and he estimates that a year ago, it was less than 20%. He attributes the increase to education initiatives - including showcasing new data products at monthly company meetings - and a focus on recruiting new hires with pre-existing data skills.
“Sadly, I’m not allowed to interview the sales director” he jokes, “but perhaps I should.”
All hands on deck
Of course, one of the best ways to learn about a topic is simply to get stuck in, and so making data accessible across the business has been an understandable priority for a number of our panellists. The theory is that by giving people greater freedom to access and experiment with data, they’ll not only become more confident in their use of it, but also start to see greater possibilities for its application within their own roles.
“We recently added a piece of software which was developed by one of our vendors and used by a couple of other accountancy firms,” Madden explains. “This gives our people a better picture of a client by bringing together data from different sources such as client databases, time and billing records, document management systems, Companies House, tax software, et cetera.”
“The aim was to provide information on one screen to enable richer client conversations. It also acts as a basic prospect and opportunities tracking tool. However, it’s a starting point rather than an end in itself.”
All of our panellists stressed the importance of combining greater data accessibility with education on how to manipulate and interpret it in order to build a culture of data literacy. McGovern points out that while giving people access to the data they want can be tricky, “the even more complicated challenge is creating access to data that people didn’t even know existed”.
To help support this, Smart’s data team have been running regular ‘lunch and learn’ sessions, taking other staff members through the process of using data to answer various questions. This includes basic information like where to find data and guidance resources, as well as more in-depth skills like how to write queries and use data visualisation tools.
“More recently, we had an all-hands event where everyone in the company split into teams (with at least one competent data person in each) and set out to solve a challenge with our data. This is all geared around getting everyone more interested and then improving their skills. I would say it’s all still quite technical at present, but alongside this, we’re looking for ways to make use of tools to simplify the process and improve team engagement.”
“I was impressed with two-thirds of the company attending the initial session and participation has stayed strong. The feedback from the all-hands event was great and team members are showing more interest. The challenge now will be keeping up with their demand for improvements.”
McDermott also favours this view over what he calls the “dump and run” approach, educating staff about how internal dashboards can be used to answer basic questions. As a result, the problems that get brought to the data team are significantly harder to solve, but much less frequent, giving McDermott’s team more bandwidth to address them.
“I echo Graeme’s view,” Madden adds. “It’s largely an education and training issue. Rather than looking just at data, the bigger issue is perhaps people not really understanding the various pieces of software, and so only scratching the surface of what is possible.”
“We’ve just appointed a dedicated IT trainer and the aim is to provide 1:1 sessions for those struggling more, classroom and remote sessions on key 'how to' messages, and in general, trying to upskill our people to get more from what they already have. The aim is to also reduce the tendency for people to want to buy more software to solve an issue that could be solved with what’s already in place, if only people had the understanding.”
This urge to invest in new software for data management and visualisation is perhaps understandable, given the glut of products currently on the market. Which tools and frameworks are best is often the subject of fierce debate within the data science community, but as McGovern identifies, Microsoft Excel stands head and shoulders above the rest in terms of sheer popularity.
“For the masses, we use Microsoft PowerBI and show them the export button,” McDermott says, “so Excel comes in as Gerard says. Generally though, we've built out a lot of data visualiations, so people don't resort to Excel that often with our data.
“I also analyse the report usage diagnostics to see who is using what and when...and more to the point who isn't. In the latter case, we softly target them to see if there is a problem. Other data tools tend to be the preserve of the data and analytics team, like SQL, or R.”
PowerBI was the starting point for Smart’s management dashboards, but he reports that these are being phased out in favour of Amazon’s QuickSight alternative, as this pairs well with RoosterMoney’s AWS data lake implementation.
“In terms of more hands-on, lower-level access, we have opened up areas of our data using AWS Athena, and have the ability for insights with JupyterLab,” he says. “We have also recently been performing some trials with Apache Superset. We’re finding that rushing to create a dashboard or report for everything was using more effort than required for something that may only be used once or twice, so we’re currently looking at ways to get a balance of what is a low-frequency analysis versus what is something that will be looked at on a regular cadence.”
For McDermott, there’s no definitive answer to the question of which data tools are ‘the best’. Instead, it all comes down to the needs and abilities of the staff that will be using them.
“I've reviewed and used many of them,” he says; “they all have their merits and demerits. It’s often more a case of the right one for you. A few years ago, everyone said Tableau this, Tableau that – and yes, it was fantastic for putting visualisations over some data, but if you needed a bit of data management or integration, then it wasn't for you. Plus, with so many open source visualisations within the tools in the market places, a great visualisation developer can make any of them look amazing.”
Draining the swamp
In order to present data appealingly, you also have to find a way to store it effectively, and there’s two main methods that have come to prominence in recent years: Data warehousing and data lakes. They each have their own advantages, and both are in use amongst our panellists.
“I prefer to use the term ‘data platform’,” says McDermott, “as ‘data warehouse’ conjures images of old, slow, incumbents with long backlogs. The data platform contains my data warehouse on Azure SQL/ADF, user data stores, and standalone datastores that don't link to the data warehouse but provide a safe, secure environment to host data for end users.”
“I fully agree on calling it a data platform,” McGovern adds. “The underlying technologies should be invisible to the users; all they should be concerned about is how they can easily access data, and be able to manipulate and use it.”
