Structured vs unstructured data management
Big data is big business – if you have the skills to manage it
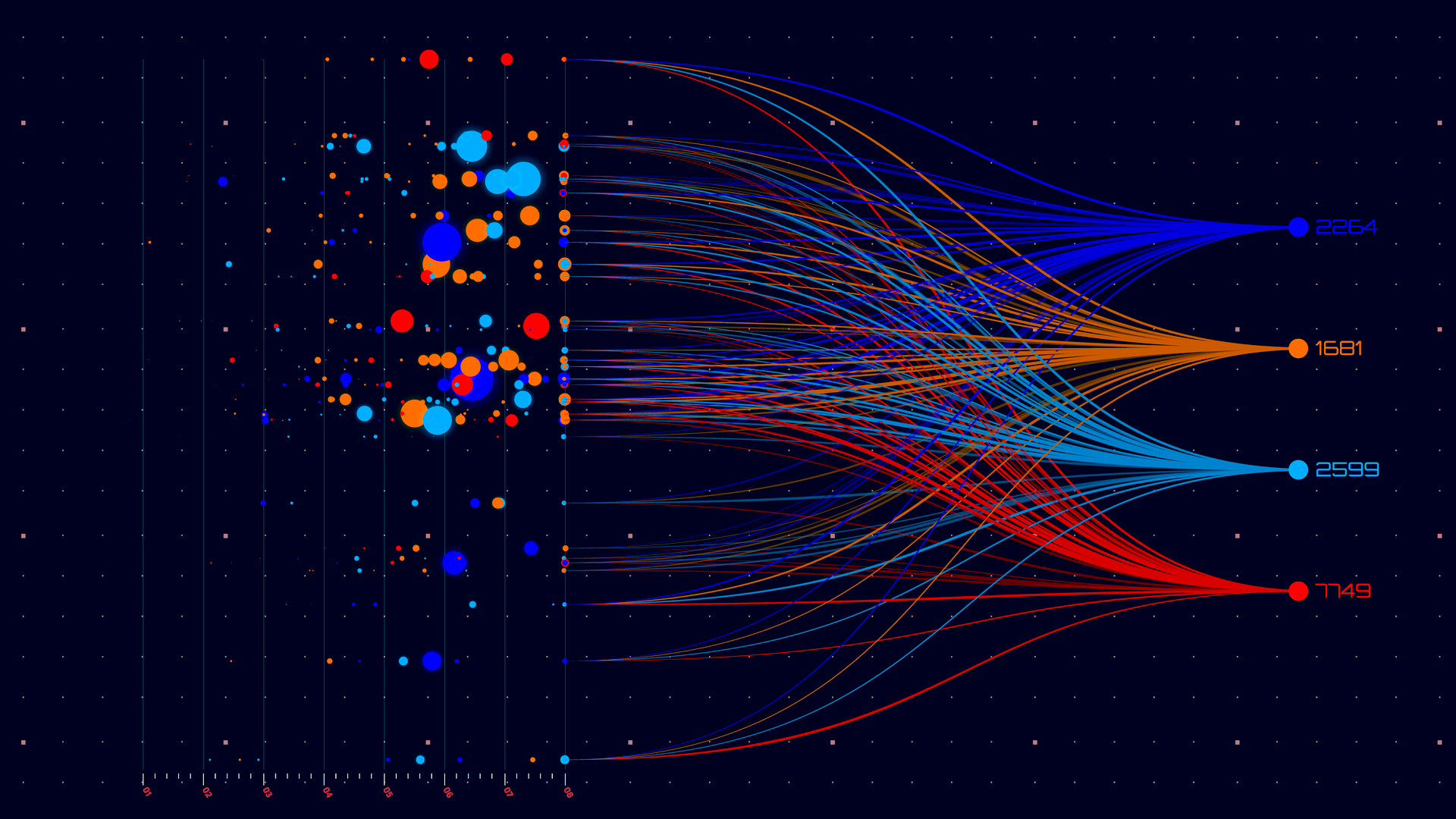
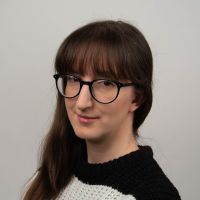
Businesses at the top of their respective industries all have one thing in common: the knowledge that data, both structured and unstructured, forms the heart of their organization and is key to their success.
A comprehensive and nuanced data strategy is therefore vital.
Creating one, however, is not an easy feat. It isn’t just a matter of dealing with all the sources of data; it’s also having expertise in the tools required to manipulate and process that data and understand what needs to be structured and where a rigid model is inappropriate.
