Seven reasons why machine learning is a good career
Seen as one of the fastest growing areas in technology, there are several key reasons why machine learning is a field worthy of carving out a career in
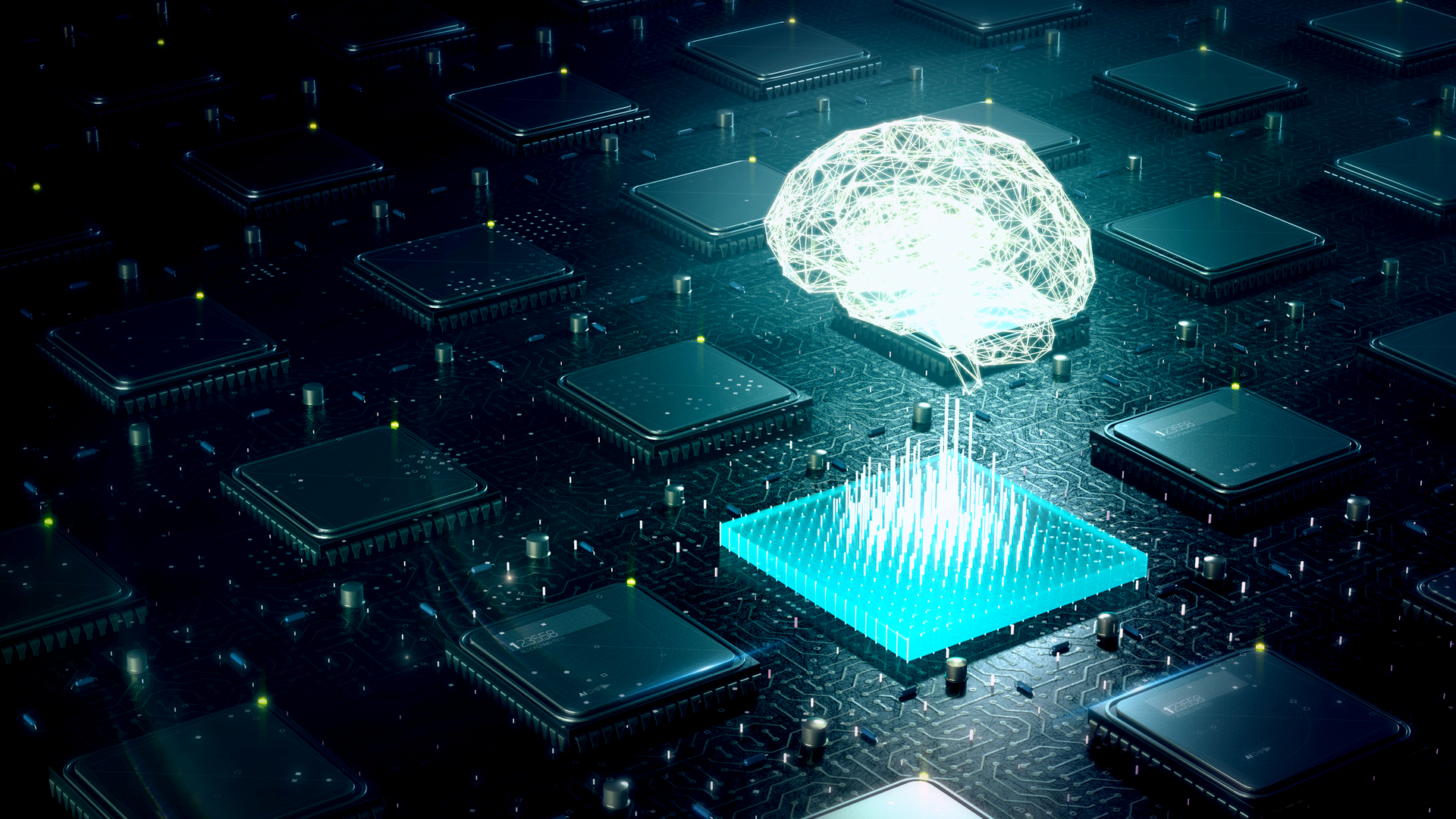
If you’re looking to enter the technology industry or pivot to a new career within it, then machine learning should be high on your list of skills to learn.