Smart, meanwhile, favours the data lake approach, where structured and unstructured data is stored together. This is primarily for the sake of improved performance, agility and responsiveness.
“We then perform transforms to produce different catalogues to assist the tools and make sure that data confirms with our dictionary,” he says. “We were essentially looking to achieve a schema on read rather than working towards everything being aligned with a Warehouse schema.”
“We have many sources of data and they range from structured to unstructured; mutable and immutable. We then also like to make changes to some of those schemas. The main advantage that we strived towards was not to have everything fall apart whenever a schema changed somewhere. On looking at the different approaches, we wanted a solution that was quite dynamic and flexible. The lake approach offered that as an option – providing we could build it in that way.
“Another advantage was the separation of storage and compute, with a race to make storage costs really cheap – having everything stored in simple storage and then running serverless technologies to transform or query the data felt quite fitting to our needs.”
However, regardless of which approach an organisation favours, preventing ‘data sprawl’, where your data assets are too numerous and varied to keep effective track of, should be a key priority for any business.
“We’ve approached it via separation of user type areas,” explains McDermott. “We had areas for insight analysts, MI analysts, database developers, and so on, so we could monitor storage, age of data and usage. We also had controls over how data gets ingested, so we had semi-permanent areas that required a senior sign off and transient areas where anyone could do what they wanted – but it would disappear after 24 hours or a week.
“It stops the users building cottage industries of data stores all over the place, makes it easy for them and the company retains control and knowledge of data. As I've often run everything from data engineering to insight and analytics, I want to make the life of all my data professionals easy.”
“It’s hard work, it takes effort to keep control of it but it’s rewarding if you get it right. And no, it’s not a data swamp or data dump; we keep control of what goes in and what we call it. A good data platform (or whatever you call it) needs good governance to keep control of what is held, why and definitions.”
To illustrate this, he likens a previous company’s so-called data warehouse to “a data cupboard”, where they simply opened the door and tossed data in, with no thought for what data was where.
As McDermott notes, though, a well-managed data strategy can prove extremely rewarding once adoption begins to pick up. Its impact can also be felt throughout the business, rather than just in the traditional departments of finance or IT. For example, McGovern cites fundraising as a particular area that has benefited immensely from Guide Dogs’ use of data.
“They simply couldn’t work without data but by creating that single platform, and giving them the right tools, they are able to drive real insights, opportunities and efficiencies,” he says, “like being able to overlay web browsing data so we can better target our email and postal communications. If we know they have visited the page on Legacy giving, we may promote will writing services.”
Smart, meanwhile, has seen his marketing department make tremendous efficiency gains, reducing processes that would have previously taken weeks of back and forth to just a few minutes. Interestingly, he also reveals that his development team has also shown an interest in using processed data to close the loop on certain queries.
Perhaps less surprising is the fact that some of the most successful users of data within Kreston Reeves have been the audit teams, which Madden says have been using AI-enabled tools to provide a more comprehensive view of client data.
“The barrier to adoption, however, is the data ingestion process, as each client’s data needs individual mapping in year one - so it’s additional work for our people initially, with the bigger benefits in year two.”
“We have not yet found a tool that can really help with the data transfer, as all require an initial mapping exercise. In a set of accounts, there may be many ways to structure the recording of transactions, bank statement entries and so on. Therefore, it’s difficult to write a program to work out what goes where, so there’s still human input needed. If anyone cracked such issues, they would make a fortune!”
McDermott’s biggest wins have been in departments that have traditionally operated with siloed data, where providing a more holistic overview can unlock advantages not just for the business but also for its customers.
“I suppose my own success has been with areas that aren't used to seeing joined-up customer data,” he says. “For example, we worked with our complaints unit to share the single customer view, so they could see how many products a customer had, how much they spent, et cetera – which siloed product systems didn't show.”
“They then changed the refund policy and customer-friendly give backs as a result. We felt we rewarded those who deserved it and had ‘paid in’ and didn't reward those who were perhaps trying it on. It helped us develop product propensity models that helped predict whether a refund or gesture would have any impact or not. It took away the subjectivity, and made it more objective and data-based.”
The common thread amongst all these examples is they involve opening the wider organisation’s eyes to the possibilities of what can be done with data. While tools, frameworks and architectures may be an attractive avenue for those of us seeking to make our businesses truly data-led, our panellists agree that all the software in the world isn’t going to succeed in this goal without a strong programme of education and enablement.
Transforming an organisation into a data-driven one is a worthy ambition, but it’s not an easy one. It requires sustained effort, and – like all things in IT – eventually, it comes down to people.
Adam Shepherd has been a technology journalist since 2015, covering everything from cloud storage and security, to smartphones and servers. Over the course of his career, he’s seen the spread of 5G, the growing ubiquity of wireless devices, and the start of the connected revolution. He’s also been to more trade shows and technology conferences than he cares to count.
Adam is an avid follower of the latest hardware innovations, and he is never happier than when tinkering with complex network configurations, or exploring a new Linux distro. He was also previously a co-host on the ITPro Podcast, where he was often found ranting about his love of strange gadgets, his disdain for Windows Mobile, and everything in between.
You can find Adam tweeting about enterprise technology (or more often bad jokes) @AdamShepherUK.