Do you have people that are experts in Excel, SQL, NoSQL, PostgreSQL and Oracle? Does your business have a suitable data lake so all corners of the company can benefit from the data itself and generate their own specific insights?
If you answered ‘no’ to any of these, then your data management strategy might not be up to scratch. How you feed that data into your business, or data lake, is up to you. But understanding how to store that data - either in a structured or unstructured manner - is crucial in making that next big step in your business’ journey to the top.
What is structured data and how is it managed?
When it comes to databases, structured data is often what first comes to mind.
Structured data - also known as relational data - is stored in tables with columns and rows. The structure is described by a schema, with relationships defined between the tables. For example, one table might contain a list of customers, another table might contain a list of telephone numbers with one row in the customer table potentially having many connected rows in the telephone numbers table.
RELATED RESOURCE
An EDR buyer's guide
Learn how to select the best endpoint detection and response (EDR) solution for your business.
Structured data is, by its very nature, already “managed”. The definition of the tables and columns means that data must be kept in an orderly fashion. Constraints can be added to the columns to, for example, ensure that only numbers are entered for telephone numbers and that no telephone number can be added without an associated customer.
The structured approach to data storage has both benefits and drawbacks. Users are able to understand and access data easily, with a wide variety of tools for querying and analysis available. However, it can also be regarded as highly inflexible when the data needing to be stored does not fit the structured data model.
It's also worth noting that a relational database management system (RDBMS) is often embedded in products that also offer far more bells and whistles than just managing data and making it available to queries.
For example, Salesforce, the cloud-based customer relationship management (CRM) platform, manages the structured data put into it, but also offers tools like chat, access to the Force.com development platform, analytics, and so on.
What is unstructured data and how is it managed?
Unstructured data is anything that can't be organised into a structured database. Common examples are free-flowing text-based interactions, such as email conversations or chat logs, word processing documents, slideshow presentations, image libraries, or videos.
Estimates vary for how much unstructured data lies in business. One recent projection estimated that 80% of global data would be unstructured in the near future.
It contains a wealth of corporate information but, by its nature, was difficult to access until modern big data analytics and AI have become a reality.
Together with structured data, it's also one of the three Vs of Big Data variety (the other two being velocity and volume).
There are many benefits to using unstructured data. It is rapid to accumulate and there is no need for time-consuming parsing since there is no predefined structure. It can also be stored in its native format and lends itself well to corporate data lakes.
However, the benefits can also be pitfalls; specialized skills and tools are required to analyze unstructured data and users can be left alienated when faced with data that does not adhere to a uniform format.
Common tools for dealing with unstructured data (also referred to as NoSQL) include MongoDB and DynamoDB.
Unstructured data management (UDM) is essential for successfully making use of all this data. Rather than there being a handful of tools to point to for UDM, there are instead some basic tenets to be followed, which we outline below.
Indexing
This term, sometimes known as "discovering" as well as other related terms, means compiling your data to really see what's there, how frequently it is accessed, for how long it has existed, and more.
The objective of indexing is to find out whether this information will potentially bring future value to the organisation and see if it is worth putting in an UDM system and archiving it.
This, however, can be a long process, and can take many weeks to sift and scan all the data. Be ready to dedicate a lot of effort and time to this process in the initial stage. This is also the section where you should add metatags so that the data is easy to search later on in the process.
Storage and availability
Now that the data has been organised, it now requires storing in a suitable location with the correct attributes that make it automatically and easily accessible.
There are a number of storage locations to choose from, which includes general cloud storage like Microsoft Azure or AWS S3 or on-premises data lakes. When the information resides here, it is able to be stored in its "natural" state, which means there is no need to store it in a database format, but also allows it to be available for automated querying through APIs.
When thinking about which type of storage to utilise, it's worth considering how frequently the data that is being stored is accessed. For example, if it's relatively frequent, it might need to be put in "cold" storage, which is usually much cheaper than if it is kept in storage that makes the information accessible at all times. However, in this "cold" storage it will be slower to access initially when you do need to sift through it and query it.
Semi-structured data
Semi-structured data can be regarded as a “halfway house” between structured and unstructured data. While it does not have the predefined model of structured data, it is easier to store and work with than unstructured data.
Examples of semi-structured include JSON or XML. Although the columns and definitions of traditional structured data are not usually present, the data does contain tags and markers to separate elements, making for simpler querying. These tags and markers are referred to as “metadata” and mean data can be better catalogued.
Vector databases
Also found in the category of unstructured data - although increasingly meriting a section of its own - and becoming progressively more popular in the era of generative AI, are vector databases.