RELATED RESOURCE
MLOps and trustworthy AI for data leaders
A data fabric approach to MLOps and trustworthy AI
Demand for machine learning professionals is soaring with more and more enterprises, businesses and organisations looking to embed and scale it, as they move away from legacy systems and work through on-going digital transformation programmes.
Compared to five years ago, experts suggest there are now more realistic expectations for what machine learning can deliver. This means the field is increasingly focused on solving specific problems, with new systems making it easier for people to understand.
There are several routes into a machine learning career, from university degrees to short-term machine learning courses. The remuneration is good too, with Indeed suggesting the average annual salary for a machine learning engineer is around £56,000. If you’re considering taking this path, there are several reasons why it could be a good career move.
Asking questions and finding wrong answers
If you’re inquisitive, then machine learning is a great career for the intellectually open and curious, says Janani Ravi, Pluralsight author and one of the original Google Docs engineers.
“The process of building a machine learning model that works is inherently about going deep into the use case,” Ravi says. “Correctly framing the problem is half the battle, asking the right questions and not only focusing on finding the right answer.
“Machine learning professionals are increasingly expected to pull their weight in all aspects of the technical development process. It’s no longer acceptable to specialise too narrowly or be standoffish about participating in the process of data cleaning, labelling and doing all the detail work that is so critical in making machine learning work well.”
Get the ITPro daily newsletter
Sign up today and you will receive a free copy of our Future Focus 2025 report - the leading guidance on AI, cybersecurity and other IT challenges as per 700+ senior executives
A wealth of different opportunities
There are many roads into machine learning, says Paul Clough, professor of search and analytics at the University of Sheffield (UoS) and head of artificial intelligence (AI) and data science at Peak Indicators. “This could be as part of any job role that may utilise more advanced analytics or modelling methods for making the most of the data available in that role,” he explains.
“Additionally, machine learning is a key tool in the toolbox of any data scientist or analyst, especially if the job involves predictive modelling.”
Clough adds machine learning may also feature in the job specs for data engineers as well as more specific roles such as machine learning engineer, machine learning (research) scientist, MLOps engineer and algorithm machine learning engineer. “We’ve seen a rise in new roles like data translators and machine learning analysts, both related to explaining the benefits of data science projects to business leaders,” he adds.
Choosing between academia and industry
There are two distinct pathways to choose from, Andrew Patel, senior researcher at WithSecure Intelligence, tells IT Pro. “From the careers side, the most obvious thing to note from reading discussions between machine learning experts is there’s a clear divide between career paths. One side is in academia and the other is in industry.
“I get the feeling the academic side is seen as more interesting – you get to work on more experimental projects and likely use more cutting-edge techniques – whereas on the industry side, you’re forced into solving very defined problems and productising machine learning solutions.
“From the salary point of view, the industry pays better. I believe there is high demand for machine learning experts in all fields currently.”
IT’s a rapidly maturing jobs market
RELATED RESOURCE
Why smart businesses view a data fabric as an inevitable approach to becoming data driven
Adopting a data-driven strategy for success
Machine learning has evolved significantly as a career over the past decade, says Heather Dawe, UK head of data at digital transformation company UST. This is because its models are no longer developed and used in isolation.
“The machine learning industry is burgeoning in the sense that a significant proportion of enterprises are still grappling with the work required to get their data ready for machine learning. As enterprises mature, the demand for ML talent within the industry will increase even more and at a pace,” she explains.
“There is currently more demand than supply. This demand will increase as businesses mature with their data and wish to leverage it further. Machine learning is a fascinating area to work in. Ultimately, it’s a way of predicting the future and affects decision-making accordingly. Machine learning underpins AI, which is increasingly a part of our futures.”
