Getting your data centers AI-ready
With the explosion of interest in generative AI and LLMs, how can businesses adapt their existing IT infrastructure?
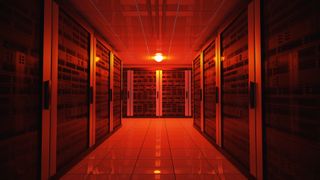
With many IT professionals finding a greater demand for AI services in enterprises, mid-sized, and even some small businesses. One key challenge is accessing hardware that can handle the compute load of AI and installing servers with AI-optimal processing power.
This challenge comes at a time when many face internal pressure to buy into the hype of generative AI sensations ChatGPT and Bard dominate the headlines. Research firms believe this isn’t merely hype but a sign of things to come as enterprises adopt AI for a broad selection of use cases including customer service chatbots and cyber security data analytics.
The costs of building generative AI platforms are racking up
What’s the cost of such rapid adoption? One firm, the Tirias Group, estimates generative AI infrastructure and OpEx costs to exceed $76 billion by 2028. Dell’Oro Group, on the other hand, predicts CapEx will be half a trillion dollars by 2027, driven by AI investments.
This leaves businesses with a dilemma: upgrade or optimize existing hardware to run AI workloads. There is, also, a third way, which may provide a stopgap between those two options.
AI means shorter hardware refresh cycles
Certain AI applications or workloads require new hardware. Brute-forcing AI applications (with high compute requirements) onto existing hardware doesn’t work. That’s according to Dell’Oro Group senior research director Lucas Beran.
AI requires, as we know, machine learning to operate. The two types of machine learning: training and inference run best on different types of hardware. But you really can’t have one without the other.
Inference workloads feed from training applications. Those training applications are compute-heavy since they consume massive amounts of data from your organization to provide a chatbot output that’s accurate in service to your customers. For instance, you can run a training application to consume all of your customer support knowledge base (training) and then use that training application to present chatbot responses to customers about your products or services (inference).
Cloud Pro Newsletter
Stay up to date with the latest news and analysis from the world of cloud computing with our twice-weekly newsletter
Cyber-resilient infrastructure starts with server security
Transform revenue operations through data-driven decision-making.
DOWNLOAD NOW
“For AI training applications, typically completely new systems with GPU accelerators would be needed,” adds Dell’Oro Group’s senior research director Baron Fung. “For AI inference applications, it’s possible to use existing general-purpose servers.”
The challenge is, without training, inference isn’t customized to a business and the entire point of AI collapses.
Chief data consultant of Datalligence, Tatsiana Sokalava, reveals the three-to-five-year server refresh cycle is no longer sufficient. The reason? Enterprises are filling their data centers with more specialized servers into their racks. According to Sokalava, AI-specific hardware includes:
- Graphics Processing Units (GPUs) – known for graphic computations but now critical for large language model (LLM) training workloads.
- Tensor Processing Units (TPUs) – extend deep learning processing power because they’re purpose-designed for LLM training efficiency.
- Field Programmable Gate Arrays (FPGAs) – integrated circuits that can be configured to meet specific user needs.
- Application Specific Integrated Circuits (ASICs) – integrate circuits customized for specific applications.
“These might have different lifespans and maintenance needs compared to traditional CPUs,” Sokalava says. “AI-optimized hardware can be more power-hungry and generate more heat.”
To manage that extra heat and power, data centers need to make changes in physical infrastructure. This isn’t cheap, which heavily influences the cost model of AI-specific hardware.
Should you retrofit existing IT infrastructure to handle AI?
Some firms turn to retrofitting existing servers to help in their firm’s first significant steps in running custom AI applications. If we take a customer service chatbot, for example, we’ll see training the chatbot to know which responses to give based on customer queries could require high compute power.
Chief analyst at SemiAnalysis, Dylan Patel, gives a few examples. ChatGPT runs a large LLM with 1.76 trillion parameters. This requires large clusters of connected AI server. Meta’s Llama 2 LLM, meanwhile, has configurations with 7 to 70 billion parameters. Patel says Meta could conceivably run the AI model through consumer GPUs but there’s not enough capacity to scale. Finally, Stable Diffusion requires 860 million and 123 million parameters for its U-Net and text encoder offerings respectively. This is where consumer-grade hardware has more of a chance
Each parameter is a data point, a discussion, a question, or a variation on a combination of all three. The need for AI-specific hardware designed to manage the training of large amounts of data is in direct relation to the size of the LLM, Patel shares, making hardware retrofitting a viable choice for AI models in enterprises.
Is it worth optimizing servers for AI?
Running AI applications on consumer-grade hardware can be done, Patel says, but should it? We’re clear on the traditional total cost of ownership challenges. But introducing compute-heavy workloads into existing hardware clusters presents several unique risks and considerations for enterprises such as:
- Will retrofitting existing equipment to run AI models void the maintenance and support warranties?
- At what age is hardware viable for AI model usage?
- If we need to scale a successful AI retrofit on existing hardware, how quickly can this be done and at what cost?
- Could leveraging older hardware for AI workloads expose us to security breaches?
- Can we meet our ESG commitments if we experience increased power demands from retrofitted hardware?
Sokalava and Fung both recommend outcome-based decision-making when considering upgrading versus retrofitting for AI. Sokalava advocates the use of benchmarking data to validate performance expectations such as model training time periods and inference speeds.
“A lot will also depend on where your organization is heading and what AI workloads are expected in the next few years,” Sokalava reveals. “If there's an expected surge in demand, it might be more cost-effective to invest in new hardware now.”
Fung adds: “It’s critical for companies to deeply understand their AI workload usage requirements.”
One way to estimate AI workload usage requirements is to turn to the public cloud. According to Fung’s research, firms may find it more economically feasible to leverage the economies of scale at Amazon Web Services (AWS), Google Cloud Platform (GCP), and Microsoft Azure, among others.
“I think going to public cloud for early adoption makes sense as companies can gauge their AI usage," says Fung. "Once they have a better sense of their usage requirements, they can leverage colocation or private clouds, and purchase the right mix of equipment to match their needs for long term use.”
Fung adds CapEx investment isn’t an inexpensive endeavor. To make the right call when seeking investments, it makes sense to understand the lifecycle of AI-specific equipment. Public cloud is a great way to do that.
Lisa D Sparks is an experienced editor and marketing professional with a background in journalism, content marketing, strategic development, project management, and process automation. She writes about semiconductors, data centers, and digital infrastructure for tech publications and is also the founder and editor of Digital Infrastructure News and Trends (DINT) a weekday newsletter at the intersection of tech, race, and gender.