As with unstructured data, pretty much anything can be dropped into the database. However, an embedding function encodes the data into vectors to capture its meaning and context. The result is something that can be searched based on similarity or vector distance.
An example would be searching for an image based on content or style. Or a large language model (LLM) generating more relevant and coherent text.
Examples of vector databases include Pinecone and Weaviate.
Get the ITPro daily newsletter
Sign up today and you will receive a free copy of our Future Focus 2025 report - the leading guidance on AI, cybersecurity and other IT challenges as per 700+ senior executives
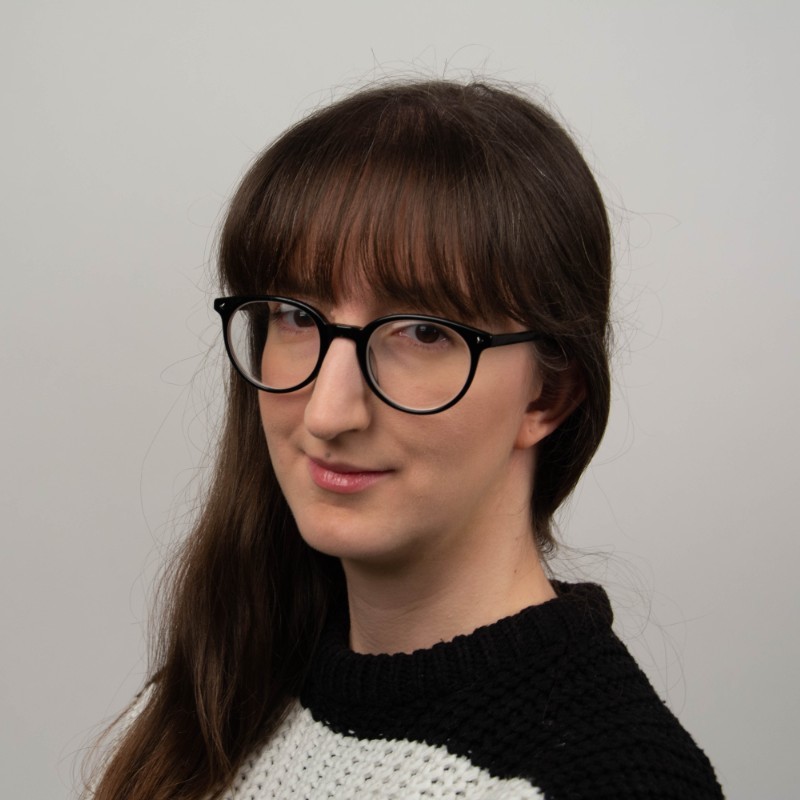
Jane McCallion is Managing Editor of ITPro and ChannelPro, specializing in data centers, enterprise IT infrastructure, and cybersecurity. Before becoming Managing Editor, she held the role of Deputy Editor and, prior to that, Features Editor, managing a pool of freelance and internal writers, while continuing to specialize in enterprise IT infrastructure, and business strategy.
Prior to joining ITPro, Jane was a freelance business journalist writing as both Jane McCallion and Jane Bordenave for titles such as European CEO, World Finance, and Business Excellence Magazine.
-
RSAC Conference 2025: The front line of cyber innovation
ITPro Podcast Ransomware, quantum computing, and an unsurprising focus on AI were highlights of this year's event
-
Anthropic CEO Dario Amodei thinks we're burying our heads in the sand on AI job losses
News With AI set to hit entry-level jobs especially, some industry execs say clear warning signs are being ignored
-
Empowering enterprises with AI: Entering the era of choice
whitepaper How High Performance Computing (HPC) is making great ideas greater, bringing out their boundless potential, and driving innovation forward
-
Accelerating revenue with IBM
whitepaper Increase your impact and grow new revenue streams
-
The CEO's guide to generative AI: Be a creator, not a consumer
Whitepaper Innovate your business model with modern IT architecture, and the principles of trustworthy AI
-
Learning and operating Presto
whitepaper Meet your team’s warehouse and lakehouse infrastructure needs
-
Scale AI workloads: An open data lakehouse approach
whitepaper Combine the advantages of data warehouses and data lakes within a new managed cloud service
-
Managing data for AI and analytics at scale with an Open Data Lakehouse approach
whitepaper Discover a fit-for-purpose data store to scale AI workloads
-
The power of AI & automation: Productivity and agility
whitepaper To perform at its peak, automation requires incessant data from across the organization and partner ecosystem
-
A guide to help you choose the UPS battery backup for your needs
Whitepaper Download this guide and stay connected with a UPS that's free of interruption or disturbance