Machine learning is accessible to the masses
New technology is making a career in machine learning far easier to consider, suggests Eleanor Watson, IEEE AI ethics engineer and AI faculty at Singularity University. Large statistical models can solve thousands of different problems just with a simple natural language request.
She explains: “With this new technology, machine learning is finally accessible to the masses, as we no longer require much skill beyond asking a simple question to obtain quick and reasonable assistance with almost any digital office task one can imagine.
“The latest models are even generating computer code, video, 3D models, virtual personalities and music on-demand from nothing more than a description of the desired output. One might think machine learning skills will be less needed as a result. However, by making the power of state-of-the-art machine learning clear to the public, the desire for improved capabilities to optimise almost any problem will be greatly increased.”
More routes into machine learning are opening up
A few years ago, the path to entering the data science field was academic and highly technical, according to Elliott Young, CTO Dell Technologies UK. Now, a lack of a degree in applied maths is no longer a barrier, he suggests.
“These days, the outlook is very different, with tools available to make data preparation and the creation of machine learning models more straightforward and automated to get results faster,” he says. “The existence of these tools helps to expand the nature of roles within the field of data science.
“A career in machine learning has become more about the value you can extract from data than writing programmes in R or building models from scratch,” he continues. “Machine learning can be a fascinating area in which to work. There is more opportunity for people to create truly novel models and approaches that didn’t exist before and make an important difference to the future.”
Self-training isn’t frowned upon
For those who were unable to take the classical route of a university education, a career in machine learning can still be within reach. Dr Huseyin Atakan Varol, department chair of robotics at Nazarbayev University SEDS and founding director of the Institute of Smart Systems and Artificial Intelligence (ISSAI) says: “There is also a growing number of self-trained machine learning specialists without formal education on this topic.”
Dr Varol suggests rather than big data and supercomputing, companies are now looking for workers who can create Tiny AI, machine learning models that can run on small microcomputers on Internet of Things (IoT) devices or wearables.
“It’s a great area to work for individuals who are keen on self-development and learning,” he adds. “Machine learning might be the fastest-evolving field in the world. Therefore, without learning new things, a person will become obsolete very quickly. It has high starting salaries compared to other technical fields.”
Jonathan Weinberg is a freelance journalist and writer who specialises in technology and business, with a particular interest in the social and economic impact on the future of work and wider society. His passion is for telling stories that show how technology and digital improves our lives for the better, while keeping one eye on the emerging security and privacy dangers. A former national newspaper technology, gadgets and gaming editor for a decade, Jonathan has been bylined in national, consumer and trade publications across print and online, in the UK and the US.
-
Women show more team spirit when it comes to cybersecurity, yet they're still missing out on opportunities
News While they're more likely to believe that responsibility should be shared, women are less likely to get the necessary training
-
Employees want purpose, and they’re willing to quit to find it – upskilling, career growth, and work-life balance have shifted priorities for workers
News Employees want purpose and meaning at work — and if they don't get it, two thirds would quit to find it.
-
DEI rollbacks could exacerbate tech talent shortages – nearly half of recruitment leaders worry diversity cuts will impact their company’s appeal and employee retention
News Finding talent with AI skills has already become a major challenge for enterprises, but with some enterprises shelving DEI hiring practices, research suggests the situation could get worse.
-
Tech firms eye temps to plug talent gaps
News The tech industry could be set for a spike in temporary hiring, according to a new study from recruitment firm Robert Walters.
-
IDC InfoBrief: Sustainability doesn’t need to be all stick and no carrot
whitepaper CIOs are facing two conflicting strategic imperatives
-
Five ways to drive innovation at the edge
Whitepaper How an effective edge strategy can generate new value for your organization
-
Google launches AI training scheme to “supercharge” SMB productivity
News The ‘easy-to-follow’ course aims to bolster SMB leaders’ understanding of machine learning and AI
-
What is quiet hiring?
In-depth Far from being the latest industry buzzword, quiet hiring could be the perfect antidote to the widening skills shortage